Key Insights
Agentic AI is transforming customer churn prediction in banking by delivering real-time insights and precise risk assessments. Through agentic workflows, banks can proactively identify at-risk customers using vast datasets and advanced machine learning. This enables tailored retention strategies that enhance customer satisfaction and loyalty. The integration of technologies like natural language processing and big data analytics within these workflows further empowers banks to effectively address churn and optimize their customer relationships.
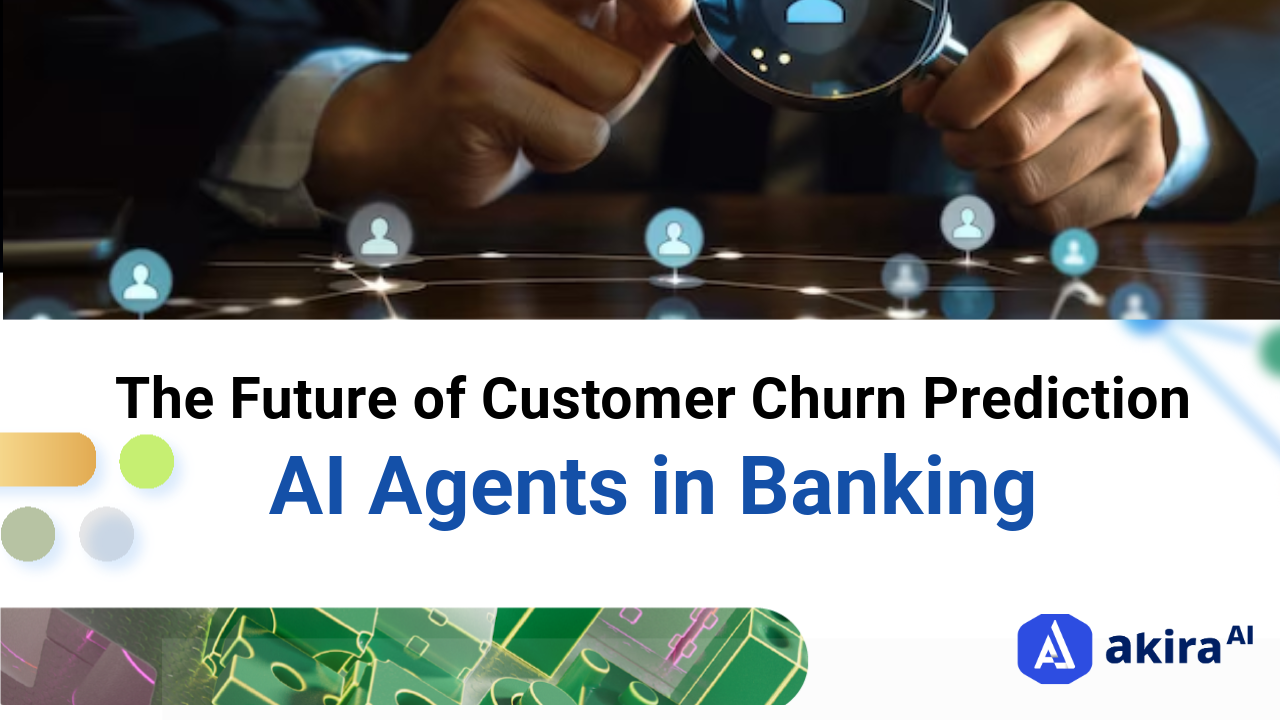
Customer churn prediction is a critical process in the banking sector, enabling banks to identify customers who are likely to leave and stop using their services. Churn prediction traditionally relied on manual assessment, whereby the data was analyzed concerning the customer's behavior. Because it's time-consuming, its scope as well as due to the inherent subjective nature of human judgment, at-risk customers often go undetected until it is too late. However, great strides are being taken in churn prediction and AI agents are increasingly adopted within banks. AI agents offer faster, more accurate, and scalable solutions that make it possible for banks to process real-time data and be proactive as regards identifying churn.
This blog delves into the differences between AI agents when predicting customer churn, where they sit in comparison to traditional methods and what makes them a great advantage in real banking applications.
What is Customer Churn Prediction?
Customer Churn Prediction is the process by which customers stop using a company's products or services, losing business. This is an extremely important metric for businesses because high churn rates could point to dissatisfaction and competition, or even an awfully bad customer experience. Predictive and preemptive measures of churn help organizations retain customers, protect revenue, and boost loyalty altogether by identifying those most likely to leave and implementing retention strategies especially targeted at those customers.
Brief Overview of Customer Churn in Banking
In banking, customer churn prediction is essential for maintaining strong relationships with clients and minimizing the loss of valuable customers. As competition intensifies and customer expectations rise, predicting which customers are at risk of leaving becomes increasingly important. Churn prediction utilizes data analysis to identify early signs of dissatisfaction, such as reduced transaction activity or changes in service usage. By using advanced AI agents, banks can proactively address churn risks, enabling targeted retention strategies like personalized offers or improved customer support. This approach not only helps retain customers but also drives long-term profitability and customer satisfaction.
How AI Agents Function in Churn Prediction
AI agents in churn prediction work together in a coordinated manner to ensure efficient, accurate, and scalable processes. AI agents in churn prediction for banking utilize machine learning algorithms to analyze vast amounts of customer data, including transaction history, account activity, demographic information, and customer interactions.
-
Data Collection: The Data Collection Agent gathers data from internal sources (transactional records, CRM systems, customer service logs) and external sources (social media, third-party CRM tools).
-
Data Preprocessing: Once the data is collected, the Data Preprocessing Agent cleans it by handling missing values, duplicates, and outliers. Then, the data is normalized and standardized to maintain uniformity. Feature generation and selection are performed to create attributes that enhance model performance.
-
Behavioral Analysis: The Behavioral Analysis Agent analyzes customer behavior patterns, focusing on transaction history, service usage, complaints, and engagement. The agent flags any patterns that suggest a decline in engagement or an increased churn risk compared to historical data.
-
Churn Risk Profiling: The Churn Risk Profiling Agent then uses machine learning models to assess each customer's churn risk. Customers are categorized into low, medium, or high risk, with the agent identifying both the churn probability and underlying reasons for each prediction.
-
Retention Strategy: Based on the churn risk profiles, the Retention Strategy Agent triggers personalized offers and retention campaigns for high-risk customers. It designs targeted efforts such as loyalty programs, personalized offers, or fee reductions tailored to the customer’s profile.
-
Final Processing: In the final step, the system aggregates the results of retention efforts. The data from the latest churn predictions feedback into the system, enabling continuous improvement of the model and evolving the system's predictions over time.
Use Cases of AI Agents in Customer Churn Prediction
AI agents can be employed in various scenarios to improve churn prediction accuracy. Some prominent use cases include:
-
Real-time Retention Offers: AI agents monitor customer behavior in real-time, enabling banks to trigger retention offers, such as discounts or lower loan rates, when churn risk increases.
-
Customer Segmentation for Personalized Banking: By clustering customers based on risk profiles and spending patterns, banks can offer tailored services that directly address customer needs.
-
Churn Prediction for Specific Products: AI agents can predict churn for individual products like credit cards or mortgages, allowing targeted marketing efforts for at-risk segments.
-
Predictive Risk Mitigation: Detecting early warning signs like frequent ATM withdrawals or lower average balances can allow proactive engagement with customers before they leave the bank.
-
Cross-Selling and Upselling: AI agents can not only predict churn but also identify opportunities to cross-sell or upsell to customers at risk of leaving. By offering personalized product recommendations, banks can increase customer engagement and reduce churn likelihood.
The Role of Akira AI’s Multi-Agent Platform in Churn Prediction
The Akira AI agentic platform has improved the prediction of customer churn through automation in the whole process from collection of data all the way to retaining the customers. The platform uses a Master Agent which oversees all the tasks and processes, while domain-specialized agents interact with specific parts of the workflow; so the churn prediction process is completely efficient and scalable and works in real-time, without human interference.
-
Master Orchestrator: The Master Agent is responsible for the supervision of all other agents that participate in the churn prediction. They collect results from those agents, change status of the system, and track performance parameters used in the analytical work to support organizational execution throughout the framework. It is fundamental in the management of uniformity of interpretation of data and objectives and goals setting.
-
Data Collection Agent: The Data Collection Agent plays a vital role by pulling customer data from both internal and external sources. Internal sources include transactional records, CRM systems, and customer service logs, while external sources could be social media, third-party CRM tools, or publicly available customer data.
-
Data Preprocessing Agent: After data collection, the Data Preprocessing Agent takes over to clean and organize the data for analysis. It handles tasks such as removing duplicates, filling in missing values, and detecting outliers. This agent also normalizes and standardizes the data, ensuring uniformity across different data types.
-
Behavioral Analysis Agent: The Behavioral Analysis Agent focuses on analyzing customer behaviors to detect early signs of potential churn. It looks for patterns in transaction frequency, service usage, complaints, and engagement levels with the bank’s services. The agent compares current behavior to historical records and flags any decline in engagement or other churn indicators.
-
Churn Risk Profiling Agent: The Churn Risk Profiling Agent leverages advanced machine learning models to evaluate the likelihood of customer churn. It segments customers into risk categories—low, medium, or high—based on their churn probability. The agent also identifies the key drivers behind each churn risk, helping to clarify why a customer might leave.
-
Retention Strategy Agent: Once churn risk profiles are established, the Retention Strategy Agent steps in to design and execute personalized interventions. This agent triggers targeted offers or campaigns aimed at customers identified as high-risk. Retention efforts might include loyalty programs, fee reductions, or personalized product offerings.
Technologies Transforming Customer Churn Prediction
Agentic AI-driven churn prediction relies on several technologies and frameworks.
-
AutoGen Framework: The AutoGen Framework is a powerful tool for enabling automated agent orchestration. It allows the creation of autonomous systems that can generate and manage multiple AI agents in real-time.
-
Knowledge Graphs: Knowledge Graphs are a crucial technology that improves how churn prediction models understand and leverage relationships between different entities, such as customers, products, and interactions.
-
Reinforcement Learning: Reinforcement Learning (RL) is another key technology in churn prediction, where agents learn optimal strategies through trial and error, receiving feedback based on their actions. In churn prediction, RL allows agents to improve their retention strategies dynamically by evaluating the success of previous interventions.
-
Data Lakehouse: Platforms like Snowflake integrate structured and unstructured data in a scalable environment, enabling agents to access large datasets in real-time for churn prediction. Data lakehouses combine the best of data lakes and data warehouses.
-
Natural Language Processing (NLP): Natural Language Processing (NLP) is used to analyze unstructured data sources, such as customer support tickets, social media interactions, and emails, to detect dissatisfaction and early signs of churn.
Operational Benefits of AI Agents in Churn Prediction
-
Real-time Insights: AI Agents have the capability to observe customer behavior in real-time and raise probable churn risks to banks. Therefore, it helps banks respond more proactively than just waiting for the periodical report.
-
Scalability: Because artificial intelligence-based systems can process large amounts of data, even the largest of institutions are able to work effectively in processing churn prediction.
-
Personalized Retention Strategy: AI agents enable hyper-personalization in retention strategies. With analysis of customer-specific data, it may recommend offers or interventions that are specific to him and most probably result in higher retention of high-risk customers.
-
Cost Efficiency: The process through the automation of AI agents reduces human input directly and therefore, operational costs. Banks free up more resources to work on more strategic initiatives by letting agents sit back and watch the routine churn monitors.
-
Improved Predictive Skills: Artificial intelligence agents use advanced machine learning algorithms with a view to increase accuracy in the prediction of churn. Continuous learning is drawn from customer data which leads to refinement of predictions along with better anticipation of churn.
Future Trends in AI Agents in Churn Prediction
The future of customer churn prediction is poised to be transformed by several cutting-edge technologies that will enhance predictive accuracy, personalization, and system efficiency. These emerging innovations are set to push the boundaries of current multi-agent systems, enabling deeper insights and faster responses to customer behavior. Here are the key future technologies that will likely transform churn prediction:
-
Quantum Computing: Quantum computing promises to revolutionize data analysis and prediction by exponentially increasing computational power. For churn prediction, quantum algorithms could solve complex optimization problems that are currently intractable for classical computers.
-
Synthetic Data Generation: Synthetic data refers to artificially generated data that mimics real customer data but without privacy concerns. This technology can dramatically expand the datasets used for training churn prediction models, enhancing model accuracy, especially in privacy-sensitive industries like finance or healthcare.
-
Edge AI: Edge AI refers to the deployment of AI algorithms directly on devices (such as smartphones or IoT devices) rather than relying on centralized cloud systems. For churn prediction, edge AI could allow real-time analysis of customer behavior at the point of interaction, enabling faster and more personalized interventions.
-
Digital Twins: A Digital Twin is a virtual replica of a customer or system that can be used to simulate scenarios and predict outcomes. For churn prediction, digital twins could model customer behavior in virtual environments to test different retention strategies before applying them in the real world.
-
Neuro-Symbolic AI: Neuro-Symbolic AI combines traditional symbolic AI (which uses logical reasoning and rules) with neural networks that learn from data. This hybrid approach could improve the interpretability and reasoning capabilities of churn prediction models.
Conclusion: AI Agents for Churn Predictions
With real-time data, machine learning, and deep analytics, AI agents revolutionize the way banks are approaching customer churn predictions. Moreover, multi-agent AI systems allow them to enhance the accuracy in churn prediction, tailor retention strategies, and eventually adopt a better customer satisfaction approach away from the traditional churn models. The customer-bank relationship is likely to be way more sophisticated as businesses strive to get outcomes in the future with relationships of the customers much more advanced.
Explore More