Key Insights
Agentic AI-driven energy optimization is transforming the manufacturing sector by enhancing operational efficiency and reducing costs, with potential savings in energy expenses. By integrating AI agents, manufacturers can achieve real-time insights and predictive analytics, leading to more sustainable practices and compliance with environmental regulations. Embracing these technologies is crucial for a competitive and eco-conscious future in manufacturing.
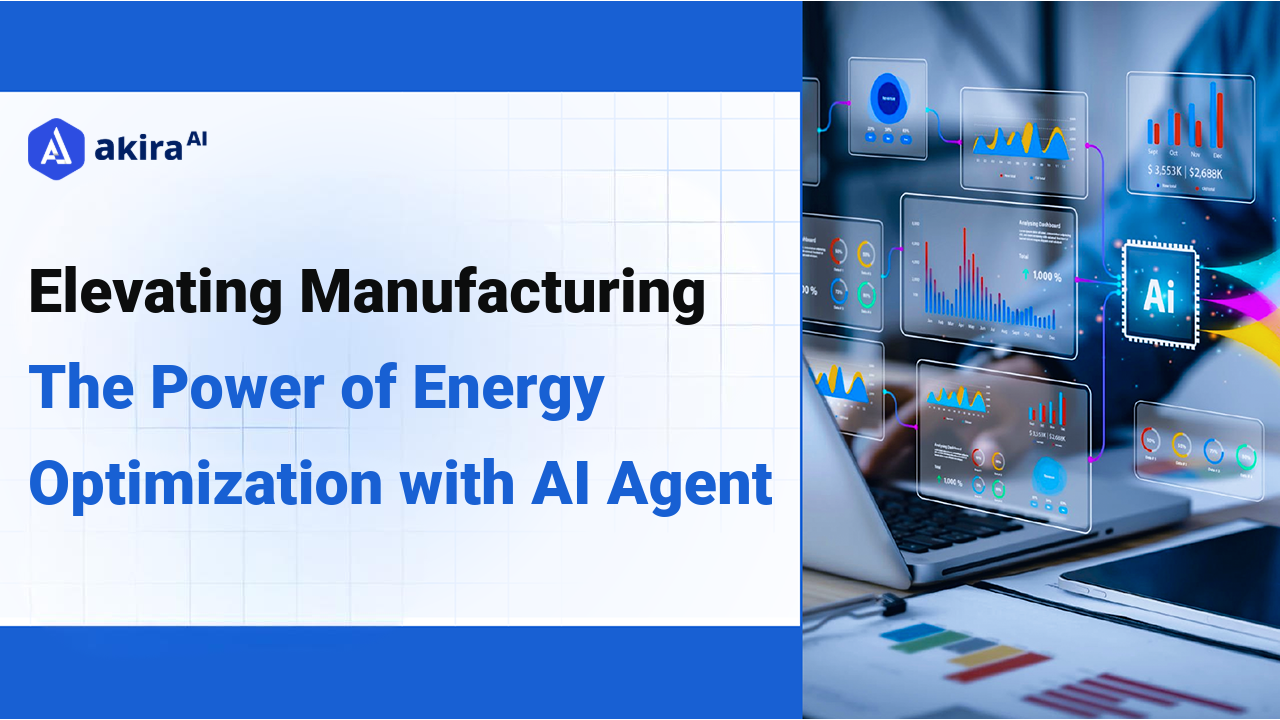
The clock is ticking for the manufacturing industry to embrace sustainability. In light of climate change, energy optimization is no longer a trend but has become a need. AI agents with large-scale applications offer tangible solutions where real manufacturers cut costs by saving extensive money and decreasing emissions. With countries adopting stringent laws on the discharge of greenhouse gases and the world consumer turning a new leaf on environmentally conscious practices, the need to get into these technologies has rarely been this important.
This blog addresses some of the ways the current agents are working towards creating a favorable and more sustainable future while giving a sneak peek at what the future might have in store. The time to act is now—let’s explore how embracing AI-driven strategies can revolutionize the manufacturing landscape and create a more sustainable world for generations to come.
What is Energy Optimization and Sustainability?
Energy management in manufacturing is the deliberate and planned enhancement of energy efficiency during production. This entails a detailed evaluation of energy usage and then taking action that reduces the required energy and its consumption while still guaranteeing efficiency and product quality. Effective energy optimization not only cuts costs but also minimizes the environmental footprint of manufacturing operations.
On the other hand, sustainability encompasses a broader vision that includes environmental stewardship, social responsibility, and economic viability. Sustainable manufacturing processes aim to reduce unwanted material outflow, efficiently utilize resources, and make the manufacturing processes more economical.
A Brief Overview of Energy Optimization and Sustainability
In the last few years, there has been growing concern for managing energy and sustainability issues owing to higher energy costs and issues related to legal compliance. Manufacturers are adopting various strategies to enhance energy efficiency, such as implementing energy-efficient technologies, optimizing production schedules, and utilizing renewable energy sources. These practices not only help in reducing operational costs but also contribute to a smaller carbon footprint.
The introduction of AI agents is a game changer in this domain. These intelligent systems are designed to analyze vast datasets, identify inefficiencies, and suggest improvements. They can dynamically adjust operations to optimize energy use by leveraging machine learning and predictive analytics.
Traditional vs. Agentic AI-Based Energy Optimization and Sustainability
Aspect |
Traditional Energy Optimization and Sustainability |
Agentic AI Energy Optimization and Sustainability |
Data Processing |
Manual analysis, often slow and error-prone |
Real-time analysis using machine learning algorithms |
Decision-Making |
Reactive, based on historical data |
Proactive, predictive decision-making |
Efficiency |
Limited optimization potential |
Continuous improvement through autonomous adjustments |
Scalability |
Difficult to scale across operations |
Easily scalable using multi-agent systems |
Integration |
Often siloed systems |
Seamless integration into existing workflows |
Cost Savings |
Gradual improvements in energy costs |
Significant cost reductions through optimized operations |
Akira AI Multi-Agent in Action
To illustrate the transformative power of agentic AI in manufacturing, we can examine the Akira AI multi-agent system. This multi-agent framework showcases how various autonomous agents work in tandem to optimize energy consumption across different manufacturing stages.
-
Data Collection Agent: This agent collects detailed energy consumption information of machinery, sensors, and other systems in the production line. It constantly avails real-time energy data which is important for analysis. This results in increased insight accuracy and enhances efficient decision-making when it is required.
-
Analysis Agent: The analysis agent processes the collected data to identify trends and inefficiencies. Using advanced machine learning techniques can uncover patterns that might not be evident through traditional analysis methods. This agent also benchmarks energy use against industry standards, helping manufacturers gauge their performance.
-
Optimization Agent: Employing the information received from the analysis agent, the optimization agent gives recommendations on how energy consumption can be minimized. It entails modifying the speed and other parameters of a production line; altering a schedule of operations; or redeploying assets. Such a situation may enable the agent to model different situations so that it can identify the best course of action to take regarding energy conservation.
-
Monitoring Agent: This agent tracks the implementation of the suggested changes in real-time, ensuring that energy optimization strategies are effectively executed. It provides feedback and alerts operators about deviations from expected energy performance, allowing for prompt corrective actions.
Use Cases and Application of Energy Optimization and Sustainability
-
Predictive Maintenance: AI agents can predict equipment failures before they occur, minimizing downtime and energy waste. By analyzing historical data and real-time sensor inputs, these agents can identify warning signs of potential malfunctions. This proactive approach allows manufacturers to perform maintenance activities only when necessary, optimizing both energy use and operational efficiency.
-
Smart Grid Integration: The multi-agent system expands the interaction between manufacturing facilities and smart grids, thereby improving the energy consumption ratio by considering the demand and supply status. Manufacturers might shield themselves from high energy prices if they are able to predict when energy consumption is likely to be at its apex and vice versa by optimizing operations to rely on renewable sources of power.
-
Process Automation: AI-driven automation helps streamline production processes, reducing energy consumption while enhancing productivity. Intelligent systems can adjust machinery operations based on real-time data, ensuring that energy is used efficiently throughout the production cycle. This not only leads to cost savings but also minimizes waste and emissions.
-
Renewable Energy Management: AI agents can effectively manage the integration of renewable energy sources, ensuring optimal utilization and storage. For instance, such agents can forecast the output of energy from solar panels or windmills hence enabling manufacturers to optimize energy consumption by consuming most of the green energy.
-
Supply Chain Optimization: The multi-agent system analyzes logistics and supply chain data to suggest energy-efficient routes and practices. By optimizing transportation and distribution processes, manufacturers can significantly reduce their carbon footprint and energy consumption. This holistic approach ensures that sustainability is considered at every stage of the supply chain.
Operational Benefits of Energy Optimization and Sustainability
-
Enhanced Efficiency: These agents improve operational efficiency by optimizing resource allocation and minimizing energy waste. By continuously monitoring and adjusting processes, manufacturers can achieve higher production rates with lower energy inputs
-
Cost Savings: Implementing agentic AI systems can reduce energy costs by up to 30%. According to a study, manufacturers adopting AI-driven strategies have reported significant decreases in their energy expenses, enabling them to reinvest those savings into other operational improvements.
-
Increased Productivity: Autonomous agents can drive productivity improvements by up to 30% through process optimization. By automating routine tasks and providing real-time insights, these agents enable workers to focus on higher-value activities, resulting in overall productivity gains.
-
Reduced Waste: Agentic AI identifies inefficiencies that lead to waste, contributing to more sustainable practices. By pinpointing areas where energy is being squandered, manufacturers can implement corrective measures that not only save energy but also reduce raw material consumption and waste generation.
Technologies Transforming in Energy Optimization and Sustainability
-
Machine Learning Algorithms: These algorithms enable these agents to analyze vast datasets for real-time insights. By learning from historical data and adapting to new information, machine learning enhances the accuracy of predictions and recommendations, leading to better decision-making.
-
IoT Integration: The Internet of Things (IoT) connects devices and sensors throughout manufacturing, gathering valuable data for analysis. By integrating IoT with AI, manufacturers can achieve deeper insights into energy consumption patterns and optimize processes accordingly.
-
Blockchain Technology: Using blockchain in supply chains simplifies the tracking of energy usage and sustainability measures. Due to this, blockchain is known to create transparency regarding energy consumption and sourcing and provide accountability for sustainability.
-
Cloud Computing: Cloud systems allow autonomous agents to store and access data if necessary, making it possible for manufacturers to benefit from big data without installing extensive infrastructure on the sites. This makes it easy to develop sustainable solutions for the business's growth in the future.
-
Digital Twins: Simulation allows manufacturing firms to virtually model new changes before physically implementing them. This increases the efficiency of decisions within an organization and the level of exposure to modifying operating processes.
The Future of AI Agents for Energy Optimization and Sustainability
-
Widespread Adoption: With time, autonomous agents are expected to drive most of the manufacturing work. As more companies recognize the value of AI in enhancing energy efficiency and sustainability, adopting these technologies will continue to grow.
-
Greater Integration: Enhanced integration of agentic AI into all manufacturing processes will enable more cohesive and efficient operations. With time, it will become an integral part of manufacturing systems, enhancing inter-departmental cooperation and, thus, efficiency.
-
Advanced Analytics: The future will see improved predictive analytics, allowing manufacturers to anticipate energy needs more accurately. Enhanced analytical capabilities will lead to more informed decision-making and further optimization of energy use.
-
Collaborative AI Systems: The development of multi-agent workflows will enable different agents to work together to tackle complex problems. By leveraging the strengths of various agents, manufacturers can achieve superior outcomes in energy optimization and sustainability.
-
Focus on Circular Economy: AI will be critical in promoting circular manufacturing practices, where waste is minimized, and resources are reused. By optimizing processes and supply chains, AI can support manufacturers in transitioning to more sustainable business models.
Conclusion: AI Agents for Energy Optimization and Sustainability
The intersection of AI agents, energy optimization, and sustainability is changing the manufacturing sector by leaps and bounds. By harnessing the power of agentic AI, manufacturers can achieve unprecedented levels of efficiency, reduce costs, and minimize their environmental impact. As the industry moves toward more sustainable practices, the role of autonomous agents will become increasingly crucial, driving innovation and ensuring a viable future for manufacturing. Embracing these technologies is not just a choice; it’s necessary for those committed to sustainability and operational excellence. The continued evolution of AI will shape the future of manufacturing, paving the way for smarter, greener, and more efficient production processes.