Key Insights
In a rapidly evolving consumer packaged goods (CPG) landscape, AI-driven quality monitoring is revolutionizing product excellence. Imagine a system that detects microscopic defects, predicts potential quality issues, and ensures consistent product standards—all in real-time. This intelligent approach is not just transforming quality control, it's redefining manufacturing precision and customer satisfaction.
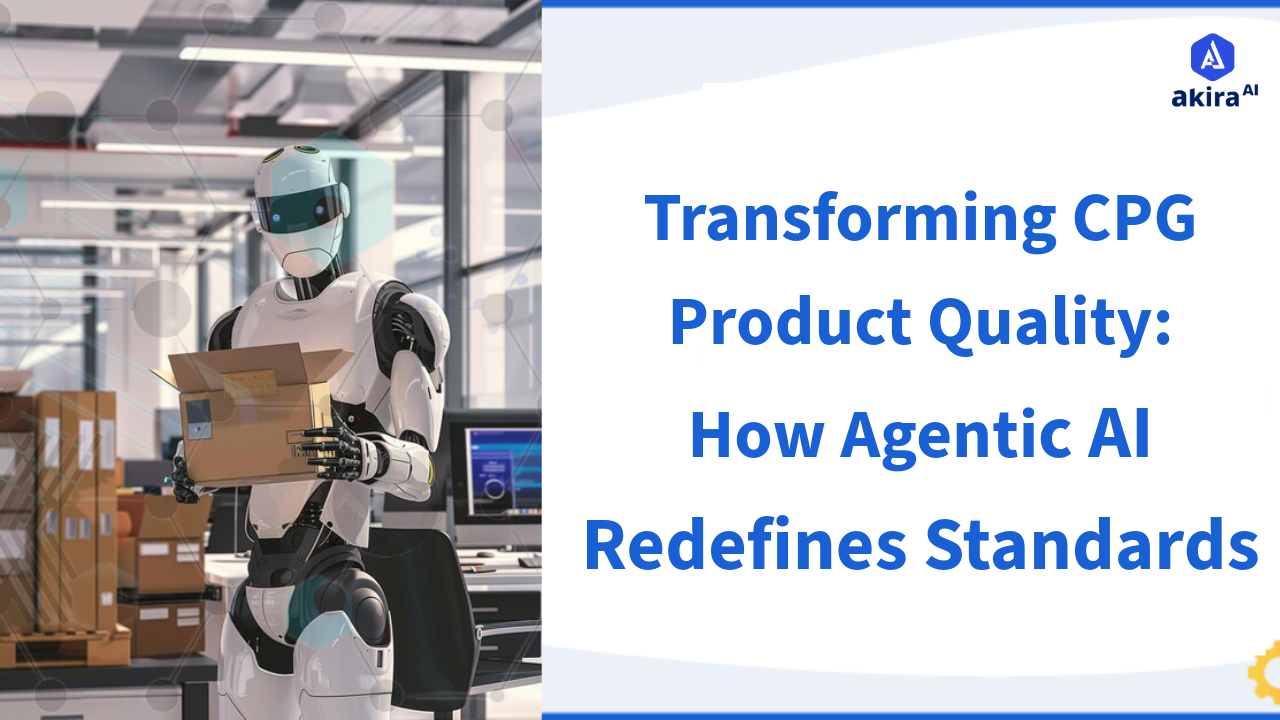
The pressure to maintain impeccable product quality in the consumer-packaged goods (CPG) industry has never been greater. With the rise of AI in quality control, CPG companies are now equipped with powerful tools to enhance quality assurance and ensure product consistency. Agentic AI technologies, such as machine learning and computer vision, are enabling faster, more accurate defect detection and predictive maintenance, transforming traditional quality monitoring methods.
For this reason, this technology is being adopted widely as a strategic tool in the CPG industry as it tries to provide consumers with better products and meet competition more effectively.
What is Product Quality Monitoring and Control?
Product quality monitoring and control refers to the processes and systems employed by companies to ensure that their products meet predefined standards and specifications. In the consumer-packaged goods (CPG) industry, these processes are particularly important due to the massive scale of production and the need for consistency across large product volumes.
It involves continuous assessment and adjustment of production processes to guarantee that each product batch adheres to established product quality frameworks, ensuring quality assurance (QA) and quality control (QC) at every stage of production.
Key Concepts of Product Quality Monitoring and Control
Necessary concepts of product quality control and improvement are connected with a series of processes that should take place in order to maintain the quality of a product during its use. These activities include:
Quality Assurance (QA): QA involves proactive measures to ensure that products are designed, developed, and produced in a way that they meet established quality standards.
Quality Control (QC): QC is concerned with the identification of spoilage factors during the manufacturing cycle and guaranteeing that commodities are perfect prior to sale.
Product Quality Framework: A comprehensive set of guidelines, standards, and practices that define quality requirements and processes for managing and maintaining product quality.
Monitoring Systems and Tools: These systems track key quality indicators, such as dimensions, weight, color, texture, or any other attributes relevant to the product's quality.
Traditional Way of Product Quality Monitoring and Control
Before the advance of technology, most CPG industries used manual quality monitoring that involved human personnel in performing visual checks on products, performing simple physical tests, and embracing the feedback from the production. These methods were limited to routine inspections, often missing real-time quality assessments. Despite some effectiveness, they had several drawbacks:
-
Time-Consuming Inspections: Manual inspections waste much time, which requires major labor input. Not to mention that they can be easily biased by human prejudice. This inefficiency hampers efficiency and cost since all batches have to undergo scrutiny by those in charge.
-
Inconsistent Quality Control: Possible deviations in human evaluation might mean that two products of a similar nature would receive dissimilar ratings. This subjectivity caused a functionality that made it hard to ensure that product quality was consistent over the years, which was a problem.
-
Escalating Operational Costs: An inadequate workforce executes repetitive inspections and tests and increases the number of employees required to understudy, thereby escalating operating costs. Also, the pointed loss due to missed inspections in terms of errors and defects increases the cost in business organizations.
-
Lack of Real-Time Insights: Traditional methods cannot gather and analyze data in real-time, which limits opportunities for predictive insights. Precisely because of this lack of real-time data analysis, businesses frequently have no way to predict new quality problems before they pose challenges to production.
-
Delayed Defect Detection: Issues could be realized in the production line only towards the end of a particular production run, leading to many losses and ineffectiveness. These delays make it hard to do prompt rectifications, which then may result in additional product returns or reprocessing.
Agentic AI is transforming quality control in manufacturing by automating defect detection, enhancing operational efficiency, ultimately improving product quality and customer satisfaction. Learn more about its impact on various industries, from automotive to pharmaceuticals.
Impact on Customers Due to Traditional Product Quality Monitoring and Control Processes
The limitations of traditional product quality monitoring directly affect customers, as inconsistent or subpar products lead to dissatisfaction and erode trust. Many businesses lose their customers' loyalty and, at the same time, create a poor image if their products do not meet quality standards. Specific impacts on customers include:
-
Inconsistent Product Experience: Slight quality deviations in food taste, texture, color, size, shape, packaging, or any other characteristic of a product model that customers consider important may result in dissatisfaction. This inconsistency can prompt customers to seek alternatives, harming brand loyalty.
-
Production Delays and Shortages: Manual inspections can cause delays in identifying quality issues, which leads to production slowdowns and inventory shortages. These delays result in empty shelves, affecting product availability and customer experience.
-
Escalating Operational Costs: If defects are not identified at the beginning, then the firm could be forced to undertake costly reprocessing or product recall. Such costs are transferred to the consumers, thus causing an increase in the prices and/or a decrease in other qualifiers of genuine products.
-
Damaged Brand Reputation: Negative experiences resulting from inconsistent or faulty products may greatly help to damage a brand. Sustained discontent can be easily disseminated word-of-mouth or in writing and force current as well as potential customers away.
-
Frustrated Consumers: Consumers who encounter subpar products may feel frustrated, especially if their expectations are not met due to poor product quality. Hence, this level of frustration can decrease customer satisfaction and brand loyalty in the long run.
-
Rising Returns and Complaints: Failure to detect the above defects early raises return rates and customer complaints, which generate other costs for administration and operation. This not only has an impact on profitability but also puts immense pressure on the customer service facilities.
Akira AI: Multi-Agent in Action 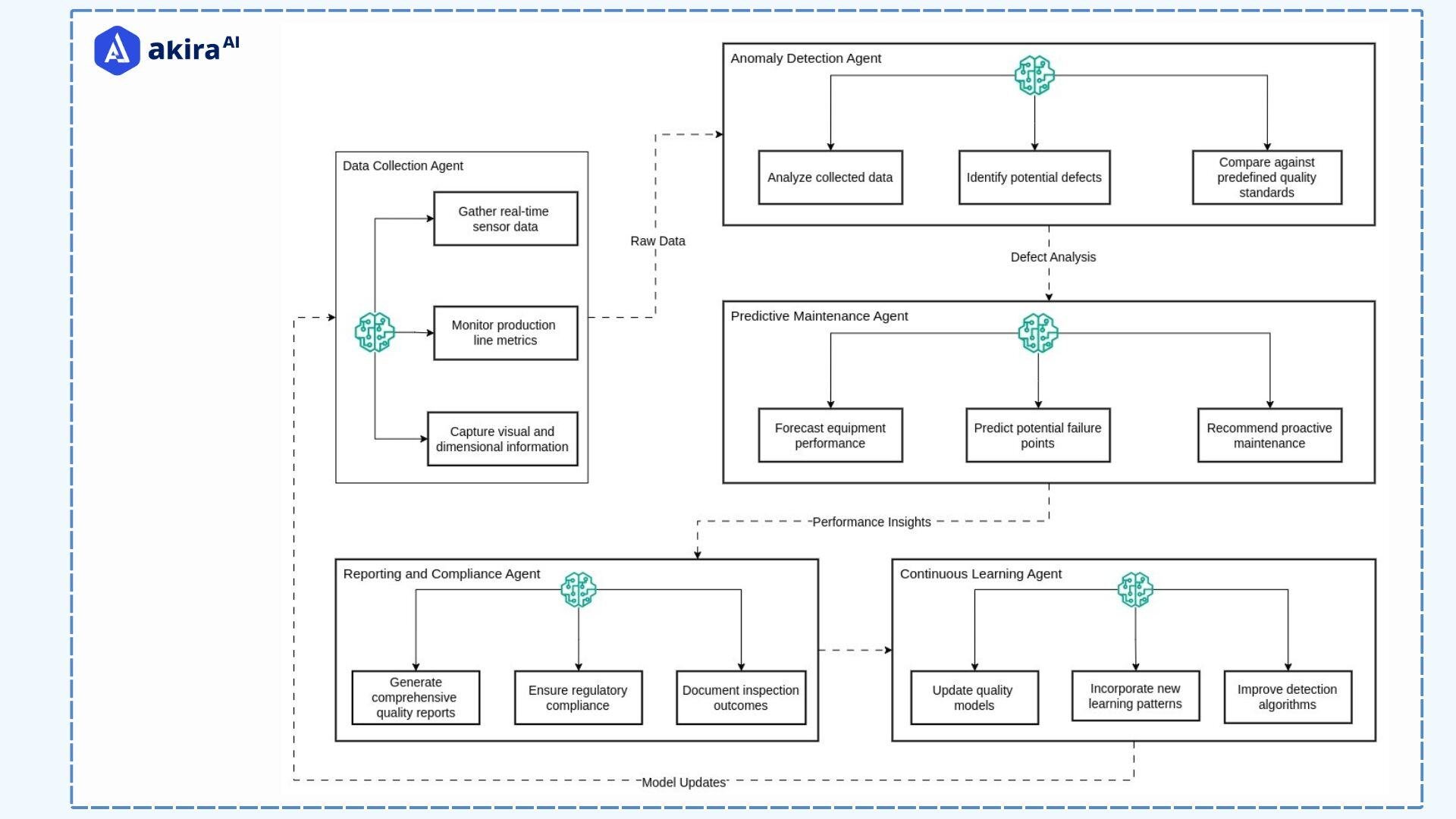
Fig1: Architecture Diagram of Product Quality in CPG
Akira AI is a top solution for AI-driven product quality control and quality assurance in the CPG industry. Using a multi-agent system, it coordinates various agents to handle specific tasks within the quality management process. Key agents in Akira AI’s agentic workflow include:
-
Master Orchestrator: The Master Orchestrator is the central hub that coordinates and manages all other agents. It guarantees the smooth and fast functioning of the overall quality control process by distributing the activities among the specific agents depending on the current information and system needs.
-
Inspection Agent: The Inspection Agent utilizes computer vision to identify flaws in products through images and videos of the products in the production process. This is important for the realization of aesthetic needs in order to guarantee that visions of quality are met.
-
Prediction Agent: The Prediction Agent analyzes historical data and uses predictive analytics to forecast when quality issues are likely to arise. By identifying potential problems ahead of time, corrective actions can be taken proactively.
-
Sensor Agent: The Sensor Agent works in tandem with IoT devices to monitor environmental conditions (such as temperature and humidity) and machinery performance to detect any factors that might compromise product quality.
-
Automation Agent: The Automation Agent automates repetitive tasks related to quality testing, such as checking product weights or conducting routine inspections, thus reducing the need for manual intervention.
Prominent Technologies in Agentic AI Product Quality Monitoring and Control
AI and machine learning (ML) technologies have revolutionized quality assurance and quality control processes in the CPG industry. Some prominent technologies driving this transformation include:
-
Computer Vision: Computer vision borrows from cameras and advanced algorithms that help detect visual defects in products. With this technology, one is able to detect phenomena like color differences, shape defections, or wrong packaging on the production line.
-
Predictive Analytics: By analyzing historical data, AI models can predict when and where quality issues are likely to occur in the production process, allowing companies to address potential problems before they arise.
-
Robotic Process Automation (RPA): RPA can automate repetitive tasks like data entry or analysis, reducing human error and improving the efficiency of quality control workflows.
-
IoT Sensors and Smart Devices: IoT devices located on manufacturing lines offer information on temperature, humidity, pressure, and other qualities affecting the final result of the manufacturing.
-
Natural Language Processing (NLP): NLP allows AI to analyze feedback from customers, helping businesses detect issues and improve product development.
-
Machine Learning Algorithms: These algorithms analyze vast amounts of data and identify patterns that can be used to improve product quality and predict future problems.
Explore how Agentic AI optimizes vendor management by automating tasks like supplier evaluation, performance monitoring, and risk assessment, providing real-time insights for proactive decision-making.
Successful Implementations of AI Agents in the Consumer-Packaged Goods Industry
Several CPG companies have successfully implemented AI-driven solutions to improve their quality management processes. For instance:
-
Nestlé: Nestlé has leveraged AI to analyze consumption data to create market research reports to respond to shifts in consumer eating habits. This approach has helped them optimize product quality and reduce waste in their production processes.
-
Unilever: Unilever implemented AI-driven predictive analytics to monitor product quality and optimize its supply chain. This initiative resulted in a significant waste reduction and improved production speed, showcasing the effectiveness of AI in enhancing operational efficiency.
-
Colgate-Palmolive: Colgate-Palmolive is utilizing generative AI technology to optimize its supply chain and improve product availability. Their AI-enabled chatbot helps streamline customer interactions, ensuring that consumer needs are met promptly while minimizing operational costs.
-
Procter & Gamble (P&G): P&G has adopted AI tools for real-time inventory management and demand forecasting, which has led to a reduction in stockouts and excess inventory. This AI application has significantly enhanced their production efficiency and customer satisfaction.
Future Trends: How AI Agents Supersede Other Technologies
-
AI and Machine Learning Evolution: Advanced utilization of artificial intelligence and machine learning will act as a basis for greatly improved and efficient quality control and monitoring that will fundamentally change real-time defect identification and process enhancement.
-
Next-Level Predictive Insights: With predictive analytics, it will be possible not only to predict potential quality problems more accurately but also to offer recommendations on preventive measures to achieve quality production standards.
-
Full-Scale Automation in Quality Control: Automation of the QC function will greatly reduce the reliance on people and increase the efficiency, precision, and uniformity of the detection line.
-
Cutting-Edge Sensor Technologies: Capturing highly detailed and accurate data will be possible with the help of enhanced and more sensitive sensor technologies, which will enhance the monitoring and control systems at the micro level of the production processes.
-
Instant, Data-Driven Decision Making: AI-driven systems will provide instantaneous, actionable insights, ensuring that responses to quality deviations and operational challenges are fast, informed, and aligned with production goals.
Conclusion: AI Agents for Product Quality Monitoring and Control
AI-driven product quality monitoring represents a transformative approach in the CPG industry with smarter, faster, and more precise quality control processes. Companies can ensure unparalleled product consistency, operational excellence, and heightened customer satisfaction by harnessing the power of intelligent agents, cutting-edge technologies, and actionable data insights.
The future of manufacturing is evolving beyond simply meeting production goals—it’s about consistently delivering superior products that not only meet but exceed consumer expectations, reinforcing brand loyalty and driving market leadership.
Explore More Order Management in the CPG Industry
Dive Into Order Fulfillment with AI Agents