Key Insights
-
AI agents enhance industrial automation by enabling real-time optimization, predictive maintenance, and reduced downtime, surpassing traditional methods.
-
They improve efficiency and accuracy by analyzing large datasets, predicting failures, and making autonomous adjustments to workflows.
-
Future trends focus on autonomous factories, human-machine collaboration, and sustainable manufacturing to optimize resources and reduce operational costs.
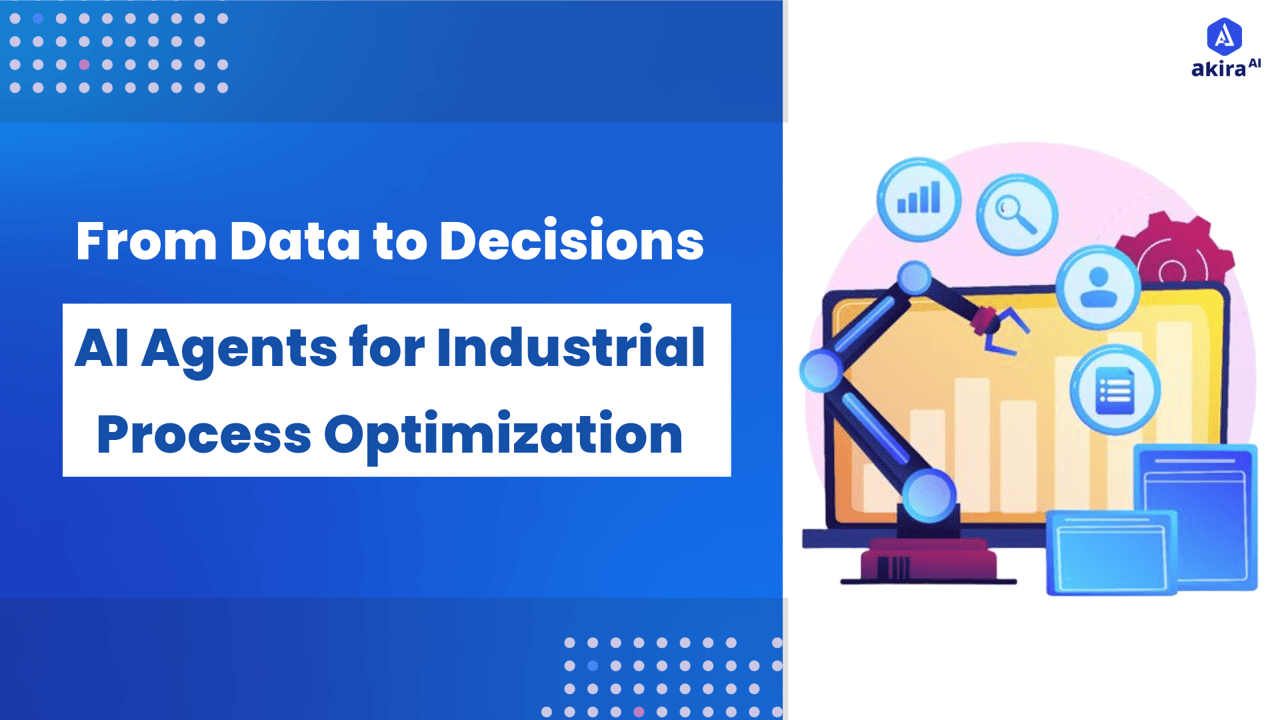
The industrial landscape is rapidly evolving, with automation playing a key role in boosting production efficiency, minimizing errors, and improving overall performance. While Industry 4.0 technologies revolutionized the manufacturing sector by enabling smarter systems, Artificial Intelligence (AI) is now taking automation to the next level.
By integrating AI agents into industrial processes, businesses can optimize operations in real time, reduce downtime, and predict potential failures before they occur. This blog explores the evolution of industrial automation, the role of AI agents, and how they are transforming industries.
What is Industry Process Optimization?
Industrial Process Optimization is the systematic approach to improving efficiency, productivity, and quality in industrial operations by refining processes, reducing waste, and maximizing resource utilization. The goal is to enhance performance, lower costs, and ensure consistent output while maintaining safety and sustainability.
Optimization involves analyzing production workflows, identifying inefficiencies, and implementing improvements to streamline operations. This can include better process planning, minimizing material and energy consumption, reducing downtime, and improving product quality.
Key Concepts of Industrial Process Optimization
To better understand how AI agents fit into industrial automation, it's essential to grasp a few key concepts:
Fixed vs. Flexible Automation: Fixed automation is designed for high-volume production with repetitive tasks, while flexible automation allows quick reconfiguration for different products.
Cyber-Physical Systems (CPS): Integration of digital and physical components enables smart manufacturing, where automated systems interact with the physical world in real time.
Digital Twin Technology: Virtual replicas of physical systems help simulate, predict, and optimize industrial processes before implementing changes in real operations.
Autonomous Decision-Making: Advanced automation systems use data-driven algorithms to make decisions, reducing the need for constant human supervision.
Interoperability & System Integration: Seamless communication between different automation components ensures smooth operation and enhances production efficiency.
Traditional Way of Industrial Process Optimization
Before the rise of AI, industrial automation systems relied heavily on Programmable Logic Controllers (PLCs), Supervisory Control and Data Acquisition (SCADA) systems, and Human-Machine Interfaces (HMIs). These traditional systems were adequate for basic control tasks but had limitations:
-
Manual Input and Limited Decision-Making: While PLCs could automate many tasks, they required significant human input for troubleshooting and optimization. Decision-making was often reactive, and predictive capabilities were minimal.
-
Inability to Adapt to Changing Conditions: Traditional systems struggled to adjust in real-time to fluctuations in production rates, power usage, or material shortages. AI agents, in contrast, can adapt continuously and automatically to these variables.
-
Reactive Maintenance: In conventional systems, maintenance schedules were based on fixed intervals, leading to premature replacements or unexpected breakdowns. AI agents shift this paradigm to predictive maintenance, where equipment issues are identified before they lead to failure.
Impact on Customers Due to Traditional Way of Industrial Automation
While traditional automation systems brought many benefits, they often led to operational inefficiencies, affecting both businesses and customers:
-
High Downtime: Traditional systems that relied on reactive maintenance led to longer unplanned downtimes, which could severely affect production timelines and customer orders.
-
Suboptimal Efficiency: Manual intervention and lack of real-time data analysis led to inefficiencies in production, where machines and workers were not operating at their peak efficiency.
-
Increased Costs: Traditional systems became costly, requiring ongoing repairs and frequent updates to handle modern demands.
Customers experienced delays, poor-quality products, and increased costs as manufacturers struggled to optimize their processes.
Akira AI: Multi-Agent in Action
AI agents play a crucial role in analyzing data at multiple levels of the industrial process, leading to optimization at every stage. Here’s how this works:
Fig1: Architecture Diagram of Industrial Process Optimization
-
Data Collection & Ingestion: The system gathers data from sensors, historical records, external APIs, and user inputs. This raw data provides insights into current and past industrial performance. It is then sent to the Master Orchestrator Agent for further processing.
-
Task Delegation by Master Orchestrator: The Master Orchestrator Agent coordinates the workflow by assigning tasks to specialized agents. Each agent has a specific role, such as data processing, optimization, monitoring, or reporting. This ensures efficient handling of all incoming data and operations.
-
Data Processing & Optimization: The Data Processing Agent cleans, filters, and structures the collected data to remove inconsistencies. The Optimization Agent then analyzes this refined data to detect inefficiencies and suggest process improvements. This step ensures smooth and optimized industrial operations.
-
Real-time Monitoring & Reporting: The Monitoring Agent continuously tracks real-time data, identifying anomalies and deviations in the process. Simultaneously, the Reporting Agent compiles structured reports summarizing key performance metrics. These reports help assess system performance and make necessary adjustments.
-
Decision-making & Dashboard Output: The Master Orchestrator aggregates all processed data and insights from various agents. A final output dashboard presents this information visually for operators and decision-makers. This enables data-driven decisions, continuous improvements, and proactive issue resolution.
Prominent Technologies in the Space of Industrial Automation
Several technologies play a key role in modern industrial automation, enabling the seamless integration of AI agents:
-
Advanced Process Control (APC): APC systems use mathematical models and real-time data to optimize industrial processes by adjusting variables like temperature, pressure, and flow rates. This technology improves production efficiency, maintains product quality, and reduces energy consumption by making continuous adjustments based on live process data.
-
Digital Twin Technology: Digital twins are virtual models of physical industrial processes that allow companies to simulate different scenarios and predict outcomes before making real-world changes. By analyzing historical and real-time data, digital twins help manufacturers identify inefficiencies, test optimizations, and improve decision-making without disrupting production.
-
Industrial Internet of Things (IIoT): IIoT connects sensors, machinery, and software across a manufacturing environment, enabling seamless data collection and communication. This connectivity allows real-time monitoring, predictive maintenance, and automated adjustments, reducing downtime and optimizing resource utilization in industrial operations.
-
Edge & Cloud Computing: Edge computing processes data near where it is generated, reducing latency and enabling faster decision-making on the production floor. Cloud computing complements this by providing large-scale data storage and analysis, allowing industries to gain deeper insights into performance trends and process optimization across multiple facilities.
-
Agentic & Automation Systems: Agentic process automation (APA) and industrial robots streamline operations by performing repetitive tasks precisely and quickly. These systems help optimize production lines, reduce human errors, and enhance safety by automating dangerous or labour-intensive tasks, improving overall process efficiency.
How AI Agents Supersede Other Technologies
AI agents outshine traditional automation technologies in several key areas:
-
Autonomous Factories: Fully automated production lines will use intelligent systems to handle manufacturing processes with minimal human intervention. This will increase efficiency, reduce labour costs, and ensure consistent output quality.
-
Edge Computing in Industrial Systems: Industries can achieve faster response times by processing data locally on devices instead of relying on centralized servers. This reduces latency, enhances real-time decision-making, and improves system reliability.
-
Human-Machine Collaboration: Automation will take over repetitive and physically demanding tasks, allowing workers to focus on strategic planning and creative problem-solving. This will improve workplace safety and enhance overall productivity.
-
Sustainable Manufacturing: Smart optimization techniques will minimize energy consumption, reduce raw material waste, and improve resource utilization. This approach supports environmentally friendly production while lowering operational costs.
-
Dynamic Process Optimization: Advanced automation systems will continuously analyze and adjust workflows in real-time to enhance efficiency. This ensures better scalability, reduces downtime, and optimizes resource allocation.
-
Real-time decision-making: They instantly process large volumes of data, enabling quicker and more efficient decision-making, reducing delays, and optimizing resource allocation.
Successful Implementations of AI Agents
AI agents have been successfully implemented in various industries to enhance productivity and reduce costs. Here are a few notable examples:
-
Predictive Maintenance – General Electric (GE): GE uses AI-powered Predix to analyze sensor data from industrial equipment, predicting failures before they happen. This has reduced unexpected downtime in power plants, aviation, and manufacturing, saving millions in maintenance costs.
-
Quality Control—BMW: BMW employs AI-driven computer vision systems to detect defects in real-time in its manufacturing plants. These AI agents inspect car components for inconsistencies, improving product quality and reducing production errors.
-
Supply Chain Optimization – Amazon: Amazon's AI-powered robots in fulfilment centres optimize warehouse operations by managing inventory, picking and packing products, and reducing order processing time, significantly improving logistics efficiency.
-
Energy Optimization – Google DeepMind & Data Centers: Google uses DeepMind AI to optimize energy consumption in its data centres by adjusting cooling systems based on AI predictions. This has led to a 40% reduction in energy costs for cooling operations.
-
Autonomous Production Lines –Tesla: AI-driven robotic assembly lines automate complex vehicle manufacturing tasks like welding and part placement. AI agents ensure high precision, improving production speed and efficiency while reducing human errors.
Final Thoughts on Industrial Automation
Industrial automation is revolutionizing industries' operations by increasing efficiency, reducing costs, and improving overall productivity. Automated systems streamline processes, minimize errors, and enhance consistency in production. By handling repetitive and labour-intensive tasks, automation allows workers to focus on strategic decision-making and problem-solving. It also improves workplace safety and ensures higher quality standards.
As industries evolve, embracing automation will be essential for maintaining competitiveness and meeting growing demands. However, successful adoption requires careful planning, workforce adaptation, and continuous improvements to maximize long-term benefits and drive sustainable growth in an increasingly dynamic industrial environment.
Next Steps with Industrial Automation
Talk to our experts about implementing compound AI system, How Industries and different departments use Agentic Workflows and Decision Intelligence to Become Decision Centric. Utilizes AI to automate and optimize IT support and operations, improving efficiency and responsiveness.