Key Insights
AI teammates are set to surpass traditional technologies in the CPG industry by providing accurate, real-time insights into demand fluctuations. With AI-powered replenishment systems, retailers can ensure optimal inventory levels, improving both customer satisfaction and bottom-line profitability through smarter, faster replenishment cycles.
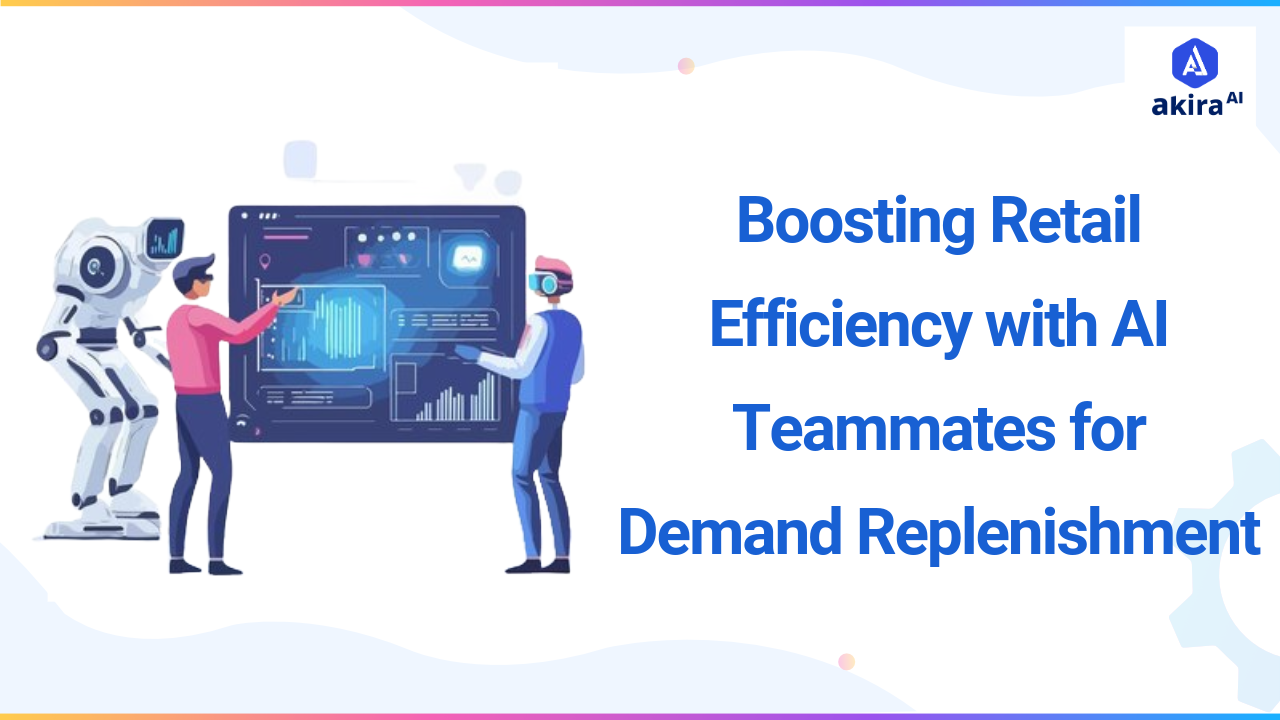
Running a retail or CPG business means constantly balancing demand with supply. This is a rather delicate process that can be overwhelming in case of stockouts or when stocks go overboard. Luckily, AI teammates have entered the scene to ease this burden. Using demand forecasting based on artificial intelligence, the AI agents then provide accurate demand prediction based on current trends, and thus, inventory is not exhausted.
In this blog, we’ll explore how these AI-driven solutions are improving the replenishment process for retailers and CPG companies, leading to better customer experiences and greater operational efficiency.
What is Demand-Based Replenishment?
Demand-based replenishment refers to the process of restocking products based on real-time demand signals rather than on historical sales data alone. In the context of the retail industry and the consumer-packaged goods (CPG) industry particularly, this strategy cannot be overemphasized due to the need to have products in stock and, at the same time, avoid high stock-level risks that could lead to stock-out because of overstocking and eventual wastage.
Key Concepts of Demand-Based Replenishment
Several key concepts form the foundation of demand-based replenishment in retail and CPG industries:
AI-powered Demand Forecasting: This refers to the regular practice of using artificial intelligence to forecast future flows of product demand to make correct replenishments. AI can analyze data from various sources, including sales history, market trends, weather patterns, and even social media, to generate accurate demand predictions.
Replenishment and Allocation: The process of restocking products at stores and warehouses based on the forecasted demand. The multi-agent system makes it easier to decide how much stock to allocate to specific areas so that no area is left with no products while other areas may be crowded with products that are not in high demand.
Demand-Driven Inventory Management: This concept centers on adjusting inventory levels based on real-time demand fluctuations rather than relying on fixed stock quantities. When it comes to inventory, the AI adapts its management by reordering whenever the existing inventory does not match the demand.
Automated Demand Forecasting: AI-powered tools automate the process of demand forecasting, eliminating human error and inefficiency. These tools use machine learning algorithms to continuously refine forecasts, making them more accurate over time.
Smart Replenishment Solutions: These are fully artificial intelligence systems that are used to manage demand forecasting, stock ordering, and stock positioning at different retail outlets. Smart replenishment systems thus increase organizational effectiveness and explosion of stockouts and overstocking.
Traditional Way of Optimizing Demand-Based Replenishment
-
Reliance on Historical Data: Traditional replenishment methods were based on sales data and used the carry-forward method, which, most of the time, did not correctly anticipate future demand. These methods did not capture dynamics in the system, such as changes in demand surge or a promotion; hence, they were inefficient.
-
Manual Intervention: Replenishment processes used to take place automatically, but they needed to be renewed manually on a daily basis, which made the system really slow and full of errors. This cut down the overall efficiency and, most of the time, caused stock issues.
-
Basic Forecasting Models: Basic forecasting models use limited data, often resulting in oversimplified demand predictions. These models were ill-suited for changing market conditions or any sort of shock.
-
Fixed Inventory Levels: Inventory levels were typically fixed, with replenishment occurring on predetermined schedules, regardless of actual demand. This inflexibility resulted in overstocking, which is costly, or stockouts, which are also costly, depending on the inventory level.
-
Lack of Agility: Traditional replenishment methods could not quickly adapt to changes in demand or unforeseen disruptions. This slow response time caused supply chain disruptions and missed sales opportunities.
Impact on Customers Due to Traditional Demand-Based Replenishment Processes
-
Stockouts: Demand forecasting was usually inaccurate; hence, stock-outs were very rampant, which in turn posed a big problem for customers and caused a loss of sales. Retailers faced missed opportunities as customers sought products elsewhere.
-
Excessive Markdown or Product Loss: Overstocking led to markdowns and unsold inventory, especially for perishable goods. This led to losses and customer dissatisfaction because they were able to amass unsold or damaged products on offer at throwaway prices.
-
Poor Customer Experience: Inconsistent product availability led to a poor shopping experience, reducing customer satisfaction. Customers were less likely to return, impacting long-term loyalty and sales.
-
Increased Operational Costs: The inefficiencies of traditional methods lead to increased operational costs by overstock storage and manual inventory control. Such costs reduced profits and prevented any business expansion.
-
Lower Sales Performance: Traditional methods led to poor sales performance, as inaccurate demand forecasting caused lost sales and excess inventory. Retailers struggled to optimize product availability, reducing overall revenue.
-
Tied Up Working Capital: Excess inventory tied up working capital, limiting the ability to invest in more profitable opportunities. This lack of flexibility reduced operational agility and further hindered profitability.
Akira AI: Multi-Agent in Action
Akira AI is an example of a powerful AI-powered replenishment system that utilizes multiple AI agents to streamline demand-based replenishment. The platform features a master orchestrator that integrates various agents to handle different tasks across the supply chain. These agents work together to ensure that replenishment decisions are based on real-time data and demand signals.
Key Agents in Akira AI
-
Master Orchestrator: The Master Orchestrator integrates the functions of all agents, ensuring smooth coordination across the replenishment process. It allows agents to communicate and work together to achieve the best results, adjusting replenishment decisions based on real-time data.
-
Demand Forecasting Agent: The Demand Forecasting Agent uses the best machine learning algorithms to analyze past sales data, the market, and other attributes in order to predict future demand. The agent is continuously updating its estimates for future demand to guarantee that reordering does not become locked into a rigid pattern.
-
Inventory Management Agent: The Inventory Management Agent is responsible for monitoring stocks in several different stores to supply the optimal inventory level and stock the inventory in such a way that it will not be over-stocked or under-stocked.
-
Replenishment Optimization Agent: The Replenishment Optimization Agent determines the optimal quantity of products to be replenished based on demand forecasts and inventory levels. It works in tandem with the inventory management agent to allocate stock efficiently.
-
Supply Chain Agent: The Supply Chain Agent optimizes supply chain operations, ensuring that products are delivered to retail locations on time and in the right quantities. It uses real-time data to adjust logistics plans and minimize disruptions.
Prominent Technologies in Agentic AI-Driven Demand-Based Replenishment
The emergence of AI-powered tools has brought a host of advanced technologies that enable more efficient demand-based replenishment. Some of these technologies include:
-
AI for Store Replenishment: AI systems can track the sales and demand in the stores, which means that replenishment has to be made automatically. These tools can specify which products require a restock and in what measure, such that stores are constantly provisioned with products with the highest customer interest.
-
AI-Powered Demand Forecasting: Machine learning algorithms can analyze vast amounts of data to predict future product demand accurately. These forecasts can be, therefore, updated in real time depending on such factors as weather conditions, promotions, or social media influencers.
-
Retail Inventory Optimization: AI tools can help retailers and CPG companies optimize their inventory levels by recommending adjustments to stock quantities, ensuring products are available without overstocking or understocking.
-
AI in Supply Chain Management: AI technologies are used to optimize various stages of the supply chain, from sourcing materials to distribution. By analyzing real-time data, AI helps ensure that the right products are delivered to the right locations at the right time.
Agentic AI solutions for transforming supply chain management with adaptive systems, real-time insights, and seamless multi-agent collaboration. Click to explore Akira AI’s innovative approach.
Successful Implementations of AI Agents in Retail and Consumer-Packaged Goods
Currently, several companies in retail and CPG have practiced the concept of AI teammates in demand-based replenishments. For instance:
-
Target: Target has adopted AI-driven solutions to enhance its replenishment processes. The retailer uses advanced algorithms to predict demand fluctuations, which helps streamline inventory management. As a result, Target has experienced improved operational efficiency and reduced waste, aligning inventory with real-time customer needs.
-
Danone: Danone has adopted AI-powered demand forecasting models that enable more accurate predictions of customer demand. This implementation has resulted in a 30% reduction in lost sales, demonstrating the effectiveness of AI in optimizing supply chain operations and enhancing product availability.
-
Zara: Zara utilizes AI and generative AI to analyze user feedback and social media trends, which helps the brand adjust its production schedules and inventory levels based on real-time demand forecasts. This approach has improved Zara's product offerings and marketing strategies, aligning them closely with customer preferences.
Future Trends: How AI Agents Supersede Other Technologies
-
Advancement of AI-Powered Tools: AI agents are set to overtake traditional replenishment technologies as they become more sophisticated. With the integration of machine learning and real-time data, AI can provide more accurate demand forecasts, enabling retailers to adjust their stock levels proactively and reduce inefficiencies.
-
Increased Automation in Replenishment Processes: As AI for stock management evolves, there will be greater automation in replenishment cycles. Retailers will reduce the need for manual intervention, increasing efficiency and minimizing human errors that typically occur in traditional methods.
-
Faster Replenishment Cycles: Agentic AI’s ability to analyze vast amounts of data in real time will speed up replenishment cycles. Retailers will be able to restock items faster, ensuring they meet consumer demand promptly and minimize the risks of stockouts or overstocking.
-
Smarter Inventory Management: AI-powered tools will lead to smarter inventory management by dynamically adjusting stock levels based on accurate demand predictions. This capability will help retailers optimize storage, reduce excess inventory, and improve product availability.
-
Reduction of Human Intervention and Errors: As this technology continues to advance, the reliance on human intervention will diminish. Autonomous agents will handle most of the forecasting, replenishment, and stock management processes, significantly reducing the chances of human error and improving overall operational efficiency.
-
Demand-Driven Replenishment as a Core Strategy: With continuous improvements in AI, demand-driven replenishment will become a central component of modern supply chain management. Retailers and CPG companies will increasingly use AI to ensure that their inventory aligns precisely with consumer demand, enhancing profitability and customer satisfaction.
Conclusion: AI Agents for Demand-Based Replenishment
The journey to optimize replenishment doesn’t have to be overwhelming anymore. With the help of AI teammates, retailers and CPG companies can now rely on intelligent tools to manage stock levels and meet customer needs. By adopting AI-powered replenishment systems, businesses are gaining control over their supply chains and offering an improved shopping experience. The result? Happier customers, more efficient operations, and a more profitable future. AI is here to support you—it's time to embrace its full potential.