Key Insights
-
Advanced predictive modelling helps insurers identify at-risk customers early, enabling proactive retention strategies and reducing cancellations.
-
AI agents continuously analyze customer behavior, offering personalized interventions that boost customer satisfaction and improve long-term loyalty.
-
AI-driven systems allow insurers to process vast amounts of data efficiently, reducing operational costs while improving prediction accuracy and customer engagement.
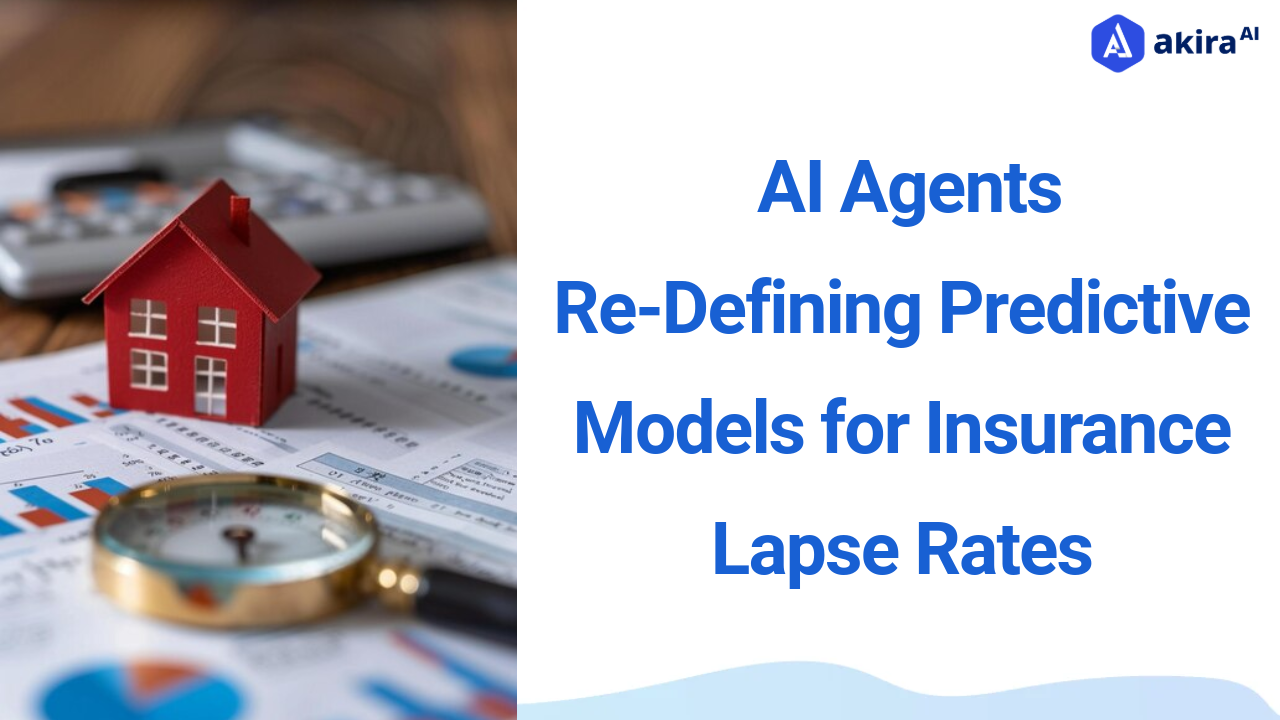
You’re an insurance company working tirelessly to provide customers with the best coverage and service. Yet, despite your best efforts, you notice a troubling trend: more and more policyholders are choosing to walk away, leaving you with a growing list of cancellations. The problem? You're always a step behind, reacting too late to prevent those policy lapses.
But what if you could predict these cancellations before they even happen? What if you had the power to engage at-risk customers with personalized, timely interventions—ensuring they stay loyal and satisfied with your service?
That’s precisely what happened when one leading insurer embraced predictive modelling. By diving deep into customer behaviour and leveraging real-time insights, they turned the tide on policy lapses, reducing cancellations and boosting retention rates. In this blog, we’ll explore how predictive modelling is revolutionizing the insurance industry and how AI Agents are helping companies stay ahead of the curve.
What is Predictive Modelling?
Predictive modelling for lapse rates in insurance is a data-driven approach used by insurers to forecast the likelihood of policyholders cancelling or lapsing their insurance policies. Insurers can predict which customers risk discontinuing their policies by analyzing historical data, such as payment history, policy tenure, customer demographics, and external factors. The model helps insurers proactively identify these high-risk individuals and take action to retain them, such as offering discounts or personalized reminders.
Key Concepts of Predictive Modelling for Lapse Rates
Predictive modeling for lapse rates involves analyzing a variety of factors that influence customer behavior. Here are the primary concepts:
Behavioral Analysis: Insurers study how customers interact with their policies. This includes payment timing, the frequency of customer service interactions, and any recent policy changes. By identifying patterns, insurers can detect early signs of disengagement or dissatisfaction.
Risk Factor Identification: This step examines external factors contributing to policy lapses, such as socioeconomic indicators (e.g., job loss or financial stress), life events (e.g., marriage or relocation), and broader market trends that may impact customers’ ability to maintain their policies.
Temporal Pattern Recognition: Predictive models also analyze time-based trends, such as seasonal variations in policy cancellations or patterns tied to specific policy durations. For example, customers may be more likely to lapse after the first year of coverage.
Traditional Methods of Predictive Modeling for Lapse Rates
Traditional predictive modelling for lapse rates in the insurance industry relies on basic statistical techniques and manual data analysis. These methods primarily use historical data to make predictions about policy cancellations. Insurers typically apply simple regression models that consider a limited set of variables, such as:
-
Payment History – Whether a customer has missed payments in the past.
-
Policy Tenure – How long has the policyholder been with the company?
The analysis is often conducted manually through periodic (monthly or quarterly) reports. This means companies react to policy cancellations only after they happen rather than proactively identifying and addressing risks.
Challenges of Traditional Methods
-
Limited Scope of Analysis – Traditional models rely on just a few variables, overlooking more complex factors influencing lapse rates, such as customer engagement, life events, or economic conditions. This leads to incomplete insights.
-
Delayed Detection – Since reports are analyzed periodically, insurers often recognize policyholder risks too late—after the customer has already cancelled their policy. This reactive approach makes it difficult to intervene in time.
-
Static Models – Traditional models are not designed to adapt to evolving customer behaviour, economic trends, or market fluctuations. As a result, insurers struggle to adjust their strategies when conditions change.
Because of these limitations, insurance companies increasingly turn to advanced predictive analytics techniques, such as machine learning and real-time data monitoring, to improve their ability to anticipate and prevent policy lapses.
Impact on Customers Due to Traditional Methods
The limitations and inefficiencies of traditional predictive modelling methods affect insurers and significantly impact customers. These methods often fail to address individual needs, resulting in poor customer experiences and diminished insurer trust. The key consequences for customers include:
-
Unexpected Policy Cancellations: Customers may cancel their policies without proactive measures due to unresolved concerns or financial strain. Traditional methods typically detect these risks too late for effective intervention, leaving customers unsupported.
-
Generic Retention Strategies: Retention efforts are often generic and lack personalization. Customers receive one-size-fits-all offers or communications that fail to address their unique pain points, making such efforts ineffective and frustrating.
-
Higher Costs for Policyholders: Missed opportunities to prevent lapses result in increased operational and acquisition costs for insurers, often passed down to policyholders through higher premiums or fees.
-
Lack of Personalization: Traditional approaches fail to provide tailored customer solutions, leading to dissatisfaction. Customers may feel that their specific circumstances or needs are not adequately considered, which can erode trust and loyalty.
-
Delayed Problem Resolution: Since traditional methods rely on periodic analysis, insurers often struggle to identify and resolve issues. This delay can cause customers to feel neglected, increasing the likelihood of policy lapses.
Akira AI: Multi-Agents in Action
Fig1: Architecture Diagram of Lapse Rate
-
Data Collection Agent: This agent gathers policyholder data from various sources, such as customer interactions, payment histories, and demographic details. It also monitors external factors, like market conditions, and standardizes the data into a unified format for further analysis.
-
Analysis Agent: Once the data is collected, it is processed to uncover meaningful patterns and trends. It generates insights from historical data and creates feature sets to develop predictive models for more accurate forecasts.
-
Risk Assessment Agent: This agent evaluates the probability of a policy lapsing by calculating risk scores based on the analyzed data. It identifies key risk factors such as irregular payment behaviour or life events and creates a detailed risk profile for each policyholder.
-
Prediction Agent: Using insights from the risk assessment agent, the prediction agent generates forecasts on lapse probabilities and time-based risk projections. It continuously updates these predictions in real-time as new data comes in, ensuring the model’s accuracy.
-
Intervention Agent: The intervention agent designs personalized retention strategies and executes automated communications like reminders or special offers. It also coordinates with human agents for more complex cases, tracking the effectiveness of these strategies to refine future interventions.
Prominent Technologies in Predictive Modeling for Lapse Rates
Technological advancements have significantly improved predictive modelling, enhancing insurers' ability to forecast lapses and improve retention strategies. Key technologies driving these advancements include:
-
Machine Learning Models: Algorithms like Random Forests and Gradient Boosting analyze complex data sets and rapidly identify patterns. These models offer improved accuracy over traditional methods in predicting customer behaviour and lapse risks.
-
Neural Networks: Deep learning models uncover intricate relationships within data, making them ideal for detecting subtle trends and predicting lapses in nonlinear, multidimensional data.
-
Natural Language Processing (NLP): NLP tools analyze unstructured data (e.g., emails, chat logs) to detect sentiment, dissatisfaction, or intent to lapse. This enables proactive engagement and helps improve retention.
-
Big Data Analytics: Big data tools process vast amounts of data from millions of policies and customer interactions, revealing hidden patterns and correlations that enhance risk assessment and prediction accuracy.
-
Cloud Computing: Scalable cloud infrastructure enables real-time data processing, allowing insurers to dynamically update models and respond swiftly to emerging risks or market shifts.
-
Visualization Tools: Interactive visualization tools present modelling results in an intuitive way, helping decision-makers explore trends and make informed choices about retention strategies.
How AI Agents Supersede Other Technologies
AI agents take predictive modelling to the next level by integrating real-time analysis, autonomous decision-making, and dynamic adaptability. Unlike traditional technologies, which rely on static models or periodic updates, AI agents continuously monitor customer behaviour, predict risks, and execute tailored interventions, offering more effective solutions to reduce lapse rates.
-
Real-Time Customer Engagement: Insurers can identify at-risk policyholders in real-time and immediately trigger personalized actions, such as reminders, customized offers, or loyalty incentives, to prevent lapses.
-
Autonomous Retention Strategies: Retention actions, including flexible payment options or premium adjustments, will be autonomously executed, reducing the need for manual intervention and enabling faster response to customer needs.
-
Continuous Adaptation to Customer Behavior: Strategies will dynamically adjust to reflect changing customer behaviour, incorporating new insights from data as it becomes available and responding to shifts in market conditions or personal circumstances.
-
Comprehensive Risk Analysis: A broader range of factors, including economic conditions, life events, and customer sentiment, will be considered to provide more precise insights into lapse risks and inform more effective retention efforts.
-
Scalability and Efficiency: Advanced techniques will allow insurers to process and analyze large amounts of data across vast customer portfolios, improving operational efficiency while keeping costs low and enhancing customer retention.
Successful Implementations of AI Agents
Implementing AI agents in predictive modelling has already transformed the insurance industry, helping insurers improve retention rates and operational efficiency. Below are some real-world examples of how prominent companies have adopted AI to reduce lapse rates:
Prudential Financial
Prudential implemented an AI-driven predictive modelling system to reduce policy lapses in their term life insurance portfolio. The system identified at-risk customers three months earlier by analyzing behavioural data and payment patterns, allowing for proactive engagement with tailored retention strategies.
Key Outcomes
-
Lapse rates were reduced by 35%, improving customer retention.
-
Strengthened customer relationships and loyalty.
-
Saved millions in acquisition costs by retaining existing policyholders.
AXA
AXA used a multi-agent AI system to enhance customer retention by monitoring interactions, market conditions, and payment histories. Combining behavioural analysis with real-time risk assessments enabled highly targeted retention campaigns.
Key Outcomes
-
Retention rates improved by 28%, reducing policy lapses.
-
€15 million in cost savings through efficient interventions.
-
Identified macroeconomic trends to adjust policies and pricing.
MetLife
MetLife adopted an AI agent platform using machine learning to improve lapse prediction accuracy. The system evaluated factors like customer sentiment and payment behaviour, focusing retention efforts on high-risk customers.
Key Outcomes
-
Lapse prediction accuracy increased to 87%.
-
Retention efforts improved by 45%, boosting profitability.
-
Used NLP to detect dissatisfaction and respond proactively.
Next Steps in Insurance
Talk to our experts about implementing Agentic AI-driven solutions in predictive modelling for insurance lapse rates, leveraging Agentic Workflows and Decision Intelligence to optimize retention strategies, enhance customer engagement, and improve overall operational efficiency.