Key Insights
AI Visual Agents are transforming industries by enabling machines to understand and interpret images and videos with human-like precision. They enhance operational efficiency, improve accuracy, and reduce costs across sectors like healthcare, manufacturing, and retail. By leveraging deep learning and computer vision, these agents can analyze vast amounts of visual data in real-time, offering powerful insights and automating complex tasks.
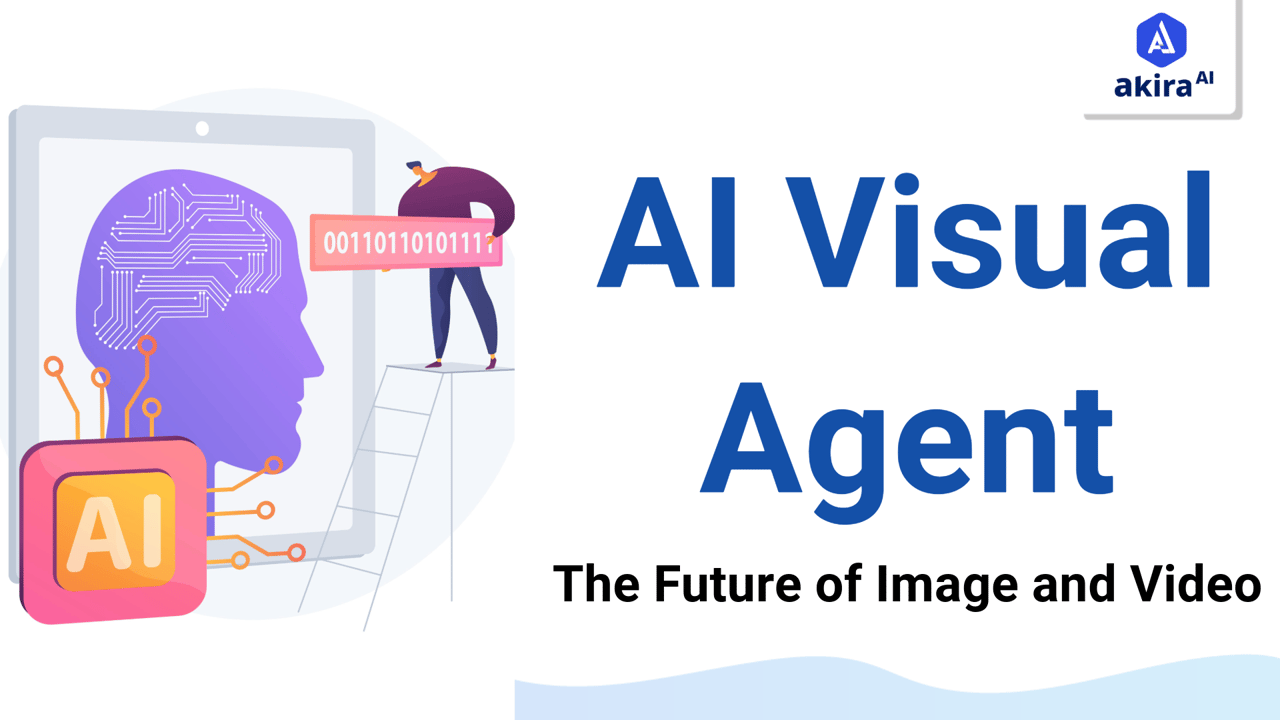
Imagine a world where machines not only see but truly understand images and videos—recognizing patterns, interpreting emotions, and making decisions in real time. This is the transformative promise of AI Visual Agents, the next frontier in artificial intelligence. These cutting-edge systems are revolutionizing industries by enabling machines to analyze visual data with precision once thought exclusive to humans. From enhancing medical diagnostics with detailed imaging to powering autonomous vehicles with unparalleled situational awareness, AI Visual Agents are redefining what's possible.
In this blog, we dive into the exhilarating potential of visual AI, uncovering how it’s shaping the future of image and video understanding, and why businesses are racing to adopt this groundbreaking technology.
Background: Overview of Core Concepts
What are AI Visual Agents?
AI Visual Agents are at the leading edge of what is currently possible in Artificial Intelligence by effectively drawing together many of the most exciting and promising technologies currently available in a manner which assists in the interpretation and manipulation of the visual environment. Such complex systems leverage state-of-art computer vision techniques for performing on-board tasks like object recognition and scene reconstruction, and enable reasoning about spatial dependencies and content evolution respectively.
Why are they Important?
The significance of AI Visual Agents stems from several crucial factors:
-
Data Explosion: The amount of visuals produced each day is incredible: more than 2.5 quintillion bytes of visual information, most of which originates from social networks, security systems, and medical technology. This abundance of video and image data is a clear indication that visual cognition and its processing is now evolving.
-
Business Needs: Approaches, such as quality control and inspection, customer-oriented visual search and product recognition, and applications in security, surveillance, and diagnostics are amongst the most sought by various organizations and institutions. These demands are can be met by the AI Visual Agents.
-
Technological Evolution: Significant advancements in deep learning architectures, increased computational power, improved sensor technologies, and enhanced data storage and processing solutions have created an environment conducive to the development and deployment of AI Visual Agents, making them more effective and accessible.
Akira AI: Multi-Agent in Action
AI Visual Agents base a lot of approaches that integrate various complex parts and procedures to achieve efficient visual interpretation and more interaction. The system and its components It comprises a Visual Perception Module anchored on CNNs and object detection YOLO/Faster R-CNN, and semantic segmentation networks for enrich image understanding.
-
Data Collection and Preparation: Collect various visual datasets meaningful for the application so that more various situations would be chosen. Mark up the data to be used in training and tag it properly, use data augmentation to help increase variability in the data used for training, and generate a cross-validation set when training the model.
-
Model Development: Create key framework blocks like Visual Perception to perform deep image analysis, Decision Module for action decisions based on vision input, and the Interaction Layer to engage with the user and outside environment.
-
Training and Optimization: By so doing, perform an initial training using the prepared datasets to lets the model learn to identify the patterns. This will be followed by a process of model optimization for the specific purpose it is to be employed for and finally system integration test which will check the efficient working of all the sub-systems.
Architecture Diagrams and Explanations of AI Visual Agents
Fig1: Architecture diagram of AI Visual Agents
The implementation of AI Visual Agents includes several layers of components that are intimately involved with the processing of visual information.
-
Input Layer: This layer is responsible for correct processing of data of various formats, such as images, videos, and live broadcasts. Testing also uses input validation and input preprocessing as a means of data quality and data consistency. Also, it handles the entry queue and sorting to facilitate the ability to handle large entries in one lot.
-
Visual Processing Layer: Here, the next computer vision algorithms are used to detect and extract features required from the image. This layer is critical for converting acquired visual data into valuable information since it also includes enhanced and normalized image conversion techniques.
-
Context Understanding: This component performs spatial structuring in the visual data and temporal processing to obtain change data. They incorporate contextual data gathered from the outside environment and therefore extend the knowledge of the context altogether and facilitate better decision-making.
-
Decision Engine: Last but not the least, the Decision Engine assesses the processed information depending on certain rules and policies of the business. It provides prompt suggestion for the AI Visual Agent to take appropriate actions based on the analysis of previous layer and aids in the processing of the visual inputs.
Together, these components create a comprehensive framework that enhances the capability of AI Visual Agents to interpret and interact with the visual world.
Key Benefits of AI Visual Agents
The implementation of AI Visual Agents offers numerous advantages:
-
Enhanced Accuracy and Reliability: AI Visual Agents normally have recognition rate of well over 95% within the image-controlled surroundings, therefore greater accuracy. When using the enhanced filtering procedures, the number of false positives can be decreased by approximately 60%, which creates fewer unreliable results. In addition, they can produce results with equal efficiency regardless of the volume of data they are required to analyze.
-
Operational Efficiency: These agents can deal with thousands of images per second thereby improving the processing time greatly. This means that they are able to cut down the amount of manual effort required to manage them by up to 80%, in terms of resources. Also, their scalable architecture enables them to address the increasing data volumes that characterize most organizations.
-
Cost Benefits: It reduce the essence of labor expenses as it eliminates the overly costly visions of mistakes during repetitive visual inspections. This in turn results in decreased cost of possible errors, as well as facilitating the optimization of human resources by distancing employees from labor intensiveness and granting them time for the most significant tasks.
-
Strategic Advantages: Businesses take advantage of being on the forefront in adopting AI Visual Agents therefore leaving competitors behind in the marketplace. These agents contribute to innovation in as much as it creates the conditions necessary for the creation of new product and services while at the same time also help improve customer satisfaction by improving on how users interact with products.
Case Studies of AI Visual Agents
AI Visual Agents find applications across various industries:
E-commerce and Retail: AI Visual Agents enhance visual search by enabling product discovery through image uploads, offering similar item recommendations, and assisting with style matching. They also improve inventory management with real-time stock monitoring and planogram compliance.
Healthcare Applications: In healthcare, AI assist with medical imaging, providing diagnostic support for radiologists and real-time surgical guidance. They enhance patient monitoring through fall detection and movement analysis.
Manufacturing and Quality Control: It boost production line monitoring by facilitating defect detection and assembly verification. They also ensure safety compliance through PPE detection and hazard identification.
Smart Agriculture: In agriculture, AI Visual Agents are used for crop monitoring and health assessment through drone imaging. They enable early detection of pests and diseases, optimize resource allocation, and improve yield predictions based on visual data analysis.
Integration with Akira AI
-
Initial Configuration: Akira AI can begin through the setting up of AI Visual Agents to take up individual responsibilities based on the business requirements. It has been established to comprise setting specific constraints like the processing modes, the data in question, and contexts in which they are to function, empowering agents to be fine-tuned for the intended tasks.
-
Image and Video Analysis: This allows to organize image analysis, using AI Visual Agents within the system. These agents can also recognize, categorize or even index pictures and pictures making sectors such as visual search in the retail business or surveillance in security better.
-
Custom Model Implementation: Akira AI can figure out how to work with custom built models that are meant for unique tasks in industries enabling it to serve the industries better with solutions that meet their need.
-
Interactive Features and Automation: Through AI Visual Agents, Akira AI can deploy active functionalities that work with graphic stimuli in real-time. It also enables real-time user interactivity and eliminates the need for users to scrutinize data in the search for anomalies and then follow this up by manually activating action mechanisms or other alerts, thus enhancing operating effectiveness, all things considered.
Challenges and Limitations of AI Visual Agents
-
Processing Requirements: Real-time execution of AI Visual Agents requires massive computational power on account of numerous image computations. This results in memory management problem, which can affect the performance and the efficiency of a system.
-
Data Quality: They also indicate that variability in input quality is a very difficult problem, due to variations in lighting and perspective. Further, noise and interferences are a threat to the quality of the visual data that is to be extracted.
-
Integration Complexity: Governing system compatibility can be challenging when making API integrations because this aspect typically has issues that need consideration. Moreover, improvement across multiple components may make its implementation quite challenging.
-
Operational Limitations: By nature, AI Visual Agents are bounded by certain resource limitations including particular hardware prescriptions, space, and bandwidth among others, which may limit their usability.
-
Implementation Hurdles: Otherwise, the massive amount of training material is obligatory for a model to provide high results, as well as acquiring specific knowledge from professionals. Other factors which are of significant influence with regard to the durability of the system are the maintenance concerns with the system on a continuous basis.
Future Trends in AI Visual Agents
-
Augmented Reality: AI Visual Agents expand augmented reality possibilities through scene understanding, real-time adaptive interactions and visual objects that are merged into actual physical environment. They proposed the said technology as ideal for gaming, educational and training applications.
-
Autonomous Systems: From an autonomous system viewpoint, the basic aspects of an AVS include self-driving cars in which AI Visual Agents are essential for accurate guidance and decision-making. They also help for robotic control and aircraft control so that the machines can know about the world and move safely.
-
Smart Environments: Smart Environment Engagement through AI Visual Agents is advanced through city and building facilities enhancement. They improve traffic lights through real time surveillance, to produce effective structural avenues for the community members and other users.
-
Healthcare Applications: In health care, the AI Visual Agents improve the medical imaging analysis, thus reducing time and misdiagnosis of abnormalities. They also facilitate augmented surgical environment since they offer operational data overlays to support the surgeons’ precision on the surgical procedures.
Conclusion:AI Visual Agents
AI Visual Agents are one of the groundbreaking technologies that are revolutionalizing how machines perceive and process visual content paving way to numerous opportunities in different fields. We can foresee that, as these agents develop, the understanding which accompanies them will progress further, originality which accompanies these agents as well as a better integration into previously existing systems, elevated rates of automation, and smoother experiences when interacting with the agents. However, implementation involves the technical specifications, operational necessities, advanced ethical issues and resource utilization. These benefits will make organizations that harness this technology gain competitive advantages in operation efficiency, customer satisfaction, innovation, and competitiveness.