Key Insights
-
Intelligent content recommendations based on behaviour, context, and preferences improve relevance and engagement.
-
Specialized AI agents work together to analyze data, predict needs, and refine suggestions dynamically.
-
Advancements in predictive analytics, sentiment analysis, and federated learning ensure smarter recommendations while maintaining data security.
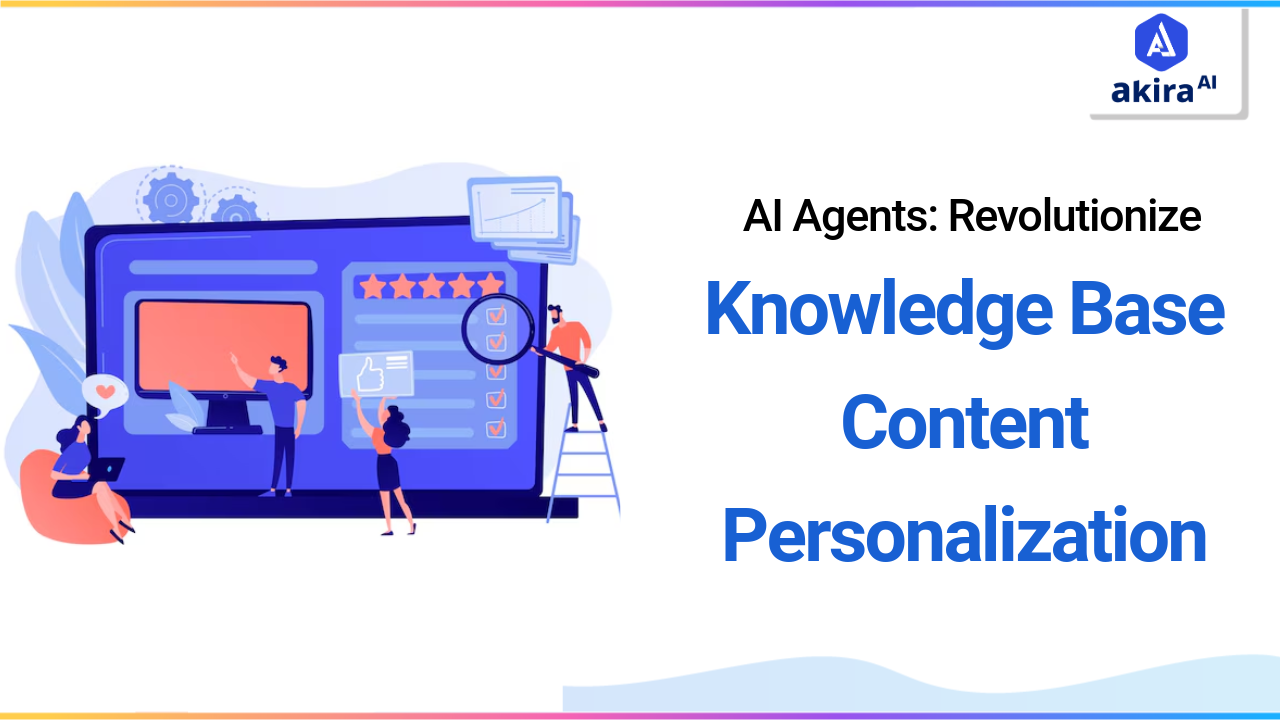
Users increasingly demand personalized and relevant content, making traditional knowledge bases insufficient in meeting these expectations. Static searches and predefined categories no longer provide the level of customization needed. Enter AI agents—these intelligent systems are transforming knowledge bases by offering proactive, context-aware content recommendations tailored to individual user needs. By analyzing user behaviour, preferences, and interactions, AI Teammates ensure that users receive highly relevant content with ease.
In this blog, we’ll explore how AI agents are revolutionizing content personalization, improving user engagement, and enhancing the overall knowledge base experience.
What is a Knowledge Base Content Personalization?
Knowledge Base Content Personalization is the practice of customizing the content within a knowledge base to align with the specific needs, preferences, and behaviours of individual users. The knowledge base can deliver tailored information most relevant to each user’s context by analysing user interactions, such as past searches and content viewed. This approach ensures that users receive content that addresses their unique challenges, helping them find the right solutions more efficiently.
Personalized content is presented dynamically, adapting based on the user’s role, interests, and stage in their journey. The goal is to enhance the user experience by making the knowledge base more intuitive and relevant, allowing users to access the information they need quickly and easily. This method boosts engagement, reduces time spent searching, and improves the overall effectiveness of the knowledge base as a resource.
Key Concepts of Content Personalization
User Profiling: Understanding user preferences, behaviors, and past interactions helps tailor content to individual needs. This involves analyzing demographics, browsing history, and engagement patterns to create personalized experiences.
Context Awareness: Content recommendations adjust based on factors like location, device type, time of access, and browsing habits. This ensures that users receive relevant information at the right moment and in the right format.
Behavioral Analytics: Tracking user interactions, such as clicks, reading time, and search history, refines future recommendations. This data-driven approach ensures that content suggestions align with evolving user interests.
Predictive Personalization: AI-driven systems anticipate user needs by analyzing patterns and suggesting content before users actively search. This proactive approach enhances engagement and reduces the effort required to find relevant content.
Privacy-Centric Personalization: Personalization is balanced with data security, ensuring ethical use of user information. Implementing anonymization, consent-based tracking, and federated learning helps maintain trust while delivering relevant recommendations.
Traditional Way of Utilizing Knowledge Base for Content Suggestion
Traditional Way of Utilizing a Knowledge Base for Content Suggestion refers to the conventional approach where users manually search for information or browse through predefined categories within a knowledge base. This method uses static indexing, keyword-based search functions, and hierarchical structures to organize and retrieve content.
-
Manual Search – Users enter specific keywords to find relevant articles or documents, often requiring multiple attempts to locate precise information.
-
Predefined Categories – Content is structured into fixed sections or folders, requiring users to navigate through menus or topic-based lists.
-
Static Recommendations – Suggestions are based on generic or frequently accessed content rather than personalized user preferences.
-
Limited Context Awareness – The system does not adapt to user behaviour, roles, or past interactions, making content discovery less intuitive.
While this approach provides a structured way to store and retrieve information, it lacks adaptability and proactive content delivery, often requiring more effort from users to find relevant content.
Impact on Customers Due to Traditional Methods
-
Generic Recommendations: Users received one-size-fits-all suggestions, often mismatched to their interests. For instance, recommending popular content to a niche audience would fail to engage them meaningfully.
-
Lack of Context Awareness: The absence of situational understanding led to irrelevant suggestions, such as recommending late-night recipes in the morning or irrelevant product suggestions during off-hours.
-
Slow Adaptation: The rigid, manual update system made it difficult to respond quickly to shifts in user preferences or emerging trends, leading to outdated or irrelevant content being suggested.
-
Reactive Nature: These systems only reacted when users actively searched, missing the opportunity to proactively engage users with helpful or interesting content based on their behaviour or activity.
-
Limited Personalization: Without the ability to truly understand individual preferences, recommendations often felt impersonal, leading to frustration or disengagement.
Akira AI: Multi-Agent in Action
A multi-agent system enhances knowledge-based content recommendations by analyzing user interactions, preferences, and behaviours. Each agent is crucial in ensuring users receive relevant and personalized content.
-
Context Extraction Agent: This agent gathers and analyzes user interactions, such as browsing history, search queries, and access time, to identify behavioural patterns. Understanding when and how users engage with content establishes the context for making relevant recommendations. For example, if a user frequently reads articles about healthcare trends in the morning, this agent notes the preference for future suggestions.
-
Preference Analysis Agent: This agent examines user behaviour to create an evolving dynamic profile. It identifies content preferences, such as whether a user prefers in-depth guides over quick summaries or video content over text. Continuously analyzing interactions refines the system's understanding of user needs, allowing it to adapt and provide better recommendations.
-
Content Matching Agent: Once user preferences and context are identified, this agent searches the knowledge base to find content that aligns with them. It matches keywords, topics, and themes to ensure users receive suggestions directly relevant to their interests. If users frequently engage with AI-related content, this agent ensures they receive the latest research or articles on AI advancements.
-
Recommendation Ranking Agent: After gathering potential content suggestions, this agent ranks them based on relevance and priority. It considers factors such as recency, user engagement trends, and content quality to determine which pieces should be displayed first. This ensures that users see the most valuable and up-to-date recommendations at the top of their list.
-
Personalization Filtering Agent: In the final stage, this agent fine-tunes the recommendations by removing irrelevant or redundant content. It ensures that only the most personalized and high-quality suggestions reach the user. If a user has already read multiple beginner-level articles, this agent filters out similar content and suggests more advanced topics to enhance learning and engagement.
Together, these agents create a seamless and proactive recommendation system that enhances user experience and makes content discovery more efficient and personalized.
Prominent Technologies Driving AI-Powered Suggestions
AI-powered content suggestions are driven by advanced technologies that enable systems to understand user behaviour and deliver personalized recommendations in real time. These technologies help AI agents analyze data, predict needs, and adapt to individual preferences for a more intuitive user experience.
-
Natural Language Processing (NLP): Enables AI to interpret and understand human language, processing text or speech to deliver contextually relevant suggestions.
-
Machine Learning (ML): AI can learn from user behaviour and data, improving recommendation accuracy over time by identifying patterns and preferences.
-
Semantic Search: Enhances search capabilities by understanding the meaning behind words, not just keywords, providing more relevant results based on user intent.
-
Deep Learning: A subset of ML, deep learning models process complex data, such as images or audio, to offer highly personalized suggestions by recognizing intricate patterns.
-
Predictive Analytics: Uses historical data and user behaviour to forecast future needs or preferences, allowing AI to recommend content proactively before the user asks.
How AI Agents Outperform Traditional Technologies
-
Predictive and Autonomous Recommendations: Systems will proactively suggest content based on real-time intent detection, reducing the need for manual searches.
-
Cross-Platform Personalization: Recommendations will seamlessly adapt across devices and platforms, ensuring a unified experience on mobile, desktop, or smart devices.
-
AI-Augmented Content Creation: AI will not only recommend content but also assist in generating summaries, highlights, and personalized insights tailored to user preferences.
-
Emotion and Sentiment-Based Suggestions: Future systems will analyze user emotions and moods to deliver content that aligns with their current state of mind, enhancing engagement.
-
Federated Learning for Privacy-Focused Personalization: AI models will process user data locally on devices, ensuring personalization without compromising data privacy or security.
Successful Real-World Examples of AI Agents
AI-driven content recommendation systems have revolutionized user experiences, with several companies leveraging this technology to create highly personalized platforms. These companies have seen remarkable success by using AI to analyze user data and predict preferences, boosting engagement, conversion rates, and overall user satisfaction.
-
Netflix: Netflix uses deep learning to analyze user viewing history, ratings, and behaviour, personalizing recommendations to individual tastes. More than 80% of the content watched on Netflix comes from AI-driven suggestions. The platform also optimizes thumbnails and marketing based on AI insights, refining its real-time approach.
-
Spotify: Spotify’s AI-driven features, like Discover Weekly and Daily Mix, curate playlists based on listening habits, genre preferences, and trends from similar users. Over 40 million users engaged with Discover Weekly in its first year, and AI now drives 30% of the time spent on the platform, including podcast recommendations.
-
Amazon: Amazon’s recommendation engine uses machine learning to suggest products based on browsing behaviour, purchase history, and wish lists. This system drives 35% of Amazon’s sales by recommending related or complementary products, such as phone cases and headphones, enhancing the shopping experience.
-
LinkedIn: LinkedIn utilizes AI to recommend professional connections, job postings, and relevant content. By analyzing user profiles, activity, and network engagement, AI suggests valuable connections and career opportunities, helping the platform grow to over 930 million users.
-
Google: Google’s AI tailors search results and recommendations across platforms like Google Search and YouTube. By analyzing user behaviour, Google delivers hyper-relevant results, with YouTube’s recommendation system driving 70% of user engagement on the platform. Personalized search results and video suggestions keep users returning for a seamless content discovery experience.
These companies highlight the power of AI in driving personalized experiences, proving that AI is not just a tool for content recommendations but a game-changer in engagement, satisfaction, and business growth.
Final Thoughts on Knowledge Base
AI agents are revolutionizing content recommendation, offering a level of personalization and accuracy that traditional methods could never achieve. By understanding user needs dynamically and using intelligent systems, these tools create seamless, highly relevant experiences across various platforms. The future of content discovery lies in proactive, AI-driven systems that truly understand what users want when they want it. As these technologies continue to advance, they promise to make content discovery faster, more accurate, and more enjoyable for users worldwide.
Next Steps with AI Agents
Talk to our experts about implementing compound AI system, How Industries and different departments use Agentic Workflows and Decision Intelligence to Become Decision Centric. Utilizes AI to automate and optimize IT support and operations, improving efficiency and responsiveness.