Key Insights
Agentic AI-driven sentiment analysis helps brands quickly understand customer preferences and pain points by analyzing feedback from diverse sources. This real-time insight enables businesses to adapt marketing strategies, improve product offerings, and boost customer satisfaction. With AI agents, companies can identify emerging trends, optimize operations, and foster deeper customer loyalty.
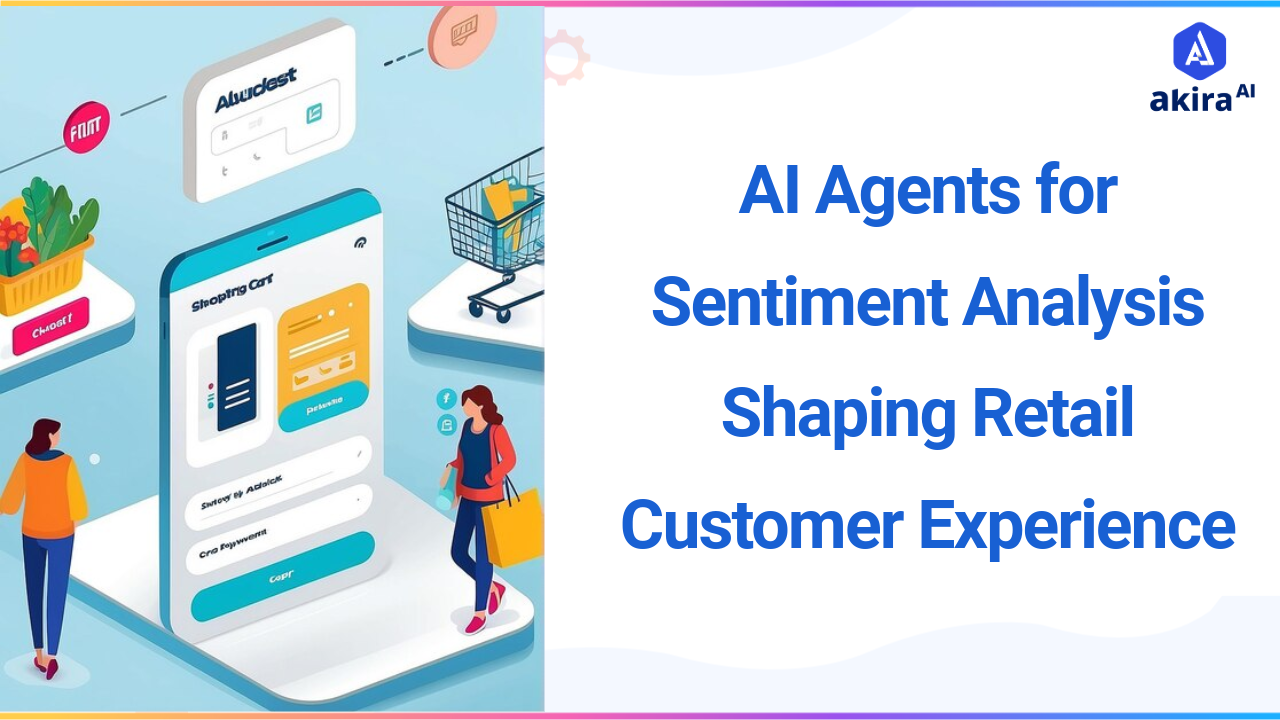
Imagine a consumer walking into a store, looking at a shelf filled with competing packaged products, each promising the best quality and value. Behind the scenes, a powerful tool is working to help brands understand not just what this consumer buys but why they make their choices. One leading brand in the retail packaged products sector recently tapped into this technology, using AI teammates to track customer sentiment across social media, product reviews, and surveys. This insight allowed the brand to pivot its marketing strategy and design future products better aligned with consumer values, significantly boosting customer loyalty and sales.
This blog will explore how AI Agents revolutionise customer sentiment analysis in retail packaged products. This helps brands quickly adapt to market changes, improve their offerings, and build deeper connections with consumers.
What is Customer Sentiment Analysis?
Customer sentiment analysis involves understanding customer opinions, emotions, and attitudes toward a brand, product, or service by reviewing feedback from sources like social media, surveys, and product reviews. It classifies customer sentiments as positive, negative, or neutral, helping businesses identify trends, address issues, and improve their offerings. Businesses can refine their strategies, enhance customer satisfaction, and build stronger relationships by analysing what customers value or dislike. In a competitive market, sentiment analysis enables companies to stay connected with customer needs and respond effectively to changes in preferences or expectations.
![]()
Key Concepts of Customer Sentiment Analysis
Sentiment Classification: Using techniques like Natural Language Processing (NLP) to categorize customer feedback into positive, negative, or neutral sentiments, providing a clear understanding of how customers feel about a product or brand.
Consumer Emotions & Preferences: Analyzing consumer feelings toward product attributes such as taste, packaging, price, and availability to understand what drives purchasing decisions and brand loyalty.
Data Sources: Collecting feedback from diverse channels like reviews, social media, and surveys, offering a comprehensive view of customer sentiment across various touchpoints.
Real-Time Insights: Providing immediate feedback on customer sentiments, allowing businesses to act quickly, address concerns, and capitalize on emerging trends.
Trend Identification: Identifying long-term shifts in consumer sentiment to anticipate market changes, adapt strategies, and maintain a competitive edge.
Traditional Way of Customer Sentiment Analysis
-
Focus Groups: Focus groups are small, guided discussions to gather in-depth feedback on products or services. These provide qualitative insights but are limited in sample size and diversity. The results often may not represent broader customer sentiment.
-
Manual Reviews: Manual reviews involve businesses collecting feedback from various sources, such as emails or product reviews. Although valuable, this method is labour-intensive and prone to human bias or error. Analyzing large amounts of feedback manually can lead to missed insights.
-
Limited Scale: Traditional methods often capture feedback from a small, non-representative group of customers. This limits the scope and diversity of insights, making it difficult to generalize findings across different regions or demographics. The narrow focus may skew decision-making.
-
Slow Results: The time-consuming nature of surveys, focus groups, and manual reviews means results can take weeks or months to process. This delays businesses' ability to act on customer feedback quickly. In fast-moving markets, such delays can hinder competitiveness.
-
Inefficient for Fast-Paced Markets: Traditional methods cannot keep up with the rapid changes in customer behaviour or market trends. They lack the speed and scalability needed to provide real-time insights, leaving businesses unable to respond quickly to emerging opportunities or issues.
Challenges in Traditional Sentiment Analysis
-
Limited Reach and Scalability: Traditional methods are typically constrained to small datasets, which means businesses miss out on insights from larger or diverse customer groups, including different regions or demographics. This creates a narrow view of customer sentiment, limiting the ability to cater to a broad audience effectively.
-
Delayed Insights: Survey and focus group results take time to collect, analyze, and interpret, often leading to delayed insights. This reduces a company’s ability to react to customer concerns and market shifts, which is critical in a highly competitive industry.
-
Bias and Inaccuracy: Manual analysis, whether through surveys or focus groups, can be prone to human biases, such as personal interpretations or selective sampling. These biases reduce the accuracy and reliability of the insights, potentially skewing business decisions.
-
Costly Operational Demands: Traditional methods involve significant manual effort, from organizing focus groups to processing survey data, which leads to high operational costs. These resources could be better allocated to more efficient processes, leading to better profitability.
Agentic AI is revolutionizing customer service in logistics by automating routine inquiries, providing real-time support, and enhancing operational efficiency for improved customer satisfaction. Click to discover more about Customer Analysis.
Impact on Customers Due to Traditional Sentiment Analysis
-
Delayed Response to Feedback: Traditional sentiment analysis often leads to slow responses to customer feedback, causing dissatisfaction. If issues such as poor product quality or bad customer experience are only addressed after significant delays, it could lead to frustrated customers who feel unheard or ignored.
-
Generic Offerings: Without personalised insights, businesses couldn't tailor products or marketing strategies to specific customer preferences. This resulted in less targeted offerings that might not resonate with the broader consumer base, affecting customer loyalty and engagement.
-
Missed Opportunities for Improvement: Traditional methods couldn’t always detect emerging trends or new customer demands. Businesses might have missed the opportunity to innovate or adapt to changes in consumer behaviour, leaving them vulnerable to competitors who were more agile in responding to trends.
Akira AI: Multi-Agent in Action
Fig1: Architecture Diagram of Customer Sentiment Analysis
-
Data Collection Agent: This agent collects unstructured customer feedback from various sources such as social media platforms (e.g., Twitter), product reviews (e.g., Amazon), and customer surveys. It acts as the entry point for all customer sentiment data.
-
Preprocessing Agent: This agent prepares the collected data for analysis by filtering out irrelevant data, handling missing values, and organizing it into a structured format. This ensures that only relevant and clean data is passed on for sentiment analysis.
-
Sentiment Analysis Agent: The NLP techniques used by this agent classify customer feedback into different sentiment categories (positive, negative, or neutral). This helps determine the general sentiment of customers towards a product or brand.
-
Insight Generation Agent: After sentiment classification, this agent aggregates the data to detect emerging trends, common pain points, and potential opportunities. For example, it can recognize patterns like a growing preference for eco-friendly packaging.
-
Action Recommendation Agent: Based on the insights generated, this agent suggests actionable strategies to improve customer satisfaction, product offerings, or marketing strategies. For instance, the system might recommend expanding the eco-friendly product line if there is a significant positive sentiment.
This multi-agent system ensures efficient, scalable, real-time sentiment analysis for businesses, empowering them to quickly and effectively act on customer feedback.
Prominent Technologies in the Space of Sentiment Analysis
-
Natural Language Processing (NLP): NLP analyzes text data, identifying patterns in language to detect sentiment, classify emotions, and extract insights, enabling businesses to understand customer feedback and trends effectively.
-
Machine Learning (ML): ML algorithms learn from new data continuously, improving the accuracy of predictions and sentiment analysis over time, allowing systems to adapt to evolving customer behaviours and preferences.
-
Cloud Computing: Cloud computing provides scalable infrastructure for storing and processing large volumes of data. It enables businesses to analyze customer sentiment efficiently without the limitations of on-premises systems, ensuring flexibility.
-
Big Data Analytics: Big data analytics processes vast amounts of structured and unstructured data, uncovering deep insights into customer behaviour and preferences. This enables businesses to identify trends and make informed, data-driven decisions.
Future Trends: How AI Agents Supersede Other Technologies
-
Real-Time Insights at Scale: Modern AI-driven systems provide real-time sentiment analysis across multiple channels, offering businesses immediate access to insights. This contrasts with traditional methods that rely on slower, manual processes, allowing businesses to respond quickly to shifts in customer sentiment.
-
Automation and Data Collection: These advanced systems automatically gather and process data from various touchpoints, such as reviews, social media, and IoT devices. This streamlined data collection allows businesses to analyze a much broader scope of feedback without delays or manual effort.
-
Handling Unstructured Data: AI technologies excel at processing unstructured data, such as free-form text or social media posts, that traditional methods often struggle with. This capability allows for a more nuanced understanding of sentiment, capturing insights that are harder to identify with basic keyword searches.
-
Identifying Emerging Trends: By analyzing ongoing interactions and feedback, AI systems can detect emerging sentiment trends in real-time. Unlike traditional methods, which often analyze data retrospectively, AI can provide proactive insights, allowing businesses to adjust strategies before issues escalate.
-
Personalized Recommendations: With the ability to analyze individual customer interactions, AI systems can offer tailored recommendations. These personalized insights help businesses improve their products, services, or marketing efforts, ensuring they meet customer needs more effectively.
Successful Implementations of AI Agents in Customer Sentiment Analysis
-
E-commerce Platforms (e.g., Amazon, eBay): Leverage sentiment analysis to track customer feedback from product reviews, refine product offerings, and personalize marketing campaigns, leading to better customer engagement and satisfaction.
-
Retail Chains (e.g., Walmart, Target): Use sentiment analysis to monitor customer satisfaction with product lines, detect early signs of dissatisfaction, and tailor promotions accordingly, enhancing customer loyalty and retention.
-
Social Media Platforms (e.g., Facebook, Twitter, Instagram): Employ sentiment analysis tools to monitor user interactions and detect negative sentiment in real-time, enabling proactive management of public relations and resolution of potential issues before escalation.
-
Customer Service Providers (e.g., Zendesk, Freshdesk): Integrate sentiment analysis into their systems to assess customer satisfaction from support tickets and calls, prioritize urgent issues, and enhance agent responses with real-time insights, improving overall service quality.
-
Travel and Hospitality (e.g., Airbnb, Booking.com): Analyze guest reviews and feedback through sentiment analysis to identify sentiment trends regarding property listings, improve personalized recommendations, and adjust services to meet customer expectations, ensuring a better guest experience.
Conclusion: AI Agents for Customer Sentiment Analysis
In conclusion, AI-powered sentiment analysis transforms how retail packaged product brands engage with customers. By leveraging real-time insights from diverse data sources, businesses can better understand customer preferences, address concerns promptly, and tailor their offerings for greater satisfaction. With AI agents handling large volumes of unstructured data, brands are improving operational efficiency and gaining a competitive edge in the fast-paced market. As consumer expectations evolve, adopting AI-driven sentiment analysis will be crucial for brands to stay agile, innovative, and connected with their audience, driving long-term success.