Key Insights
AI agents enhance real-time traffic monitoring by optimizing traffic flow, predicting congestion, and automating signal adjustments. They proactively address issues like accidents and roadblocks, ensuring faster response times. The integration of AI improves road safety, reduces delays, and supports efficient urban mobility. This technology contributes to smarter, more sustainable traffic management systems.
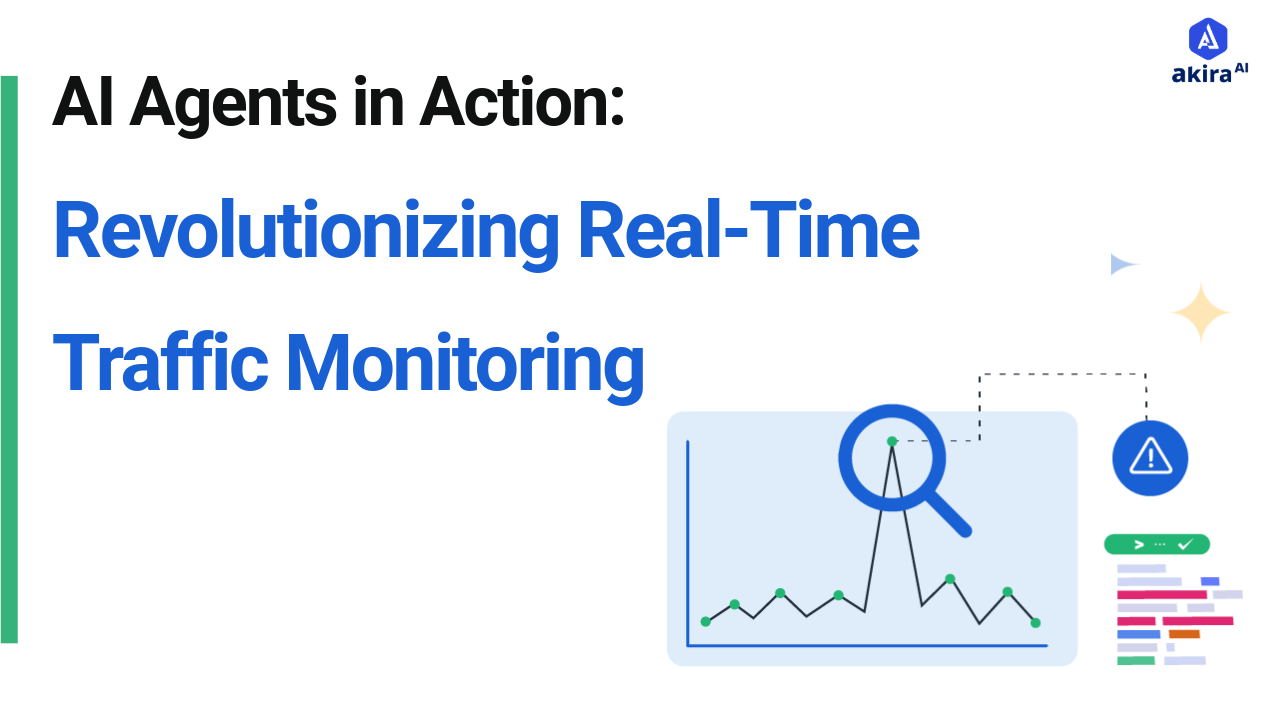
Traffic congestion in urban areas is a growing challenge, causing delays, fuel wastage, and safety concerns. Traditional traffic management methods often fall short of addressing the complexity of modern traffic flows. To tackle this, real-time traffic monitoring and issue resolution with AI agents are emerging as innovative solutions. By utilizing technologies like image processing, vehicle detection, and machine learning, AI agents can monitor traffic conditions, analyze congestion patterns, and dynamically adjust signal timings. These intelligent systems not only optimize traffic flow but also enhance safety by prioritizing emergency vehicles and preventing accidents.
This blog explores how AI agents are transforming transportation by providing real-time traffic updates, automating signal control, and ensuring more efficient, safer roads for everyone.
What is Real-Time Traffic Monitoring and Issue Resolution?
Real-Time Traffic Monitoring and Issue Resolution involves continuously tracking and managing traffic conditions to optimize the flow of vehicles and reduce congestion. This approach gathers live data on traffic patterns, road usage, accidents, and delays to detect issues as they arise. By analyzing this real-time information, traffic systems can make dynamic adjustments, such as altering traffic signal timings, rerouting vehicles, and giving priority to emergency vehicles. The goal is to proactively address disruptions, improve traffic efficiency, reduce waiting times, and enhance road safety, ultimately ensuring smoother transportation for all road users.
A Brief Overview of Real-Time Traffic Monitoring
Real-time traffic monitoring, enhanced by AI agents, revolutionizes how cities manage traffic flow and address congestion. It uses live data collected from various sources like cameras, GPS, and sensors to monitor traffic conditions, detect anomalies, and predict potential issues. These intelligent systems analyze vast amounts of data to identify traffic patterns, congestion hotspots, and accidents. By processing this information in real-time, AI agents can make dynamic decisions, such as adjusting traffic signal timings or rerouting vehicles, ensuring smooth and efficient traffic management.
Agentic AI also plays a crucial role in proactive issue resolution. For example, they can prioritize emergency vehicles, reduce delays by optimizing routes, and even predict traffic disruptions caused by accidents or road closures. By continuously learning from data and refining their algorithms, improve their accuracy and efficiency over time, leading to smarter, more responsive traffic systems. This approach not only enhances traffic flow but also reduces accidents, fuel consumption, and travel times, making transportation systems safer and more sustainable.
Traditional vs. Agentic AI Real-Time Traffic Monitoring and Issue Resolution
Aspect |
Traditional Traffic Management |
Agentic AI Traffic Management |
Data Collection |
Manual or limited to basic sensors |
Comprehensive, real-time data from IoT devices and cameras |
Analysis |
Reactive; based on historical data |
Proactive; predictive analytics using machine learning |
Issue Resolution |
Time-consuming and manual |
Automated, real-time interventions |
Traffic Flow Optimization |
Limited to static timing of signals |
Dynamic optimization using real-time traffic conditions |
Scalability |
Difficult to scale with growing urbanization |
Scalable across cities with cloud-based AI solutions |
Akira AI: Multi-Agent in Action for Real-time Traffic Monitoring
Fig1: Architecture Diagram of Real-Time Traffic Monitoring
-
Data Collection Agent: This is the first step in the traffic monitoring process, where a wide range of real-time data is collected. The Data Collection Agent sources information from various tools, such as traffic cameras, IoT sensors installed on the roads, GPS tracking devices in vehicles, and other traffic monitoring systems.
These devices continuously provide data on traffic conditions, vehicle speeds, congestion levels, and environmental factors like weather. This steady stream of data forms the foundation for accurate and timely traffic analysis. -
Data Analysis Agent: Once the raw data is gathered, it is processed by the Data Analysis Agent. This agent applies AI models, machine learning algorithms, and data analytics techniques to make sense of the information. It detects traffic patterns, such as peak congestion periods, unusual traffic behavior, or vehicle bottlenecks.
It can also identify anomalies like accidents, broken-down vehicles, or road hazards. By categorizing this information, the Data Analysis Agent can differentiate between routine traffic patterns and exceptional events that require attention. -
Prediction Agent: After analyzing the data, the processed information is sent to the Prediction Agent. Using machine learning models and historical traffic data, this agent forecasts future traffic conditions, such as potential congestion points, upcoming delays, or areas of concern.
The Prediction Agent can estimate traffic flow trends, highlight trouble spots, and predict when and where delays are likely to occur. This predictive capability allows the system to anticipate problems before they escalate, giving authorities the chance to prepare and intervene proactively. -
Optimization Agent: Based on the predictions from the Prediction Agent, the Optimization Agent takes action to adjust traffic management systems in real-time. It can dynamically alter traffic signal timings to alleviate congestion, reroute vehicles to less congested roads, or manage lane assignments to optimize traffic flow.
For example, if a specific route is predicted to become congested, the Optimization Agent may change traffic light patterns or implement detour routes to prevent a build-up of vehicles in that area. This helps to smooth traffic flow, reduce waiting times, and prevent potential bottlenecks from worsening. -
Issue Resolution Agent: When a traffic issue such as an accident, roadblock, or other disruptions is detected, the Issue Resolution Agent steps in to resolve the problem. This agent activates automatic measures such as alerting traffic authorities, dispatching emergency services, or notifying drivers about the incident and alternate routes.
If necessary, it can also deploy response teams or activate road clearance protocols. The Issue Resolution Agent plays a crucial role in minimizing the impact of unexpected events, ensuring a swift response to reduce delays and restore normal traffic conditions as quickly as possible.
Use Cases and Applications of AI in Traffic Management
-
Real-Time Traffic Flow Optimization: AI models analyze real-time data to dynamically control traffic lights and reroute vehicles, reducing congestion, minimizing delays, enhancing fuel efficiency, and ensuring smoother traffic flow across urban networks.
-
Accident Detection and Response: Computer vision algorithms monitor traffic camera feeds to detect accidents instantly, identify incident locations, and alert emergency services, enabling faster responses and reducing the impact of traffic disruptions.
-
Traffic Congestion Prediction: Predictive analytics models use historical and real-time traffic data to forecast congestion, identify potential bottlenecks, and recommend preventive measures, such as rerouting or adjusting traffic signal timings.
-
Smart Parking Systems: AI-driven systems analyze parking availability in real-time, guiding drivers to vacant spots using mobile apps or in-car navigation, reducing unnecessary circling, and improving parking efficiency.
-
Public Transportation Optimization: AI systems monitor demand and real-time conditions to adjust bus and train schedules dynamically, improving frequency, reducing overcrowding, and ensuring efficient and reliable public transportation services.
Operational Benefits of Real-Time Traffic Management
-
Improved Productivity: Automated traffic management tasks, such as adjusting signals and detecting incidents, free up human operators to focus on strategic decisions. This leads to a significant improvement in overall system efficiency, enhancing traffic management by up to 80%.
-
Reduced Congestion: By optimizing signal timings, rerouting vehicles, and forecasting traffic patterns, advanced systems improve urban traffic flow, cutting down congestion by up to 30%. This results in reduced travel times and a better experience for commuters.
-
Faster Issue Resolution: With real-time detection of accidents, roadblocks, and other disruptions, the system can respond quickly to incidents, reducing delays by 25%. This ensures smoother traffic operations and allows for quicker recovery from unexpected events.
-
Cost Savings: Optimized traffic flow helps reduce fuel consumption and operational costs, resulting in a higher return on investment for municipalities. These efficiencies also support environmental sustainability by minimizing fuel wastage and lowering emissions.
Technologies Transforming Real-Time Traffic Management
-
Computer Vision: Computer vision technologies analyze live video feeds from traffic cameras to detect accidents, unusual events, and traffic conditions in real-time, enabling instant alerts and enhancing situational awareness for better response times.
-
IoT Devices: IoT sensors and GPS systems collect real-time data on traffic flow, vehicle speed, and road conditions. This data helps in monitoring traffic patterns and provides valuable insights for decision-making and optimization.
-
Machine Learning Models: Machine learning algorithms analyze historical and real-time traffic data to predict traffic patterns, identify congestion risks, and optimize traffic signal timings, improving overall flow and reducing bottlenecks across urban areas.
-
Cloud Computing: Cloud computing provides scalable infrastructure for storing and processing large volumes of traffic data. It enables quick data access, real-time analytics, and centralized management, supporting advanced AI and machine learning applications in traffic management.
-
Edge AI: Edge AI processes data locally at traffic signal devices or IoT sensors, enabling faster decision-making and real-time responses. This reduces latency and improves traffic signal adjustments, accident detection, and routing efficiency.
The Future Trends of AI Agents in Traffic Management
-
Autonomous Traffic Control: AI-driven systems autonomously manage traffic signals, rerouting vehicles and adjusting flow patterns in real-time. This ensures seamless movement, reduces congestion, and enhances the efficiency of urban transportation networks.
-
Integration with Autonomous Vehicles: AI enables coordinated movement between traffic control systems and autonomous vehicles, ensuring smoother transitions at intersections, improving safety, and optimizing flow by allowing vehicles to respond to real-time conditions.
-
Enhanced Urban Planning: Traffic data provides valuable insights into patterns, congestion hotspots, and infrastructure needs, allowing city planners to design smarter urban environments with efficient transport systems and optimized resource distribution.
-
Environmental Benefits: By optimizing traffic flow and reducing congestion, these systems minimize fuel consumption and vehicle idling, leading to lower emissions, improved air quality, and contributing to more sustainable, eco-friendly transportation networks.
-
Global Standardization: AI systems can be standardized across regions to create uniform traffic management protocols, enabling consistent and efficient control, reducing complexity, and improving interoperability between different cities and countries.
Conclusion: AI Agents for Real-Time Traffic Management
AI agents are revolutionizing transportation by offering proactive and scalable solutions for traffic management. With capabilities like real-time monitoring, predictive analytics, and automated issue resolution, these systems optimize traffic flow, reduce congestion, and enhance road safety. AI models analyze traffic patterns to predict and prevent bottlenecks, while dynamic traffic light adjustments and real-time rerouting ensure smooth transportation across cities. Additionally, AI's integration with autonomous vehicles further enhances efficiency and safety. As technology continues to evolve, AI agents will become even more integral in building smarter, sustainable, and resilient urban infrastructures. These advancements will not only improve mobility but also contribute to environmental sustainability by reducing emissions and fuel consumption, making cities more livable and efficient for future generations.
Explore More