Key Insights
-
AI agents process data in real time for faster, smarter decision-making.
-
Eliminates data silos, delays, and errors with continuous learning and automation.
-
Enhances operations, customer experiences, and competitiveness across sectors.
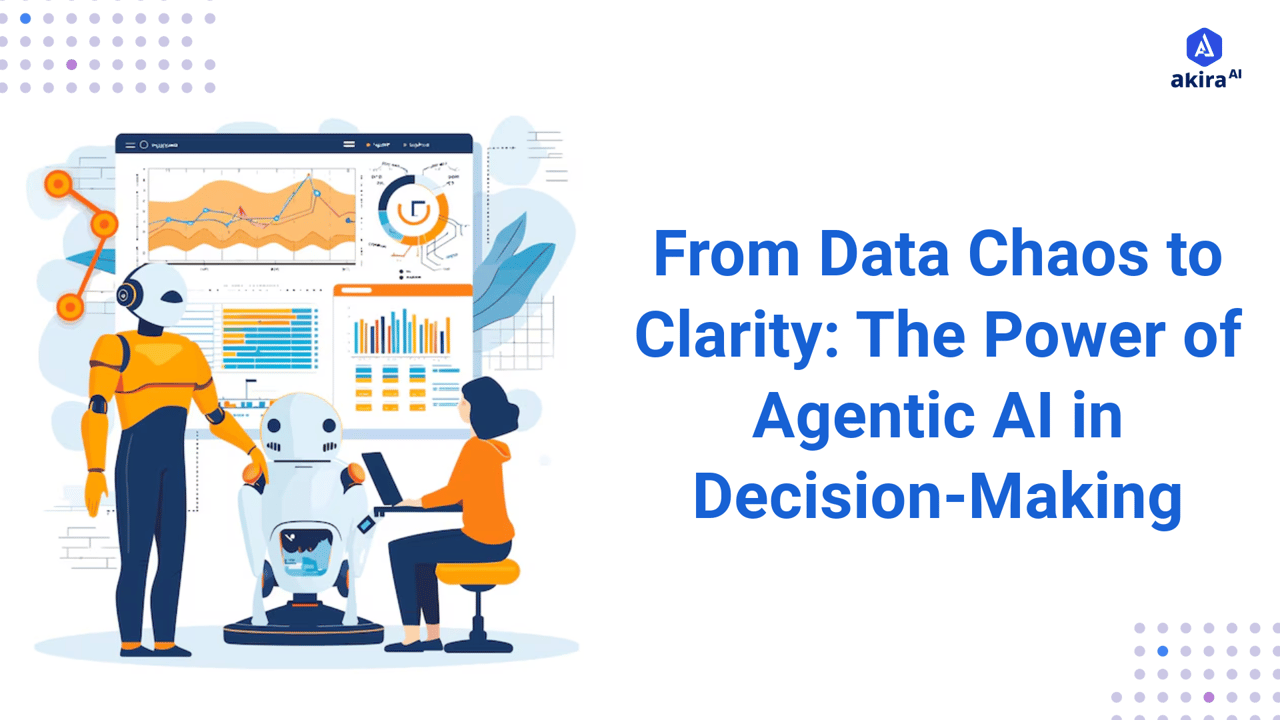
For years, companies have struggled with slow, fragmented, and error-prone data analysis, leading to missed opportunities and costly mistakes. AI Agents is changing the game. Unlike traditional systems, it thinks, learns, and acts autonomously, transforming raw data into real-time, actionable intelligence. Whether predicting customer behavior, optimizing supply chains, or automating workflows, AI-driven decision-making ensures businesses stay agile, competitive, and future-ready.
In this blog, we’ll explore how Agentic AI is revolutionizing decision intelligence, the challenges it overcomes, and real-world success stories that prove its impact.
What is Data-Driven Decision Support?
Decision support in data analysis and management refers to the use of data-driven tools, analytics, and AI technologies to assist organizations in making informed decisions. It involves collecting, processing, analyzing, and visualizing data to identify trends, optimize operations, and enhance strategic planning. By integrating AI-driven predictive analytics, dashboards, and automation, businesses can reduce errors, improve efficiency, and make real-time decisions.
Example: In e-commerce, AI-powered decision support systems analyze customer behavior and predict demand, helping retailers optimize inventory levels and avoid stock shortages or overstocking.
Key Concepts of Data-Driven Decision Support
Data Collection & Integration – Aggregating data from multiple sources to ensure accurate and real-time insights.Data Processing & Cleaning – Ensuring data quality by removing errors, inconsistencies, and duplicates.
Predictive & Prescriptive Analytics – Using AI and machine learning to forecast trends and recommend optimal actions.
Decision Automation – Leveraging AI-driven systems to automate and optimize decision-making with minimal human intervention.
Visualization & Reporting – Presenting insights through interactive dashboards and reports for better interpretation and strategy formulation.
Traditional Way of Data Analysis and Management
Organizations using conventional methods prior to agentic AI implementation typically demonstrated slow speed and poor integration and error rates.
-
Data Silos: The storage of data across different unrelated systems resulted in manual processes that produced latency together with data inconsistencies.
-
Time-Intensive Workflows: Analysts needed to dedicate prolonged periods of time toward data preparation and cleaning steps thus causing delays in decision-making processes.
-
Limited Automation: Traditional systems relied on static, rule-based approaches that could not adapt to rapidly changing data or unforeseen circumstances.
-
Batch Processing: Insights generated through batch processing happened exclusively on pre-scheduled times (daily, weekly, or monthly) so they were always delayed compared to present events.
-
Subjective Analysis: Human-based analyses during this method created fundamental mistakes because the processes relied on individual assessments conducted manually.
-
Delayed Actions: Actionable insights from reports became less effective for market response because the time needed for their generation rendered them outdated.
Impact on Customers Due to Traditional Data Analysis and Management
Traditional data management inadequacies lead to both operational problems and degrade customer satisfaction during their interactions with companies.
-
Slow Decision-Making: Customer dissatisfaction builds when companies need extended time to analyze data and make responses to customer demands.
-
Inefficient Support: Customer service provides substandard assistance when teams use outdated reports since these reports result in poor service quality and mistakes in support.
-
Flawed Strategies: Business decisions with faulty information about data quality often produce misdirected service solutions including price errors combined with unsuitable product guidance.
-
Operational Inefficiencies: The execution mistakes in manual procedures create higher operating expenses that service customers through price hikes or degraded product quality.
-
Customer Dissatisfaction: Inaccurate delivery and persistent delays drive customer trust down and lower their loyalty to the business.
Akira AI: Multi-Agent in Action
A comprehensive solution based on agentic AI transforms the entire data processing lifecycle into an autonomous, integrated workflow. Here’s how a multi-agent system analyzes data and seamlessly integrates with existing systems:
Fig 1: Architecture Diagram of AI Agents in Decision Support
-
Data Ingestion and Preprocessing Agents: Specialized ingestion agents connect to diverse data sources—ranging from cloud-based data lakes and traditional databases to real-time sensor networks—and aggregate the data. These agents perform crucial preprocessing tasks: cleaning, normalization, and enrichment.
-
Analytical Processing Agents: Once data is prepared, dedicated analysis agents deploy advanced machine learning algorithms and predictive analytics models to extract insights. These agents can operate at multiple levels:
-
Predictive Analytics: Forecast future trends, such as predicting equipment failures for predictive maintenance or identifying potential fraud in financial transactions.
-
Prescriptive Analytics: Suggest actionable strategies based on simulations and “what-if” analyses. The agents continuously learn from incoming data, leveraging continuous learning mechanisms to fine-tune their models. Advanced NLP capabilities allow them to translate complex numerical outputs into human-understandable insights, making the results actionable for decision-makers.
-
Visualization and Reporting Agents: Visualization agents transform raw analytical outputs into interactive dashboards and reports. By integrating with existing business intelligence tools and enterprise software (such as CRM and ERP systems), they ensure that insights are communicated effectively across the organization.
-
Interoperability and Integration: Crucially, the entire workflow is built with interoperability in mind. Custom APIs, middleware solutions, and connectors allow each agent to plug into legacy systems and modern platforms alike.
Prominent Technologies in the Space of Data Analysis and Decision Support
Modern data-driven decision support systems leverage a range of advanced technologies:
-
Big Data Platforms: Tools like AWS Kinesis and Snowflake enable the rapid ingestion, storage, and processing of massive datasets. These platforms ensure that even high-velocity data streams can be analyzed in real time.
-
Machine Learning and Deep Learning: The combination of Machine Learning and Deep Learning algorithms uses powerful algorithms to process past and current data in order to detect patterns and forecast outcomes. Front-running techniques in model development include neural networks and reinforcement learning methods.
-
Natural Language Processing (NLP): NLP offers systems the ability to decode and understand natural human spoken or written communication. The system enables users who lack technical skills to conduct data queries in regular English language and receive purposeful results.
-
Multi-Agent Frameworks: LangChain with AutoGen serves as a platform which enables organizations to run several customized agents simultaneously for tackling extensive and multi-stage operational tasks. The frameworks enable effective and scalable performance of the data workflow.
Make Smarter, Faster Decisions with AgentInstruct
How AI Agents Supersede Other Technologies in Data Analysis
Agentic AI transforms data analysis by addressing the inherent limitations of traditional systems. Agentic AI systems differ fundamentally from conventional analytics tools through several advanced features:
-
Real-Time, Autonomous Decision-Making: Unlike static batch-processing or rule-based systems, AI agents operate continuously, processing streaming data and making instant decisions. Using reinforcement learning and continuous learning, these systems autonomously adapt their predictive models as new data arrives.
-
Multi-Agent Collaboration for Enhanced Efficiency: Agentic AI involves specialized agents who perform separate operations that include collecting data as well as data preparation before performing analysis and visualization.
By using multiple agents for collaboration, companies can achieve both scalability and resilience in their operations. The collaborative framework instructs agents to perform different duties such as cleaning raw data and conducting predictive analysis tasks.
-
Seamless Integration and Continuous Adaptation: AI agents are designed to integrate effortlessly with existing systems via APIs and middleware. By connecting to big data platforms (such as AWS Kinesis or Snowflake) and enterprise systems, they ensure coherent data exchange and workflow orchestration.
Successful Implementations of AI Agents in Data-Driven Decision Support
Several industries have successfully adopted agentic AI systems to revolutionize their decision support and data analysis capabilities. Here are notable examples:
-
AI-Driven Data Cleaning (Google Cloud Dataprep): Google Cloud Dataprep automates data preparation by detecting anomalies and inconsistencies in large datasets. This reduces manual effort, enhances data accuracy, and speeds up decision-making.
-
Real-Time Customer Insights (Netflix): Netflix uses AI to analyze user behavior, viewing history, and preferences, optimizing content recommendations. This data-driven approach improves customer engagement, retention, and satisfaction.
-
AI-Powered IT Service Management (ServiceNow Virtual Agent): ServiceNow's AI-powered Virtual Agent automates IT service requests, troubleshooting, and data analysis. It helps organizations streamline workflows, reduce manual workload, and improve operational efficiency.
-
Supply Chain Data Optimization (Siemens): Siemens leverages AI to analyze supply chain data, predict potential disruptions, and optimize inventory levels. This enhances logistics efficiency, reduces costs, and improves overall supply chain management.
-
Financial Data Analysis (BlackRock's Aladdin): BlackRock’s Aladdin platform uses AI to process vast financial datasets, assess market risks, and recommend investment strategies. This enables asset managers to make data-driven investment decisions with greater accuracy.
Benefits of AI Agents in Data-Driven Decision-Making
Using agentic AI for decision support delivers various important advantages to organizations.
Faster and More Accurate Decisions – Advanced algorithms process large datasets in real time, identifying trends and insights instantly. This eliminates human errors, reduces biases, and enables quick, data-driven choices.
Reduced Operational Costs – Automation minimizes manual effort in data collection, cleaning, and analysis, leading to significant cost savings. Businesses can allocate resources more efficiently while improving productivity.
Personalized Customer Experiences – Intelligent systems analyze user behavior to deliver tailored recommendations, enhancing engagement and satisfaction. This is widely used in industries like e-commerce, banking, and entertainment.
Improved Risk Management – Predictive analytics detect fraud, cybersecurity threats, and financial risks before they escalate. Organizations can proactively implement mitigation strategies and strengthen security.
Continuous Learning and Improvement – Machine learning models adapt over time, refining decision-making processes and optimizing operations. This ensures businesses stay competitive by evolving with market trends.
Final Thoughts on Data-Driven Decision Support
The transition to Agentic AI is transforming decision support by enabling businesses to operate with greater efficiency, precision, and adaptability. Unlike traditional systems, AI-driven decision-making automates complex processes, analyzes vast datasets in real time, and minimizes human biases. This shift allows companies to respond faster to market changes, optimize operations, and improve customer experiences.
As AI continues to evolve, its impact on strategic planning, tactical execution, and operational efficiency will grow, making data-driven decisions more intelligent and proactive. Organizations that embrace Agentic AI will gain a competitive edge, driving innovation and long-term business success in a fast-changing landscape.
Next Steps with Agentic AI
Talk to our experts about implementing Agentic AI systems and discover how industries leverage decision intelligence to become truly data-driven. Enhance workflows, automate decision-making, and optimize IT operations for greater efficiency, accuracy, and responsiveness in a competitive landscape.