Key Insights
Accident and incident prediction and prevention in transportation leverages AI Agents to enhance safety and reduce risk. By analyzing real-time data such as traffic patterns, environmental conditions, and driver behavior, AI Teammates systems can proactively identify and mitigate potential hazards. This approach leads to fewer accidents, improved traffic flow, and reduced operational costs. As AI technology advances, its role in optimizing road safety and efficiency will continue to grow, offering long-term benefits for both transportation systems and the environment.
The increasing prevalence of traffic accidents and incidents presents a major challenge to road safety, especially in urban environments where vehicle numbers are soaring. These accidents not only cause significant fatalities and injuries but also lead to traffic congestion and substantial economic losses. In low-income countries, fatality rates are alarmingly high compared to wealthier regions, highlighting a pressing need for effective intervention strategies.
Through Agentic AI, advanced machine learning models, and predictive algorithms, the transport industry is gaining new tools for accident prediction and prevention. By integrating geospatial data, spatial analysis, and optimization techniques, AI can analyze traffic patterns, predict accident hotspots, and proactively mitigate risks. This blog will explore how AI agents are revolutionizing road safety, reducing congestion, and offering precise, data-driven solutions for enhancing transportation systems' efficiency and minimizing the impact of accidents.
What is Accident and Incident Prediction and Prevention?
Accident and Incident Prediction and Prevention in the transport industry involves the proactive identification of risks to avoid road accidents and other incidents. This process aims to predict and prevent accidents before they occur, reducing the negative impacts on human lives, traffic flow, and overall transportation safety. The focus is on utilizing data from various sources, including traffic patterns, weather conditions, and driver behavior, to anticipate when and where accidents are most likely to happen. By forecasting high-risk scenarios and locations, transportation authorities can implement preventive measures, such as adjusting traffic signals, improving road infrastructure, or introducing public awareness campaigns. Effective accident and incident prediction not only saves lives but also reduces congestion, ensures smoother traffic operations, and contributes to long-term improvements in road safety.
A Brief Overview of Accident and Incident Prediction and Prevention
Accident and Incident Prediction and Prevention is a critical field within the transportation industry that focuses on reducing the occurrence of road accidents and other incidents through proactive measures. It involves analyzing data from various sources such as traffic patterns, environmental conditions, and human factors to predict potential accidents or traffic disruptions. The goal is to identify high-risk locations and scenarios to implement preventive strategies like optimizing traffic management, enhancing road safety infrastructure, and informing drivers about potential hazards.
In addition to human safety, this approach aims to alleviate traffic congestion and reduce economic losses associated with accidents. With the use of advanced analytics, including machine learning and AI-based systems, it is possible to accurately forecast where and when incidents are likely to occur. The integration of spatial and temporal data further enhances the ability to plan and deploy interventions in real-time, ensuring that accidents can be prevented and the flow of traffic remains uninterrupted.
This approach not only saves lives but also contributes to smoother traffic flow, improved transportation systems, and the efficient use of resources.
Traditional vs. Agentic AI Accident and Incident Prediction and Prevention
Aspect |
Traditional Methods |
Agentic AI Methods |
Data Processing |
Slower, often manual or batch processing with limited data points |
Real-time processing of multiple data streams. Process vast data in seconds. |
Predictive Accuracy |
Limited by historical trends. Subject to human error |
High accuracy with real-time analysis and predictive modeling |
Risk Detection |
Reactive; often after an incident occurs |
Proactive; predicts potential risks minutes in advance |
Pattern Recognition |
Based on obvious trends and historical records |
Advanced pattern detection including subtle correlations |
Scalability |
Resource-intensive and challenging to scale |
Easily scalable with cloud-based and automated AI solutions |
Environmental Monitoring |
Limited; reliant on static data |
Dynamic; incorporates real-time weather and traffic data |
Learning Capability |
Limited to documented cases |
Continuous learning from new scenarios |
Akira AI: Multi-Agent in Action
Fig1: Architecture Diagram of Accident and Incident Prevention
The multi-agent system consists of specialized AI agents working together to ensure comprehensive safety coverage. Each agent focuses on specific aspects while sharing information through a central coordination system.
-
Data Collection Agent: This central agent consolidates data from vehicle sensors, environmental monitors, and driver behavior analysis. By gathering comprehensive telemetry, road, and environmental information, it provides a real-time, unified data stream to inform all other agents’ decision-making processes.
-
Risk Assessment Agent: Using data from the Data Collection Agent, this agent evaluates risk in real-time, combining historical patterns with live data on driver actions, vehicle health, and environmental conditions. It proactively forecasts potential incidents and issues alerts for preemptive action to prevent accidents.
-
Route Optimization Agent: Responsible for dynamic route planning, this agent assesses current traffic, weather, and road conditions to create the safest and most efficient travel paths. By continuously adjusting routes in response to real-time data, it enhances safety while minimizing travel delays.
-
Compliance Agent: This agent supports drivers by monitoring compliance with safety protocols (like hours of service and speed limits) and issuing corrective feedback. It detects risky driving behaviors, such as harsh braking or signs of fatigue, and provides in-cab alerts to help maintain safe driving standards.
-
Emergency Coordination Agent: In the event of an incident, this agent coordinates the emergency response by notifying relevant teams, calculating optimal response routes, and managing communication with dispatch. It ensures emergency services arrive promptly and resources are allocated efficiently to minimize incident impact.
-
Alerting Agent: Acting as the network’s communication backbone, this agent distributes alerts and updates to drivers, dispatchers, maintenance teams, and emergency responders. It ensures all parties have access to critical, real-time information for coordinated, effective decision-making.
-
Reporting Agent: Post-incident, this agent reviews data to understand root causes and generates actionable insights. Its analysis feeds back into the system, continuously improving prediction models and optimizing future safety strategies.
Use-cases of Accident and Incident Prediction and Prevention
-
Fleet Management: AI optimizes route planning by assessing risks like traffic and weather, ensuring safer and more efficient routes. Real-time vehicle health monitoring tracks critical vehicle data, helping prevent accidents caused by mechanical failure. Dynamic scheduling adjusts delivery plans based on factors like driver fatigue and weather, prioritizing safety alongside efficiency.
-
Driver Safety Systems: AI-powered fatigue detection systems monitor driver behavior to detect signs of drowsiness and trigger alerts. Behavior analysis identifies risky driving patterns, like harsh braking or speeding, to provide feedback and improve safety. Personalized training tailors safety programs based on individual driving habits, helping drivers enhance their skills.
-
Infrastructure Monitoring: AI helps with road condition assessments by identifying hazards like potholes and road damage, enabling timely repairs. Traffic pattern analysis uses real-time data to predict congestion and improve route planning. Weather impact prediction informs drivers of weather-related hazards, allowing them to adjust speeds or routes for safety.
-
Emergency Response: Automated incident detection quickly identifies accidents, alerting emergency teams for a faster response. Optimal response route calculation guides emergency services to accident sites via the fastest, safest routes. Resource allocation ensures emergency resources are dispatched efficiently, prioritizing critical incidents.
Operational Benefits of Accident and Incident Prediction and Prevention
-
Improved Safety: Implementing predictive systems can reduce accidents by up to 30-40%, resulting in a 50-60% decrease in accident-related costs (medical expenses, insurance claims, vehicle repairs). This translates to significant savings, as well as improved driver and passenger safety.
-
Reduced Traffic Congestion: By minimizing accidents and predicting traffic patterns, the flow of traffic can be optimized, cutting down 15-20% of congestion-related delays and reducing fuel consumption by up to 10-15%. This translates to improved logistics and lower operational costs for freight and public transport.
-
Lower Operational Costs: A predictive approach can save 20-30% on operational costs by reducing accidents that typically result in increased insurance premiums, vehicle maintenance, and emergency response fees. Companies can save money by lowering insurance premiums by as much as 25-35% annually.
-
Increased Efficiency: Transportation systems with predictive models can see up to a 25-35% increase in operational efficiency, improving traffic management, reducing emergency response times, and decreasing idle times for vehicles. This leads to higher resource utilization and savings in infrastructure costs.
-
Enhanced Decision-Making: By leveraging predictive insights for infrastructure planning, transportation systems can reduce costs associated with unnecessary road repairs or redesigns by up to 20-25%. Long-term maintenance costs drop by up to 15% when incidents are minimized and traffic patterns are better managed.
-
Environmental Benefits: With fewer accidents and reduced congestion, fuel consumption decreases, saving up to 10-15% in fuel costs. This leads to environmental ROI through reduced carbon emissions and potential savings in fuel taxes or penalties, contributing to sustainability goals.
Technologies Transforming Accident and Incident Prediction and Prevention
-
Neural Networks for Pattern Recognition: Neural networks process complex data to detect patterns in accident trends, driver behavior, and vehicle performance, supporting predictive insights for safer decision-making.
-
Random Forests for Risk Assessment: Random forests analyze multiple data points to assess risk levels, identifying high-risk scenarios and enabling targeted preventive actions to reduce potential incidents.
-
Support Vector Machines for Classification: Support vector machines classify data like risky vs. safe driving, helping AI systems make real-time decisions to improve safety.
-
Computer Vision: Computer vision analyzes visual data from cameras, supporting applications like object detection, driver behavior analysis, and road condition monitoring for safer operations.
-
Natural Language Processing (NLP): NLP processes communication data, supporting automatic report generation, alert management, and monitoring of driver interactions for safety.
-
IoT Sensor Integration: Sensors gather real-time data on vehicle performance, environmental conditions, and traffic, helping AI systems make accurate safety predictions and adjustments.
Future Trends of AIPP
The future of Accident and Incident Prediction and Prevention lies in even more sophisticated AI agent integration. We can expect:
-
Advanced Integration: The future of AIPP will see seamless communication across various transport modes, enabling integrated systems that share critical data in real-time. This coordination will enhance the accuracy of predictions and improve overall safety by proactively addressing risks across the entire transport network.
-
Enhanced Real-Time Coordination: AI agents will facilitate better coordination between vehicles, drivers, and traffic management systems, providing instant updates on risks, traffic conditions, and necessary adjustments to ensure continuous safety and smooth operations.
-
Improved Prediction Accuracy: With ongoing advancements in AI algorithms, prediction accuracy will improve, allowing for better forecasting of accidents and incidents, including more precise risk assessments for various factors such as driver behavior, road conditions, and environmental factors.
-
Technology Evolution: The integration of emerging technologies like quantum computing will enable faster, more complex data processing, while enhanced sensor technologies will provide more detailed, real-time information to AI systems, boosting their predictive and analytical capabilities.
-
Advanced Sensor Technologies: Next-generation sensors will provide more detailed environmental and vehicle data, including higher precision in detecting road conditions, vehicle health, and driver behavior, helping AI systems make more accurate predictions and faster decisions.
Conclusion: AI Agents for AAIP
The transportation industry is at a turning point, with AI agents revolutionizing safety by making proactive accident prediction and prevention a reality. These systems reduce accidents, improve efficiency, and drive cost savings, offering a competitive edge to forward-thinking organizations. As technology evolves, AI agents will only become more powerful, pushing the boundaries of transport safety. Investing in AI-powered safety systems is not just about compliance—it's about leading innovation and protecting lives and assets on every journey. The future of transport safety is here, and it’s powered by AI.
Discover How AI Agents Can Enhance Road Safety
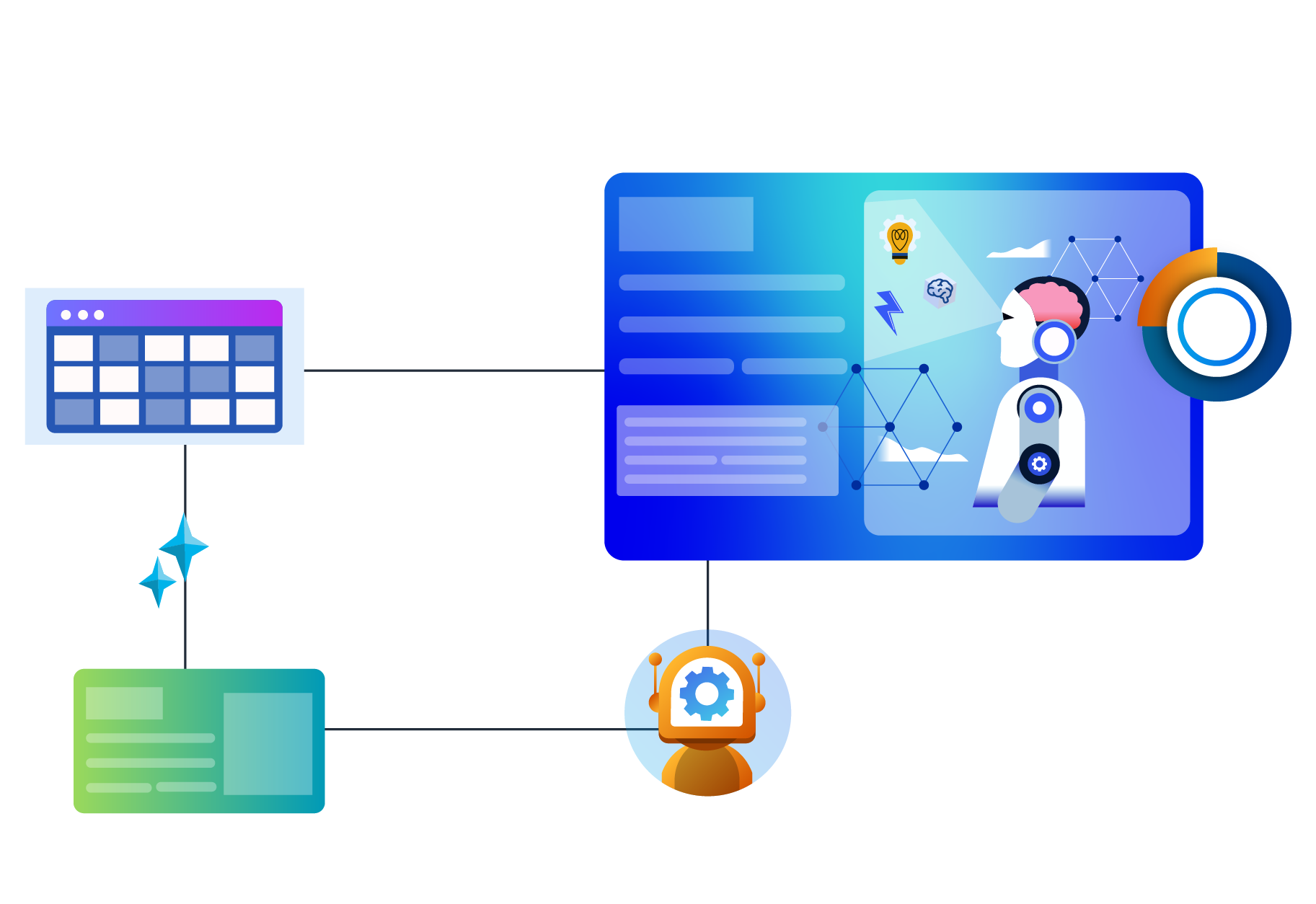