Key Insights
Agentic AI is transforming drug discovery by speeding up research, reducing costs, and enhancing precision. It automates data analysis, predicts risks, and optimizes clinical trials for better outcomes. With personalized medicine, AI helps create treatments tailored to individual needs. The future promises more efficient and successful drug development, benefiting healthcare worldwide.
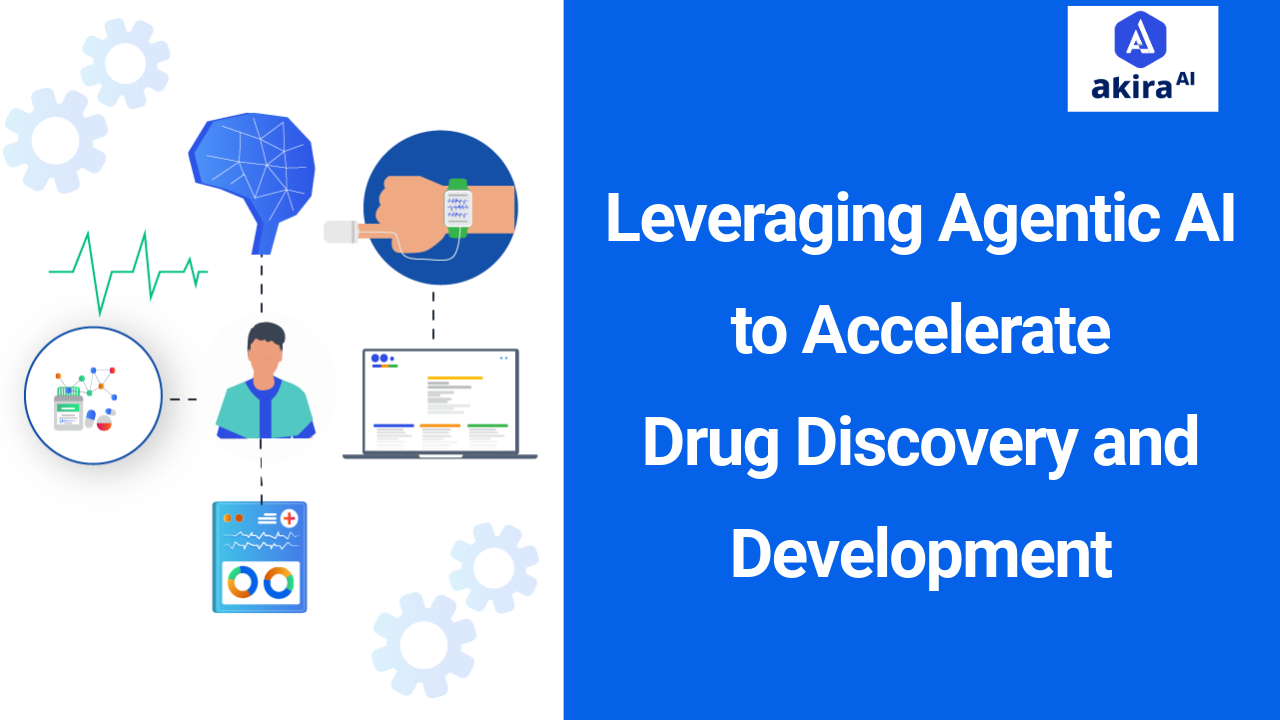
The journey of drug discovery and development is a cornerstone of medical advancement, aiming to create innovative treatments that transform Healthcare. However, this process is often lengthy, complex, and resource-intensive, involving years of research and collaboration across multiple scientific disciplines. Enter Agentic AI—an emerging technology poised to revolutionize how drugs are discovered and developed.
By leveraging intelligent agents, AI systems can accelerate target identification, optimize lead compounds, and predict therapeutic outcomes with unprecedented precision. These AI agents-driven tools integrate vast datasets from genomics, chemical libraries, and clinical studies, streamlining decision-making and reducing time-to-market for critical medicines. In this blog, we explore how Agentic AI is reshaping the pharmaceutical landscape, enhancing efficiency, and paving the way for breakthroughs in healthcare innovation.
What is Drug Discovery and Development?
Drug discovery identifies potential therapeutic compounds that can modulate biological processes to treat or manage diseases. It involves extensive research to understand disease mechanisms, identify molecular targets (such as proteins or genes), and design or screen compounds that interact effectively with these targets.
Drug development follows the discovery and encompasses all the steps necessary to turn a promising compound into an approved medication. This phase involves preclinical testing (laboratory and animal studies), clinical trials (testing in humans across multiple phases for safety and efficacy), and regulatory approval.
Drug discovery and development are essential in bringing safe, effective, and innovative therapies to patients, though they are often lengthy and resource-intensive processes. Advanced technologies, like AI, are increasingly being used to optimize and accelerate these stages.
A Brief Overview of Agentic AI in Drug Discovery and Development
Agentic AI represents a revolutionary shift in drug discovery and development by enabling systems to autonomously make decisions and adjust strategies based on incoming data. In the context of drug development, these AI agents process large amounts of complex data from sources such as genomics, chemical libraries, and clinical trials, helping researchers identify promising drug candidates much faster than traditional methods. This acceleration reduces the time from discovery to clinical trials and ensures that the most viable drug candidates move forward with higher accuracy.
The integration of AI Teammates also enhances the precision of drug design and patient-specific treatments. By analyzing patterns and relationships within diverse datasets, these intelligent agents can optimize drug compounds, predict potential side effects, and design clinical trials more effectively. With the ability to personalize treatments based on individual patient data, agentic AI is transforming drug development into a more efficient, cost-effective, and tailored process, ultimately improving healthcare outcomes.
Traditional vs. Agentic AI Drug Discovery and Development
Aspect of Drug Discovery |
Traditional Method |
Agentic AI Approach |
Speed of Discovery |
Slow, often takes years to identify viable candidates |
Fast, AI can process and analyze data much quicker |
Cost of Development |
Expensive, often exceeds $1 billion for a new drug |
More cost-effective by reducing trial and error |
Success Rate |
Low, many compounds fail in clinical trials |
Higher success rate by identifying more effective candidates |
Data Analysis |
Relies on human experts to interpret data |
AI automates data analysis, uncovering hidden patterns |
Personalization |
Limited ability to tailor treatments to individuals |
Can develop personalized medicine based on genetic data |
Akira AI: Multi-Agent in Action for drug discovery and development
Fig1: Architecture Diagram of Drug Discovery and Development
The key aspect of Agentic AI is that it can process vast amounts of information within a short period and provide intelligence about the behavior of the driver and the vehicle.
-
Data Collection Agent: The process begins with the Data Collection Agent, responsible for aggregating data from diverse sources such as preclinical studies, clinical trials, and genomic databases. This agent ensures that all the data is accurate, validated, and harmonized for further processing. By creating a centralized repository of reliable data, this step enables precise analysis and decision-making across the drug discovery pipeline.
-
Behavioral & Mechanism Analysis Agent: The Behavioral & Mechanism Analysis Agent focuses on investigating the biological underpinnings of diseases and patient behavior. It extracts actionable insights by studying relationships between drug mechanisms, disease progression, and clinical outcomes. These analyses help uncover patterns that guide the development of targeted and effective therapies.
-
Risk Prediction Agent: The Risk Prediction Agent evaluates potential risks associated with drug candidates, such as adverse effects or suboptimal therapeutic responses. It leverages predictive models to analyze aggregated data and estimate the likelihood of treatment failures or complications. This proactive assessment minimizes risks and enhances the safety profile of drug candidates.
-
Optimization & Personalization Agent: The Optimization & Personalization Agent refines treatments to improve their effectiveness and efficiency. By tailoring interventions to individual patient data, this agent ensures personalized therapeutic strategies. It also optimizes drug formulations and dosages to maximize outcomes while minimizing side effects, aligning with the principles of precision medicine.
-
Decision Support & Feedback: All insights are consolidated into a decision support module, which guides stakeholders (clinicians, researchers) in making informed decisions. Following this, the Feedback & Recommendations Agent refines the outcomes, feeding data back to the Performance Monitoring Agent, which oversees:
5.1 Clinical Trial Refinement: Improving trial designs for better outcomes.
5.2 Drug Compound Optimization: Enhancing the efficiency and safety of drug formulations.
Use cases of Drug Discovery and Development
-
Accelerating Drug Candidate Identification: AI agents analyze large datasets of chemical compounds, genomic sequences, and biological interactions to identify potential drug candidates. By predicting compound effectiveness and toxicity early, these agents significantly reduce the time required for initial discovery.
-
Personalized Drug Development: Optimization and personalization agents design drugs tailored to individual genetic and clinical profiles. This precision medicine approach enhances treatment efficacy and minimizes side effects, improving patient outcomes.
-
Clinical Trial Optimization: Agents evaluate clinical trial designs to identify the most effective patient cohorts, predict trial success rates, and monitor real-time data for mid-trial adjustments. This improves trial efficiency and ensures higher success rates.
-
Predicting Adverse Drug Reactions: Risk prediction agents use historical and real-time data to identify potential side effects or interactions of drug candidates. This enables pharmaceutical companies to mitigate risks early and improve drug safety profiles.
-
Virtual Screening for Drug Candidates: Behavioral and mechanism analysis agents perform virtual simulations to screen thousands of chemical compounds. This eliminates the need for exhaustive physical experiments and narrows down promising candidates for further testing.
-
Improving Rare Disease Research: AI agents analyze genomic and clinical data to develop treatments for rare diseases, where traditional methods face limited data and financial constraints.
The Operational Benefits of Drug Discovery and Development
The application of Agentic AI in the pharmaceutical industry is not limited to enhancing the drug discovery processes; it also brings about major operational advantages such as the following:
-
Accelerated Research and Development: Machine learning models rapidly process large datasets to identify promising drug candidates and predict how compounds will interact with biological systems. This reduces the time required for research, speeding up the journey from initial discovery to clinical trials. As a result, the overall drug development timeline is significantly shortened.
-
Enhanced Accuracy and Precision: Advanced algorithms model molecular structures and biological pathways, allowing for more accurate predictions of how drugs will behave in the body. This precision minimizes human error and helps select the most viable drug candidates. It ensures that only promising compounds proceed to the next stages of development.
-
Cost Efficiency: AI Agents reduce the financial burden of drug development by quickly identifying effective compounds and streamlining experimental processes. Predicting clinical outcomes and eliminating inefficient steps, lowers the risk of failed trials. This leads to a decrease in the cost of bringing new drugs to market.
-
Improved Risk Management: Predictive models assess potential risks such as adverse reactions, clinical trial failures, and ineffective treatments early in the process. By analyzing genetic data and chemical properties, the system identifies red flags before they become major issues. This proactive approach reduces the likelihood of costly late-stage failures.
-
Optimized Clinical Trials: AI aids in selecting the right patients and designing more effective trial protocols based on real-time data analysis. It monitors trial progress, adjusting protocols as necessary to improve accuracy and efficiency. This ensures that trials are completed more quickly and with better outcomes.
Technologies Transforming Agentic AI in Drug Discovery
Several trends in technology are likely to augment agentic AI in the context of drug discovery:
-
Machine Learning: Algorithms that enable machines to improve their predictions based on the provided data. Such algorithms are central to the processes of studying the activity of large volumes of biological and chemical information for drug discovery.
-
Natural Language Processing: Natural language processing enables machines to read and comprehend scientific papers, thus facilitating the search for potential drug targets, drug interactions, and how they work.
-
Quantum Computing: It is a new field of science that is reshaping drug research, by enabling AI to resolve intricate issues which has long been impossible with classical computing. Facilitates faster simulations and enhances the drug’s design.
-
Genomic Data Analysis: The growing capacity of AI to handle extensive genomic information is accelerating the development of medications that are based on the particular genes of the people they work with, which increases the effectiveness of precision medicine.
The Future Trends of Agentic AI in Drug Discovery
The integration of Agentic AI in drug discovery is revolutionizing the healthcare industry, with significant advancements expected in the coming years. Here are some key trends to watch for:
-
Hyper-Personalized Drug Development: The future of drug development will focus on creating personalized treatments tailored to individual patient profiles, incorporating genetic, molecular, and environmental data. This approach is expected to lead to more effective therapies, with fewer side effects and quicker approval timelines.
-
Integration with Genomics and Precision Medicine: Combining advanced analytics with genomic sequencing will allow researchers to identify more accurate drug targets. This integration will streamline the process of analyzing genetic variations and improving drug efficacy, accelerating the development of precision medicine.
-
Real-Time Drug Discovery and Optimization: Future systems will be able to analyze ongoing research and clinical trial data in real-time, optimizing drug formulations and treatment plans instantly. This will reduce development cycles and allow for rapid adjustments based on emerging findings.
-
Expanded Role in Clinical Trials: Advanced technologies will significantly enhance clinical trials by improving patient selection, trial design, and predicting outcomes. This will increase the success rate of trials while reducing recruitment time and improving overall efficiency.
-
Collaboration Between Technology and Researchers: Rather than replacing human researchers, these technologies will complement them by handling data processing and predictive modeling, allowing researchers to focus on strategy, decision-making, and creative problem-solving. This collaboration will speed up the innovation and drug development process.
Conclusion: AI Agents for Drug Discovery and Development
The integration of agentic AI in drug discovery and development marks a transformative shift in the pharmaceutical industry. By enhancing speed, precision, and cost-efficiency, these intelligent systems enable the discovery of more effective and personalized treatments. With the ability to analyze vast datasets, predict risks, and optimize clinical trials, AI-driven technologies are reshaping how drugs are developed and brought to market. As advancements in AI continue, the future of drug discovery looks promising, offering the potential for breakthrough therapies that will significantly improve patient outcomes and revolutionize healthcare worldwide.
Explore More Agentic AI Virtual Assistant