Key Insights
Modern credit analysis emphasizes the shift toward more accurate and efficient financial evaluations. By automating processes and analyzing extensive datasets, organizations are better equipped to assess risk and make more informed lending decisions. These improvements lead to more personalized credit offerings, effective risk management, and enhanced portfolio performance, ensuring better financial outcomes for both lenders and borrowers.
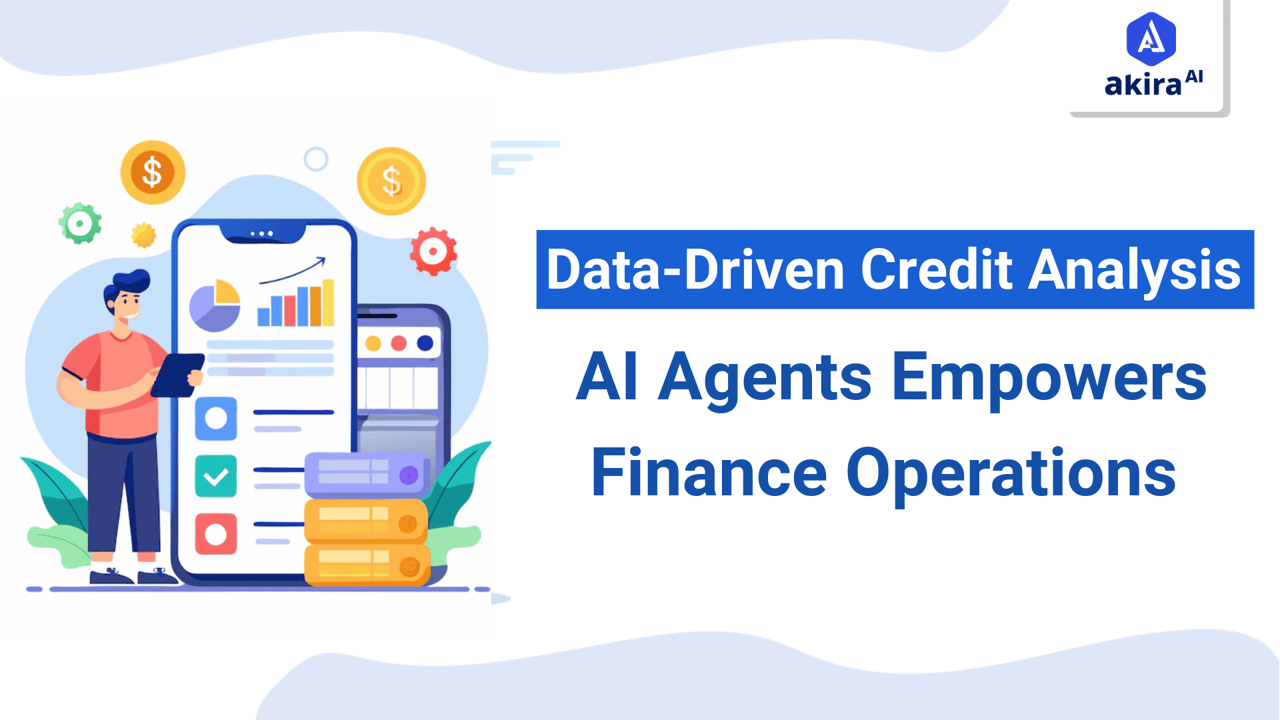
Credit risk analysis is no longer confined to traditional metrics like credit default swaps (CDS); it now encompasses data-driven methodologies that leverage sentiment and topic analysis to unearth deeper financial insights. A recent study analyzing 3,386 Dow Jones analyst reports from 2009 to 2020 found that sentiment and specific topics in these reports correlate strongly with CDS spreads, offering a nuanced perspective on risk assessment. This highlights the untapped potential of combining established credit metrics with qualitative insights from financial experts.
As non-financial companies and banks face growing pressure to understand credit exposure and avoid counterparty defaults, Agentic AI-driven tools have emerged as transformative solutions. These technologies can analyze unstructured data, automate interpretations, and provide real-time recommendations, enabling risk managers to stay ahead in volatile markets. By integrating AI Agents, organizations can redefine credit analysis, making it more precise, scalable, and responsive to evolving financial landscapes.
What is Credit Analysis in Financial Services?
Credit analysis in financial services is the systematic evaluation of an individual, business, or institution's ability to meet financial obligations, such as repaying loans or fulfilling contractual agreements. It is a critical process for lenders, investors, and risk managers to minimize the risk of default and ensure sound financial decision-making. The analysis combines both quantitative and qualitative factors. Quantitative assessment involves examining financial statements, ratios like debt-to-equity, and cash flow patterns to measure financial health. Qualitative analysis delves into external factors, such as market conditions, industry trends, and management quality, which may influence creditworthiness.
A Brief Overview of Agentic AI Credit Analysis in Financial Services
Agentic AI-powered credit analysis in financial services transforms how financial institutions assess a borrower’s creditworthiness. This modern approach automates the gathering and validation of data, incorporating a mix of structured financial records and unstructured sources like market trends and news. By leveraging advanced models, the system predicts potential risks more accurately and faster than traditional methods.
This shift reduces biases, enhances decision-making, and improves consistency in evaluating credit risk. It helps financial institutions manage portfolios more proactively, detecting signs of potential default and improving fraud detection. As a result, institutions can offer more personalized lending options and optimize credit strategies with greater operational efficiency, all while maintaining a higher level of transparency and reducing manual effort. The future of credit analysis lies in harnessing these systems for smarter, more responsive financial services.
Traditional vs. Agentic AI Credit Analysis
Feature |
Traditional Credit Analysis |
Agentic AI Credit Analysis |
Data Processing |
Manual data collection and review |
Automated data ingestion and real-time analysis |
Scalability |
Limited by human effort and time |
Highly scalable with computational power |
Accuracy |
Susceptible to human errors |
Enhanced accuracy through algorithmic predictions |
Speed |
Time-intensive |
Rapid and near-instantaneous |
Fraud Detection |
Reactive, based on identified discrepancies |
Proactive, leveraging anomaly detection models |
Cost |
Higher due to labor-intensive processes |
Lower operational costs |
Decision-Making |
Experience-based |
Data-driven with explainable AI models |
Akira AI: Multi-Agent System in Action
Fig1: Architecture Diagram of Automated Credit Analysis
-
Credit Application Submission & Financial Review Request: The process begins with the submission of a credit application or a request for financial review. This triggers the credit analysis workflow that begins with the Master Orchestrator Agent, which is responsible for coordinating the entire process.
-
Data Collection & Validation: The Data Collection and Validation Agent gathers data from various sources, such as APIs and financial records. It ensures data accuracy by checking for completeness, flagging incomplete or incorrect data, and validating the data sources. This is essential for maintaining the integrity of the entire analysis process.
-
Financial Metrics & Risk Assessment:
-
The Financial Metrics and Ratios Analysis Agent calculates key financial metrics, such as financial ratios, to assess the client’s financial health.
-
The Risk Assessment and Scoring Agent analyzes the 4 Cs of credit (Character, Capacity, Capital, and Collateral) and generates a credit score based on the financial analysis.
-
-
Predictive Modeling & Trend Analysis: The Predictive Modeling and Trends Agent analyzes historical data, market trends, and potential risks, including the likelihood of credit default. It uses machine learning models to predict default risk and forecast future creditworthiness.
-
Reporting and Recommendations: Finally, the system generates a Credit Analysis Report through the Master Orchestrator Agent. This includes the credit score, risk assessment, and tailored recommendations to support decision-making for financial institutions, improving their ability to manage risk effectively.
Use Cases of Data-Driven Credit Analysis
-
Loan Underwriting: Automates risk assessment using predictive algorithms, accelerating decision-making, and reducing biases in credit approval processes, ensuring fairer and more efficient loan evaluations.
-
Fraud Detection: Utilizes real-time anomaly detection algorithms to flag suspicious transactions and prevent fraud, enhancing security and protecting financial institutions from potential losses.
-
Customer Profiling: Creates comprehensive borrower profiles by integrating data from financial records, social behavior, and market trends, enabling personalized credit solutions and deeper customer insights.
-
Portfolio Management: Continuously monitors loan portfolios, identifying underperforming assets, and suggesting proactive measures to reduce non-performing loans (NPLs) and improve portfolio health.
-
Market Segmentation: Analyzes borrower data to classify customers into high-risk or high-reward segments, enabling targeted credit offerings and improving risk-adjusted returns.
Operational Benefits of Credit Analysis Financial Services
-
Workload Automation: Handles 80% of credit analysis tasks by 2025, automating routine processes, reducing manual effort, and freeing resources for strategic initiatives, improving scalability and efficiency in institutions.
-
Productivity Boost: Increases productivity by 30%, enabling analysts to focus on high-value activities like strategy development and client engagement, instead of repetitive, time-consuming operational tasks.
-
Efficiency Gains: Enhances operational efficiency by 25%, reducing processing time for credit decisions and improving speed, accuracy, and overall effectiveness in risk management.
-
Fraud Prevention: Detects anomalies in real-time, flagging fraudulent activities before they escalate, protecting financial institutions, and ensuring safer operations.
-
Risk Mitigation: Forecasts potential risks using predictive analytics, enabling proactive decision-making and minimizing losses from bad debts or market fluctuations.
-
Improved Customer Experience: Offers personalized credit solutions based on insights, building trust and loyalty while enhancing satisfaction and retention.
Technologies Transforming Agentic AI Credit Analysis
-
Machine Learning (ML): ML algorithms are essential in analyzing vast datasets, learning from historical trends, and predicting potential credit risks. They allow AI agents to improve their decision-making capabilities over time, such as adjusting risk predictions based on new data and market trends.
-
Natural Language Processing (NLP): NLP is used to analyze unstructured data, such as news articles, analyst reports, and social media sentiment. By extracting valuable insights from these sources, AI agents can enhance credit assessments by factoring in qualitative elements like market sentiment and company reputation.
-
Predictive Analytics: This technology uses historical data and advanced algorithms to predict future trends, including potential defaults. Predictive models allow AI agents to assess risks proactively and provide more accurate credit scores and financial recommendations.
-
Knowledge Graphs: Knowledge graphs help AI agents to contextualize financial data and relationships between entities (e.g., companies, their financial health, and industry trends). This enables more sophisticated analyses by connecting various data points, providing a broader understanding of credit risk.
-
Automated Decision-Making: AI agents can automate the entire credit analysis process—from data collection to generating reports. By reducing manual intervention, automation speeds up credit decision processes, enhances scalability, and reduces human error.
The Future of AI-Powered Credit Analysis in Financial Services
-
Hyper-Personalized Lending: Loan products are tailored to individual borrowers' needs using insights from AI, ensuring optimal terms, improving customer satisfaction, and increasing accessibility for underserved segments of the population.
-
Decentralized Credit Systems: Blockchain enables secure, tamper-proof global credit scoring systems, offering a transparent and reliable framework for lenders while reducing the risk of fraud and improving financial inclusion.
-
Explainable AI: Transparent AI models ensure decisions are understandable, meeting regulatory requirements and fostering trust by allowing stakeholders to see the rationale behind credit approval or rejection.
-
Continuous Monitoring: Real-time systems provide 24/7 credit risk management, tracking borrower behavior, market trends, and potential risks to mitigate issues before they escalate.
-
Integration with IoT: IoT data, such as payment habits or location trends, is incorporated into credit analysis, providing deeper borrower insights and enabling more informed lending decisions.
Conclusion: AI Agents for Credit Analysis
In conclusion, the future of credit analysis in financial services lies in leveraging advanced technologies to streamline decision-making and enhance risk assessments. These tools provide a more accurate understanding of creditworthiness by automating processes, improving the efficiency of financial operations, and offering real-time insights into risk factors. As these technologies continue to evolve, they will enable better risk management and more personalized services, ensuring a more transparent and effective credit evaluation process. Embracing these innovations will help financial institutions remain competitive while enhancing the customer experience and ensuring smarter financial decisions.