Key Insights
AI agents are transforming the management of autonomous transportation fleets by optimizing operations, enhancing efficiency, and improving safety. These intelligent systems enable real-time decision-making, route planning, and vehicle coordination, reducing human intervention while maximizing fleet productivity. As autonomous vehicles become more prevalent, AI-driven solutions will be key to revolutionizing the transportation industry.
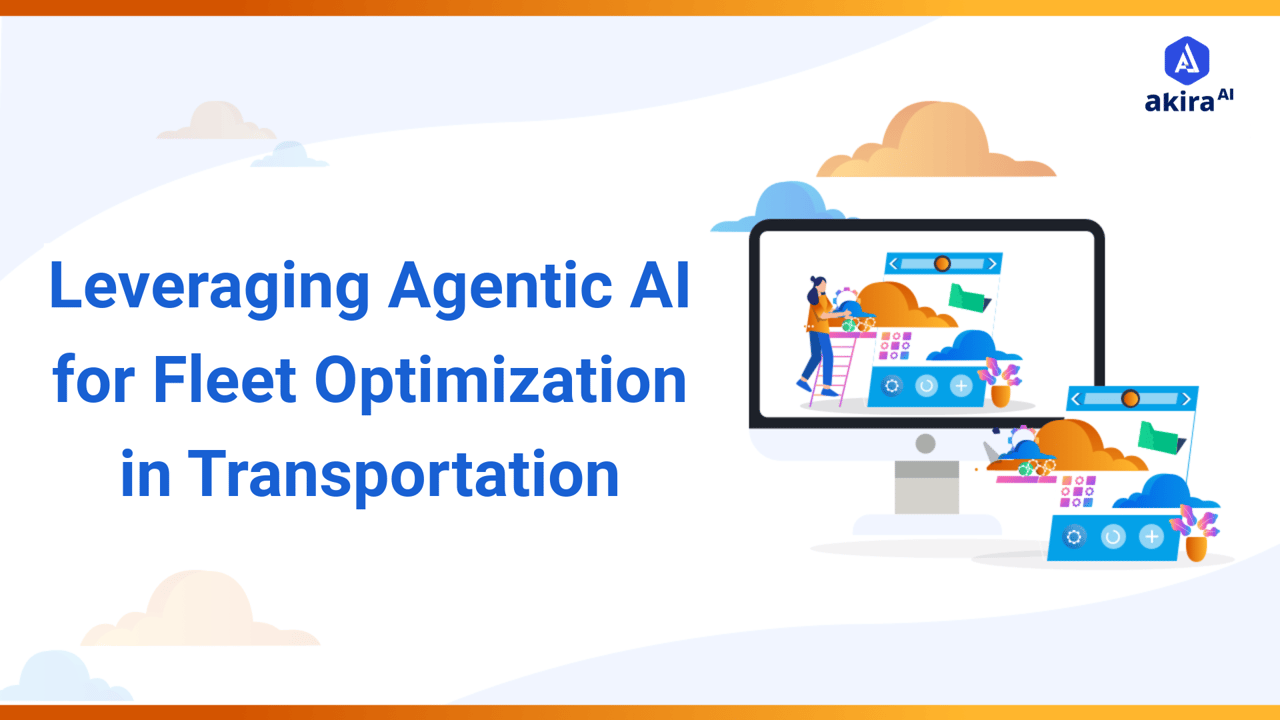
Imagine a fleet where every vehicle operates at peak performance with minimal human intervention, making fleet management more efficient than ever before. Thanks to AI agents for autonomous fleet management, this vision is becoming a reality. These intelligent systems are used to perform several complex operations, including live routing optimization, equipment maintenance planning, and decision-making, helping the fleets to operate efficiently and on schedule.
By utilizing vast amounts of data, these agents continually analyze and optimize fleet operations, making them not only more efficient but also highly cost-effective. The integration of AI in fleet management is reshaping the transportation industry, revolutionizing the way logistics and operations are managed, and driving the industry into a more innovative, more sustainable future.
What is Autonomous Fleet Management in Transportation?
Autonomous fleet management refers to the integration of advanced technologies, including agentic AI, to oversee and optimize transportation fleets. The implementation of this system makes AI agents responsible for mission-critical tasks such as dynamic route Optimization, vehicle scheduling and fuel management, and prediction of maintenance needs, among others, with minimal human interference.
The implementation of information collected from automobiles and traffic, sensors, and other systems in autonomous fleet management provides an opportunity to analyze the situation in real-time, which results in better effective utilization in terms of cost. The escalation of these processes not only aids managers in more effectively making decisions but also increases safety, cost reduction, and orchestration among the intelligent agents scattered over the fleet.
A Brief Overview of Automated Autonomous Fleet Management in Transportation
Through real-time data collected from sensors, GPS systems, and cameras, autonomous agents in fleet management take on critical responsibilities, such as route planning, vehicle diagnostics, and fuel management. By continuously analyzing this data, these intelligent agents can make timely decisions to enhance operational efficiency. As a result, autonomous fleet management reduces human intervention, lowers costs, and improves fleet performance.
In autonomous fleet management, these agents collaborate to handle tasks previously managed by human drivers or dispatchers. All these agents collaborate to improve the effectiveness and safety of fleet operations through the execution of automated practices like planning, real-time routing, and management of vehicle service. With the help of progressive AI in transportation, fleets can foretell problems that are associated with predictive maintenance and avoid vehicle breakdowns and related time off.
Traditional vs. Agentic AI Autonomous Fleet Management
Aspect |
Traditional Fleet Management |
Agentic AI Fleet Management |
Human Involvement |
High human involvement in scheduling, route planning, and decisions. |
Low human involvement; AI agents manage most operations autonomously. |
Route Optimization |
Reactive, based on historical data. |
Proactive, with real-time, AI-driven route optimization. |
Predictive Maintenance |
Limited to scheduled checkups and basic diagnostics. |
Advanced AI-driven predictive maintenance. |
Operational Efficiency |
Lower efficiency due to manual processes and delays. |
Higher efficiency with continuous optimization by AI agents. |
Safety Management |
Relies on driver skill and awareness. |
AI-powered safety protocols and real-time hazard detection. |
Cost Efficiency |
Higher costs due to inefficiency and manual labor. |
Lower costs through optimized routes, reduced downtime, and predictive maintenance. |
Data Integration |
Limited data integration and basic GPS systems. |
Comprehensive integration of real-time data from multiple sources. |
Scalability |
Limited scalability, requiring more human resources. |
Highly scalable with minimal human intervention. |
Akira AI: Multi-Agent in Action
In the case of Akira AI, a multi-agent system is used for fleet management whereby the different sub-intelligent agents are self-organized to undertake unique tasks. This system is anchored on the Master Orchestrator, which controls the movement and activities of all agents in the system. Below is a breakdown of the agents used in Akira AI’s system:
-
Master Orchestrator: The Master Orchestrator acts as the central control unit, ensuring seamless communication among all agents. It guarantees that all tasks are synchronized for optimal fleet performance.
-
Route Optimization Agent: The Route Optimization Agent processes traffic information, current weather conditions, and road closures on an ongoing basis. It optimizes or makes changes to the routinary flow of the vehicles so as to select the best paths.
-
Maintenance Prediction Agent: Using AI algorithms, the Maintenance Prediction Agent predicts potential vehicle issues by analyzing historical data and vehicle health. It allows for proactive maintenance, minimizing unexpected breakdowns.
-
Fuel Efficiency Agent: The Fuel Efficiency Agent constantly observes fuel consumption and makes recommendations for use based on the driver's usage and road conditions. It assists in cutting down fuel expenses by encouraging the attainment of efficient driving.
-
Driver Assistance Agent: While fully autonomous vehicles are being developed, the Driver Assistance Agent supports semi-autonomous vehicles with real-time navigation and safety assistance. It also helps during load management and makes work easier for the human drivers to handle.
-
Performance Monitoring Agent: The Performance Monitoring Agent gives feedback and makes adjustments in real-time for the vehicle to perform to the optimal level. It is used to achieve and sustain efficient operating conditions throughout the fleet. It helps maintain optimal operational standards across the fleet.
Use Cases of Autonomous Fleet Management
Autonomous fleet management is gaining significant traction in the transportation industry with various use cases and applications:
Real-Time Dynamic Route Optimization: AI agents can follow through with traffic and environmental situations to find the best routes with less time on the road and less fuel consumption.
Predictive Maintenance and Early Repairs: By leveraging AI-driven analytics, fleets can predict when maintenance is needed, thus avoiding costly repairs and minimizing downtime.
Cost Reduction Through Operational Efficiencies: Autonomous agents can help cut operational costs by reducing the need for human drivers, improving fuel efficiency, and minimizing vehicle downtime through predictive maintenance.
Driverless Vehicles Transforming Fleet Operations: The future of autonomous transportation involves driverless trucks. These vehicles use AI in fleet management to navigate, plan routes, and monitor vehicle performance without human intervention.
Enhancing Safety with AI Monitoring: Autonomous agents ensure safety by continuously monitoring the vehicle's surroundings and responding to potential hazards in real time.
The Operational Benefits of Autonomous Fleet Management
Autonomous fleet management with the multi-agent system provides numerous operational benefits, including the following:
-
Improved Productivity with AI Automation: These agents assist in improving efficiency by allowing tasks related to scheduling and management to become automated. This results in greater fleet utilization and saves operators time when they need to take on more critical management tasks, thereby enhancing output.
-
Significant Cost Reduction Strategies: With predictive maintenance, autonomous vehicles can prevent unexpected breakdowns, thus reducing costly repairs and vehicle downtime.
-
Enhanced Efficiency through Dynamic Routing: Autonomous agents use sophisticated means to calculate the best shifts and lanes so that fuel consumption will be limited, and delivery will be speedy. Real-time optimization allows for precise considerations to be made in order to achieve optimum returns for the entire fleet.
-
Reduced Human Errors in Operations: By using AI to control operations, companies can reduce human errors that lead to inefficiency, safety incidents, and operational delays.
-
Scalable Growth Without Extra Labor: Autonomous fleet management allows transport corporations and companies to expand their businesses without increasing requirements for human employment. This results in cost-effective expansion.
-
Improved Safety with Real-Time Monitoring: With constant monitoring and real-time hazard detection, AI agents significantly enhance safety and reduce accident rates, improving the overall safety of both vehicles and personnel.
Technologies Transforming Autonomous Fleet Management in Transportation
Several key technologies are driving the growth of autonomous fleet management systems:
-
AI and Machine Learning Integration: These technologies help predict vehicle behavior, optimize routes, and prevent failures before they occur.
-
Leveraging IoT for Data Integration: Real-time data from vehicles, sensors, and other sources are integrated into fleet management systems for more accurate decision-making.
-
Role of Autonomous Vehicles in Transformation: The development of self-driving trucks and delivery vehicles is a major part of the transformation in autonomous fleet management.
-
Faster 5G Connectivity for Communication: With faster data transmission speeds, 5G enhances communication between autonomous vehicles, fleet managers, and AI agents.
-
Big Data Analytics Enhancing Decision Making: AI systems can analyze a variety of data and make projections, thereby enhancing decision-making and efficiency of fleet management operations.
The Future of Automated Autonomous Fleet Management in Transportation
-
Full Autonomy in Fleet Operations: By 2030, many fleets will operate fully autonomously, eliminating the need for human drivers.
-
Increased AI Integration for Complex Decisions: As AI continues to evolve, AI agents will become even more intelligent, handling complex decision-making tasks in real-time.
-
Sustainability Focus with Electric Vehicles: Autonomous fleet management systems will likely incorporate electric vehicles, reducing carbon footprints in the transportation industry.
-
Predictive Maintenance Reducing Fleet Downtime: Predictive maintenance will become more advanced, allowing companies to address potential issues before they cause downtime.
-
Global Expansion of Autonomous Fleet Systems: As AI in fleet management proves its value, autonomous fleet systems will become commonplace in both developed and emerging markets.
Conclusion: Autonomous Fleet Management in Transportation with Agentic AI
The growing adoption of AI agents for autonomous fleet management signals the dawn of a new era in the transportation industry. With agentic AI driving change, businesses can look forward to more efficient and optimized fleet operations. These intelligent systems are transforming the way fleets are operated, resulting in enhanced efficiency and reduced operational expenses.
As these agents continue to evolve, fleet operators will benefit from better route planning, predictive maintenance, and smarter decision-making capabilities, all of which translate into more streamlined operations and enhanced service delivery. The future of fleet management is increasingly automated, and AI agents are at the core of this exciting transformation.