Key Insights
The integration of AI agents in predictive maintenance transforms hospital operations by enhancing equipment reliability and reducing downtime. Through real-time data collection, anomaly detection, and predictive analytics, AI enables proactive interventions, optimizing resource allocation and improving patient safety. The Master Orchestrator Agent ensures seamless coordination among all AI agents, streamlining workflows for maximum efficiency in healthcare technology.
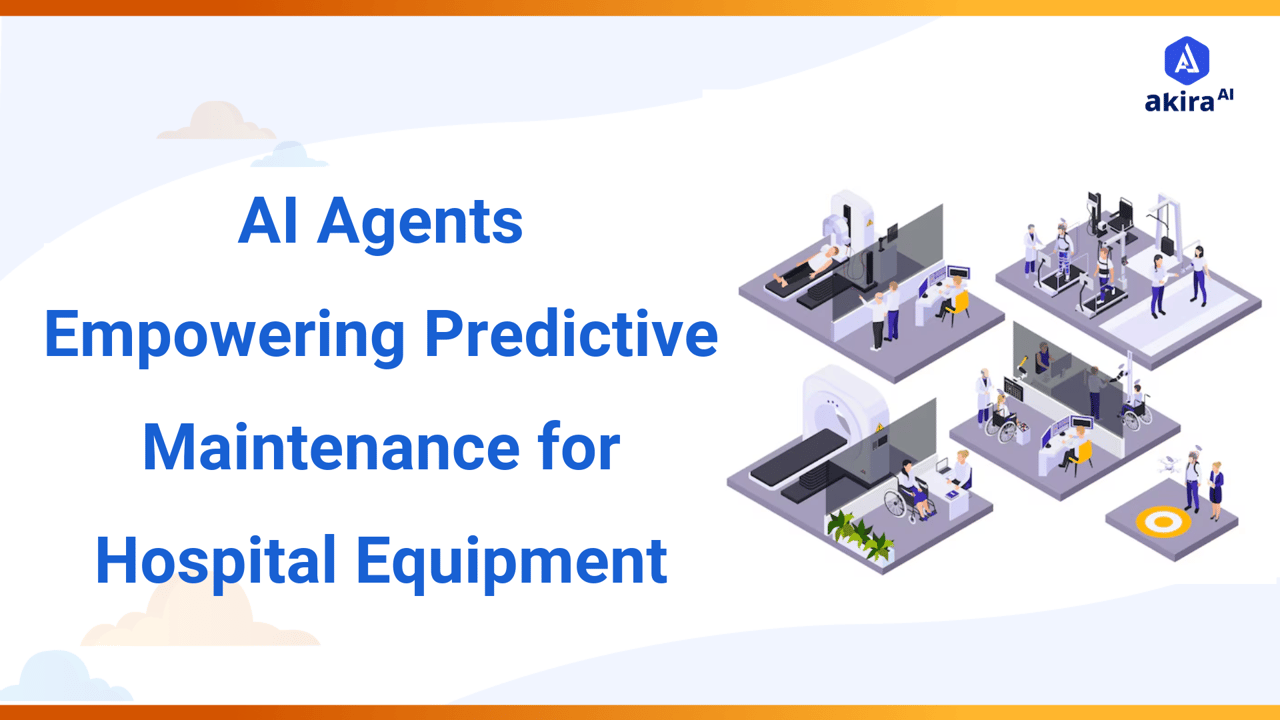
"An ounce of prevention is worth a pound of cure." – Benjamin Franklin.
This adage proves true in the healthcare setting, as new and reused products must be highly reliable, consistent, and safe for each patient. Today, AI agents are reformulating how predictive maintenance is done using data analytics, machine learning, and IoT to predict when equipment will fail. By continuously monitoring device performance, AI can prevent unexpected breakdowns, reduce downtime, and improve the efficiency of hospital operations. With agentic AI in predictive maintenance, hospitals can ensure that their equipment operates at peak performance, ultimately improving patient outcomes and operational costs.
What is Predictive Maintenance for Medical Equipment?
Hospitals rely on the seamless operation of critical medical equipment, such as MRI machines, ventilators, and diagnostic tools, to ensure optimal patient care. Predictive maintenance uses advanced data analytics to anticipate equipment failures before they occur, significantly reducing downtime and enhancing patient outcomes.
In the present world, where AI agents are dominating the market, this process has grown faster with the help of machine learning and predictive algorithms used in the real-time monitoring and maintenance of healthcare devices.
Key Concepts of Predictive Maintenace for Medical Equipment
Data-driven Approach: Predictive maintenance uses technologies such as IoT, machine learning, and AI to forecast equipment failures by analyzing real-time data from medical devices.
Continuous Monitoring: Sensors installed on medical devices (such as temperature, pressure, and vibration sensors) collect data to monitor machine health.
Proactive Maintenance: Predictive maintenance by AI system discovers probable failings in advance and avoids age-related repairs that are time-consuming and costly.
Extended Equipment Lifespan: By addressing issues before they escalate, predictive maintenance extends the life of medical equipment, optimizing healthcare facility resources.
Traditional Way of Predictive Maintenance for Medical Equipment
Traditional predictive maintenance in medical equipment involves regular check-ups and inspections to determine whether faults are likely to occur. It has been applied in many hospitals and healthcare facilities for years, mainly for preventive maintenance (PM) and condition-based maintenance (CBM).
-
Preventive Maintenance (PM) entails routine, periodic equipment maintenance regardless of its need, condition, or status. One ultimate goal is to decrease the chances of breaking down, which is done when parts are changed, or services are done regardless of any sign of trouble.
-
Condition-based maintenance (CBM), on the other hand, is dependent on the state of the equipment through signs such as temperature, vibration, or pressure. This is done only when certain levels are proposed, meaning some problems can occur. This approach depends on time-to-time evaluation, and any problem identified is corrected.
-
Scheduled Inspections and Manual Reporting: Maintenance teams usually perform their inspections and repair activities according to timetables or predetermined usage rates. The techniques used by the technicians are crude; basically, they examine and maintain the equipment by hand.
Challenges in Traditional Predictive Maintenance for Medical Equipment
Traditional methods of predictive maintenance for medical equipment face several challenges:
-
Unpredictable Downtime: Despite maintenance schedules, equipment reliability is still at risk due to unforeseen failures. Downtime can result in interrupted care, affecting patient safety.
-
Resource Waste: Both PM and CBM may result in over- or under-maintaining equipment, wasting hospital resources, and increasing operational costs.
-
Inefficient Use of Data: While IoT (Internet of Things) and sensors are often in place, data generated is underutilized, lacking the necessary analytics to predict failures or optimize predictive maintenance in healthcare systems.
-
Delayed Interventions: Preventive and condition-based maintenance may not detect issues early enough, leading to delays in addressing critical malfunctions, which is detrimental to patient safety.
Impact on Customers due to the Traditional way of Predictive Maintenance
-
Disrupted Hospital Operations: The conventional approaches to carrying out maintenance activities include a calendar-based approach, which can cause equipment to break down at the wrong times and blow different working schedules in the hospital.
-
Increased Risk of Equipment Failures: Reactive maintenance and schedule usually do not identify problems at early stages, and the equipment breakdowns that occur can disrupt important medical operations and endanger patients’ lives.
-
Unoptimized Use of Resources: Manual maintenance scheduling results in wasted resources, as maintenance personnel are dispatched regardless of whether the equipment needs attention. This inefficient allocation of labour and time increases operational costs.
-
Reduced Equipment Lifespan: Traditional methods, particularly PM and CBM, can result in over-servicing or under-servicing medical equipment. Both scenarios can lead to excessive wear or neglected maintenance, shortening the equipment's useful life.
-
Inconsistent Patient Care: Faulty equipment or a lack of repair services often results in repeated or ongoing patient treatment. Sometimes, equipment is not in optimum working order when needed most.
-
Dissatisfaction with Hospital Services: Patients who experience delays or disruptions due to equipment issues may develop dissatisfaction with the hospital's services. This can lead to reduced trust in the healthcare provider and lower patient retention rates.
Discover how Agentic AI transforms chronic disease management by leveraging real-time data and intelligent decision-making to provide personalized, proactive care solutions.
Akira AI: Multi-Agent in Action
In predictive maintenance, multiple AI agents operate together to manage and monitor a hospital's vast array of equipment. Here’s how the process flow generally works:
-
Master Orchestrator: The Master Orchestrator Agent acts as the central control system, coordinating all AI agents within the predictive maintenance ecosystem. This ensures that all agents communicate effectively and, more importantly, that maintenance processes flow efficiently.
-
Data Collection Agent: The Data Collection Agent gathers real-time telemetry from hospital equipment, including usage statistics, temperatures, and error codes, establishing a continuous data stream for monitoring machine health and performance.
-
Anomaly Detection Agent: The Anomaly Detection Agent computes data for any suspicious pattern or shift and immediately makes other departments aware of a possible mechanical breakdown that needs intervention to avoid equipment breakdown.
-
Predictive Analytics Agent: The Predictive Analytics Agent applies historical data and machine learning models to forecast possible equipment breakdowns, enabling proactive maintenance and reducing the likelihood of unexpected disruptions.
-
Alerting and Reporting Agent: The Alerting and Reporting Agent automatically notifies the hospital staff about maintenance requirements while providing detailed documents to support the need for maintenance and eliminate lengthy outage durations.
-
Optimization Agent: The Optimization Agent evaluates equipment usage trends to optimize scheduling and resource allocation, ensuring devices are available when needed and supporting efficient maintenance planning.
Prominent technologies in the space of Predictive Maintenance for Medical Equipment
In recent years, several technologies have emerged to support predictive maintenance for medical equipment:
-
Condition-Based Maintenance (CBM): This approach involves using sensors and predictive algorithms to monitor the real state of equipment and then proceed to maintenance only if necessary.
-
Reliability-Centered Maintenance (RCM): A process used to guarantee that assets can continue to function effectively by taking into account the potential for equipment to fail as well as the implications of this for operations.
-
Data Analytics: Using data to predict trends and potential failure points, allowing for proactive decisions and actions.
-
IoT Sensors: Smart sensors collect real-time data on medical equipment performance, enabling more accurate wear and tear assessments.
How AI Agents Supersede Other Technologies for Predictive Maintenance
-
Real-Time Performance Monitoring: AI agents can monitor equipment performance in real-time using machine learning and data analytics. This allows them to determine when maintenance is needed—even before the equipment starts to operate outside of normal parameters.
-
Smarter Predictive Maintenance: AI in predictive maintenance leverages vast amounts of historical and real-time data, enabling AI systems to predict failures more accurately than traditional methods. These agents can adapt over time by learning from previous patterns, which enhances their predictive capabilities.
-
Resource Optimization: AI agents save resources by scheduling repairs at specific times only, not frequently. This results in some savings and technicians are dispatched only when the equipment needs attention.
-
Enhanced Patient Safety: Autonomous agents combined with real-time monitoring and predictions for required actions allow us to provide the best equipment availability and ensure that all the necessary devices are in proper working order. This leads to increased patient safety mainly because the likelihood of equipment failure during medical procedures is also reduced.
-
Agentic AI and Agentic Workflow: Agentic AI refers to AI systems that can autonomously manage tasks without constant human oversight, while agentic workflows describe the automation of complex maintenance processes. This reinforces the whole maintenance process, eliminating most human errors and increasing productivity.
Successful Implementations of AI Agents in Predictive Maintenance for Medical Equipment
-
MRI Machines: AI agents observe the critical state of the cooling systems and the components and report any problem, like overheating, to make MRI machines available to diagnose patients.
-
Ventilators: Autonomous agents in ICUs track airflow, pressure, and oxygen levels to identify irregularities early, preventing malfunctions that could impact patient care.
-
Laboratory Equipment: The multi-agent system looks over diagnostic equipment such as centrifuges and analyzers to check for changes in calibration or error rates, thus achieving accurate results.
-
Infusion Pumps: AI agents detect irregularities in fluid delivery rates or pressure, preventing potential malfunctions that could lead to critical errors in drug administration.
-
X-ray machines: AI monitors component status and usage data to ensure prolonged component durability by predicting malfunctions during patient diagnosis.
-
Patient Monitoring Systems: AI-powered predictive maintenance ensures that vital sign monitors function correctly, analyzing sensor data to predict potential failures before they occur.
Conclusion: AI Agents for Predictive Maintenance in Healthcare
AI-powered predictive maintenance transforms healthcare by enabling hospitals to proactively manage medical equipment, reducing costly failures and ensuring reliable patient care. By predicting equipment issues before they occur, hospitals can prevent downtime, extend equipment lifespans, and achieve significant cost savings.
With AI automating monitoring and alerts, hospital staff can focus more on patient care, boosting productivity and enhancing service quality. Continuous data analysis from IoT and machine learning improves diagnostic accuracy and ensures equipment performance.
This data-driven approach ensures uninterrupted, high-quality care, making AI-powered predictive maintenance a key driver in shaping the future of healthcare. Life-saving equipment is always ready when needed.
To Get More Insights About Healthcare