Key Insights
Energy loss detection and prevention in transmission focuses on identifying and addressing inefficiencies in power systems to minimize energy waste. By targeting both technical losses, such as equipment inefficiencies and resistance in transmission lines, and non-technical losses like theft or system errors, organizations can optimize energy flow and reduce costs. This approach offers significant operational benefits, including enhanced efficiency, prolonged equipment lifespan, and improved regulatory compliance, while supporting sustainability goals.
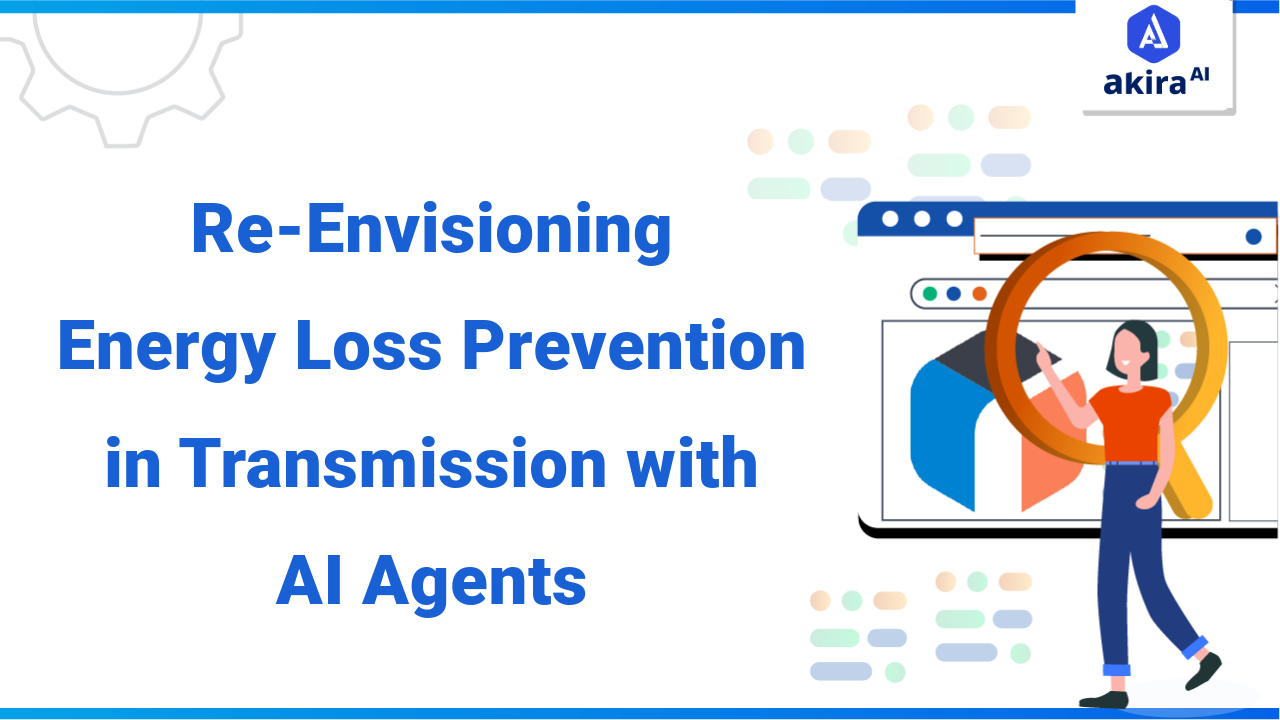
The reigning concern in contemporary society is energy efficiency due to the hasty ever-growing demand for power. Given the increasing power requirements and the urgent need for power transmission systems that are sustainable, energy waste during transmission remains one of the largest hurdles. Most of the techniques that have been employed to monitor and control the losses incurred in electrical grids have not worked efficiently in several situations.
Nevertheless, the progress of artificial and machine learning has made energy transmission systems smart and much more efficient than before. This blog discusses how Agentic AI is changing the endgame in loss reduction of transmission network energy, providing a solution to what has been considered the oldest problem of all.
What is Energy Loss Detection and Prevention in Transmission?
Energy loss detection and prevention in transmission refers to identifying inefficiencies and minimizing energy waste in the power systems that support telecom networks. These losses can occur in various forms, such as technical losses (due to resistance in wires or equipment inefficiencies) and non-technical losses (such as energy theft or system mismanagement).
Telecom networks rely heavily on robust power systems, including base stations, data centers, and transmission lines, to function seamlessly. Losses in energy transmission can lead to higher operational costs, reduced network reliability, and increased environmental impact due to wasted energy.
To address this, advanced technologies like AI agents are employed. These systems monitor energy consumption, detect anomalies, and optimize energy flow. For example, AI can analyze data from power systems to identify patterns, predict equipment failures, and suggest preventive measures. By implementing these solutions, telecom companies can enhance network efficiency, reduce costs, and contribute to sustainable operations.
A Brief Overview of Energy Loss Detection and Prevention in Transmission
Energy loss detection and prevention in transmission is a critical area of focus in industries such as energy, telecommunications, and utilities. It involves identifying, analyzing, and mitigating the factors that contribute to energy inefficiencies during power transmission. These losses can be broadly categorized into:
-
Technical Losses: Energy dissipated due to the inherent resistance of transmission lines, transformers, and other infrastructure components. This includes heat loss, faults in equipment, and inefficiencies in power conversion or distribution.
-
Non-Technical Losses (NTL): Losses resulting from human or system errors, such as inaccurate metering, billing issues, or unauthorized energy usage (e.g., theft).
In telecommunications, energy loss impacts the performance and sustainability of the network, especially in power-hungry systems like base stations, data centers, and transmission networks. Advanced tools like AI agents are now being used to monitor and analyze energy flow, identify anomalies, and optimize system performance. These intelligent systems leverage machine learning, real-time data analysis, and predictive modeling to detect potential inefficiencies, suggest corrective measures, and enhance energy management.
Traditional vs. Agentic AI in Energy Loss Detection
Aspect |
Traditional Approaches |
Agentic AI Approaches |
Data Collection |
Manual readings, limited sensors |
Real-time data collection from IoT sensors |
Maintenance Approach |
Scheduled inspections and repairs |
Predictive maintenance based on continuous data analysis |
Energy Efficiency |
Basic optimization, often inefficient |
Advanced algorithms for real-time energy optimization |
Operational Costs |
Higher costs due to manual labor and reactive actions |
Lower costs through automation and predictive insights |
System Reliability |
Prone to human error and outdated systems |
Increased reliability through constant monitoring and AI-driven decisions |
Detection Speed |
Slow and often reactive |
Immediate anomaly detection with predictive alerts |
Akira AI: Multi-Agent in Energy Loss Detection and Prevention in Action
The application of artificial intelligence as an agent for the identification and mitigation of energy losses is a complex activity involving many levels of operations for optimal results. This is how these agents function in the field:
-
Data Collection Agent: The transmission network is fitted with sensors that constantly relay information about the system to the hub. Such data includes electrical voltage and current, temperature, and load within the various components of the grid.
-
Data Processing Agent: This agent analyzes the real-time stream of incoming data using machine learning techniques to find patterns that suggest excessive energy consumption. Thus, a threatening energy leak can be suspected and located when the stream of data is examined.
-
Prediction and Optimization Agent: This agent makes predictions, using the analytics above, on system failures or inefficiencies and alters the energy supply network for losses in particular sections of the grid, that are likely to be overloaded, to avert losses.
-
Actionable Insights Agent: This agent provides actionable information and warns operators about inefficiencies. Such warnings may include simple alerts on the presence of anomalies and, in some cases, more complex instructions on the recommended changes that need to be made to the system to minimize the loss of energy.
Use Cases and Applications of Energy Loss Detection and Prevention
Energy loss detection and prevention plays a pivotal role in optimizing operations across various sectors, particularly in telecommunications and energy management
-
Smart Grid Management: Energy loss detection helps optimize power distribution within smart grids by identifying inefficiencies, equipment malfunctions, and unauthorized usage. This ensures a reliable electricity supply and minimizes waste.
-
Telecommunications Network Management: In telecom infrastructure, energy loss detection improves the efficiency of components such as base stations and data centers by identifying and mitigating inefficiencies in power consumption.
-
Preventive Maintenance: Energy transmission systems benefit from regular monitoring that identifies potential failures before they occur. Preventive measures, like maintaining transmission lines and transformers, reduce unexpected outages and energy wastage.
-
Integration of Renewable Energy: Energy loss detection supports the seamless integration of renewable energy sources into power grids, ensuring efficient transmission without overloading or energy dissipation.
-
Demand and Load Balancing: Dynamic load balancing distributes electricity evenly across networks, reducing overloading and associated energy losses. This ensures stability during peak demand periods.
-
Compliance and Sustainability: Energy detection and prevention align with regulatory standards by providing accurate reporting of energy usage and losses. This supports sustainability goals and efficient resource management.
The Operational Benefits of Energy Loss Detection and Prevention
-
Improved Efficiency and Reduced Costs: It ensures that energy is utilized optimally, reducing waste and associated costs. By addressing inefficiencies in power delivery systems, organizations can save significantly on operational expenditures.
-
Enhanced Equipment Longevity: Monitoring helps identify overloading or inefficiencies in equipment like transformers, transmission lines, and power stations. Proactively addressing these issues extends the lifespan of critical infrastructure.
-
Minimized Downtime and Outages: By preventing energy losses caused by faults or inefficiencies, organizations reduce the likelihood of power disruptions, ensuring consistent operations and better service delivery.
-
Regulatory Compliance and Sustainability: Energy loss prevention helps organizations meet regulatory requirements by ensuring accurate monitoring and reporting of energy usage. It also supports sustainability goals by reducing unnecessary carbon emissions tied to wasted energy.
-
Increased Operational Transparency: Real-time energy monitoring provides actionable insights, allowing operators to make informed decisions. This transparency fosters trust and accountability across stakeholders.
-
Support for Renewable Energy Integration: Efficient energy transmission is crucial for incorporating renewable energy sources into grids, ensuring minimal losses and maintaining grid stability.
Technology Transforming of Energy Loss
Internet of Things (IoT): In AI systems, IoT sensors, which are used for data collection, feed real-time information on all measures of the transmission network.
Machine Learning & Deep Learning: This class of computer techniques is applied for data mining of big volumes of data in which these techniques find applications.
Edge Computing: With edge computing, AI agents need not send data to the cloud and rather enjoy localized data processing thereby enabling the faster realization of real-time decisions.
Cloud Computing: For effective implementations in such systems, cloud-based services are indispensable for processing and storing massive amounts of information.
The Future Trends of Energy Loss Detection and Prevention
-
Advanced Energy Monitoring Systems: Future systems will incorporate real-time and highly accurate monitoring technologies, enabling the continuous assessment of energy losses across complex transmission networks. These systems will utilize enhanced sensors and data analytics to identify inefficiencies faster.
-
Integration of Renewable Energy Sources: As renewable energy sources like solar and wind become more widespread, energy loss detection systems will evolve to manage fluctuations in energy supply and demand efficiently. This will ensure minimal transmission losses even in dynamic grid conditions.
-
Predictive Maintenance and Fault Detection: The transition from reactive to predictive maintenance will dominate the future. By analyzing historical performance data and environmental factors, organizations will preemptively address potential transmission losses before they escalate into critical issues.
-
Microgrid Expansion: The rise of microgrids will localize power generation and distribution, reducing transmission distances and energy losses. These localized systems will work in tandem with broader grids, ensuring efficiency and stability.
-
Dynamic Energy Distribution: Adaptive systems capable of redistributing power based on real-time demand will minimize overloading and underutilization of transmission lines, ensuring more efficient energy flow across networks.
-
Blockchain for Energy Transactions: Blockchain technology will facilitate transparent and efficient energy trading, enabling precise tracking of energy flows and losses across the transmission chain.
-
Regulatory and Policy Innovations: Governments will likely establish stricter guidelines for energy loss reporting and incentivize the adoption of technologies that minimize transmission losses, aligning with sustainability goals.
Conclusion: Energy Loss Detection and Prevention in Transmission
The utilization of AI agents in energy loss identification and prevention is transforming the management of electric power transmission systems. Hence, by resorting to AI, energy firms can curtail energy losses, boost productivity, and enhance the dependability of their transmission networks. With the advancement of technology, there is no end to the use of energy transmission systems that are smarter and more sustainable. Without a doubt, the future of energy is going to be AI-based as there is a way to have a better and greener energy grid.
-
Explore More About Customer Data Analytics with AI Teammates