Key Insights
-
AI agents optimize energy transmission in real-time, reducing energy waste, lowering costs, and boosting overall system reliability.
-
By identifying inefficiencies and potential failures early, AI agents ensure timely maintenance, preventing energy loss and minimizing disruptions.
-
AI helps balance energy supply and demand, ensuring efficient distribution and supporting a more sustainable, reliable energy future.
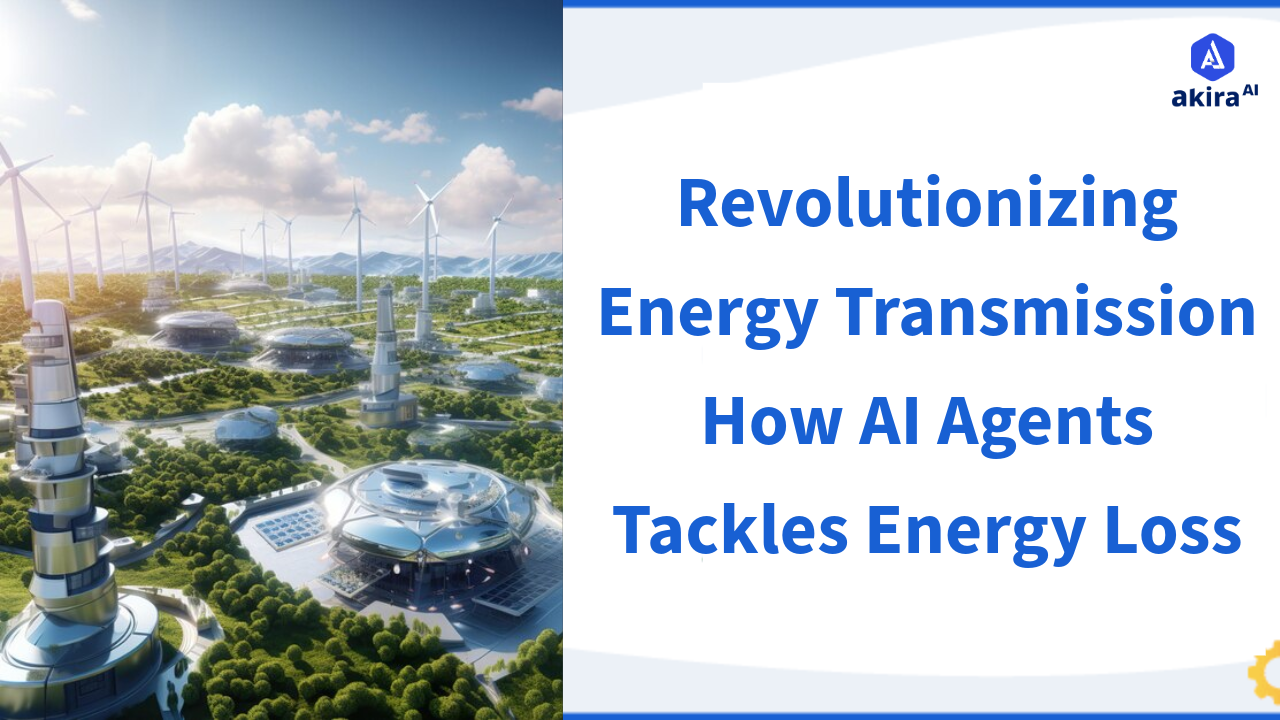
A utility company facing rising operational costs and frequent power outages due to energy loss in transmission realized it needed a new approach. Traditional monitoring and control methods were no longer enough to keep up with the growing energy demand and maintain efficiency. The company turned to artificial intelligence (AI), deploying AI agents to optimize its transmission network in real-time.
The results were remarkable: energy waste was reduced, costs were cut, and system reliability improved. This transformation not only enhanced the company's bottom line but also demonstrated the power of AI Teammates in creating a more innovative, sustainable energy future. This blog will explore how telecom revolutionises energy loss detection and prevention in transmission systems.
What is Energy Loss Detection and Prevention in Transmission?
Energy loss detection and prevention in transmission refers to identifying and mitigating the loss of electrical energy that occurs as power is transmitted from generation sources to end users. This energy loss can occur due to various factors, such as resistance in transmission lines, inefficiencies in transformers, and faults in the grid infrastructure.
It aims to monitor and optimize the transmission network, ensuring energy is efficiently delivered while minimizing waste. Effective detection and prevention strategies help reduce operational costs, enhance system reliability, and promote sustainability in energy distribution.
Key Concepts of Energy Loss Detection and Prevention
Energy Loss Types: Understanding technical losses (e.g., resistance in transmission lines and transformers) and non-technical losses (e.g., theft or faulty metering) is essential for effectively managing energy waste.
Real-Time Monitoring: Continuous monitoring of the transmission network enables the rapid detection of inefficiencies, faults, or abnormalities, allowing quick corrective actions to minimize energy loss.
Anomaly Detection: Identifying unusual patterns or behaviours in the system, such as unexpected voltage drops or overheating components, helps prevent energy loss before it becomes a major issue.
Predictive Maintenance: Using data from the transmission system to predict potential equipment failures or inefficiencies, allowing for timely maintenance and preventing unexpected energy losses.
Grid Optimization: Adjusting the electricity distribution based on real-time demand and supply conditions to optimize the energy flow, reduce losses, and ensure efficient energy delivery.
Traditional Way of Detecting and Preventing Energy Loss
Historically, transmission systems' energy loss detection and prevention were manual and reactive. Operators relied on periodic inspections and manual readings of devices, providing limited data and only detecting energy loss after it occurred. This approach often missed emerging issues between inspections, making it challenging to address inefficiencies promptly.
Maintenance was scheduled at fixed intervals, leading to unnecessary repairs or delayed fixes, which could cause unplanned downtimes. Without real-time monitoring or predictive tools, energy distribution couldn't be optimized dynamically, resulting in higher energy losses and increased operational costs.
Impact on Customers Due to Traditional Ways of Energy Loss Detection
The traditional methods of energy loss detection led to several issues:
-
Inefficient Energy Use: Without the ability to monitor and optimize energy use in real-time, transmission systems often run inefficiently, wasting energy.
-
Higher Operational Costs: Manual inspections, scheduled repairs, and reactive maintenance contributed to higher utility costs, affecting consumers.
-
Unreliable Power Supply: Lack of real-time monitoring often leads to unplanned outages and power failures, which inconvenience customers and reduce system reliability.
As a result, customers experienced more frequent and prolonged outages, higher energy bills, and an overall decline in service quality.
Akira AI: Multi-Agent in Action
As an agent for identifying and mitigating energy losses, Akira AI is a complex activity involving many levels of operations for optimal results. This is how these agents function in the field:
-
Data Collection by Sensors: IoT sensors installed across the transmission network continuously collect data such as voltage, current, and temperature. These sensors send real-time data to the monitoring system, providing detailed insights into the grid’s operation.
-
Data Analysis by Monitoring Agents: Specialized monitoring agents analyze the incoming data to identify patterns, anomalies, or inconsistencies that may indicate energy loss. These agents process large volumes of data quickly to detect issues in real-time.
-
Anomaly Detection by Detection Agents: Detection agents identify unusual behaviours, such as sudden voltage drops or overheating, which could signal potential faults or inefficiencies. These agents can alert operators immediately to address problems before they escalate.
-
Predictive Maintenance by Maintenance Agents: Maintenance agents use historical data and predictive analytics to forecast potential failures or inefficiencies. Based on these predictions, maintenance actions are scheduled proactively, preventing unexpected breakdowns and reducing energy loss.
-
Energy Flow Optimization by Optimization Agents: Optimization agents adjust the energy distribution in real-time, balancing supply and demand to minimize energy wastage. These agents dynamically optimize the grid to ensure efficient energy flow, reducing losses and improving overall system performance.
By integrating these agents into the energy transmission process, companies can significantly reduce operational costs and improve energy efficiency, leading to a more sustainable and reliable system.
Prominent Technologies in the Space of Energy Loss Detection
Several key technologies have influenced the field of energy loss detection:
-
Internet of Things (IoT): IoT sensors collect real-time data from various components of the transmission network, providing crucial information about energy usage, voltage, and temperature.
-
Edge Computing: Edge computing processes data locally rather than sending it to the cloud, reducing latency and enabling real-time decision-making at the transmission level.
-
Cloud Computing: Cloud-based services store and analyze vast amounts of data, making it easier for AI systems to identify patterns and predict potential energy losses.
-
Advanced Metering Infrastructure (AMI): AMI systems monitor energy consumption and provide utilities with detailed insights into how and where energy is lost across the transmission network.
AI agents enable real-time bandwidth management, enhancing network performance and reducing congestion for seamless telecom service delivery.
Successful Implementations in Energy Loss Detection and Prevention
-
Smart Grids: PG&E in California uses smart grid technology to monitor and adjust energy flow in real-time based on demand and weather patterns. This helps reduce transmission losses and ensures efficient energy distribution across the grid.
-
Predictive Maintenance for Transformers: Siemens and Con Edison use predictive maintenance tools to monitor transformer health continuously. By detecting potential failures early, they can schedule maintenance proactively, reducing downtime and costly outages.
-
Grid Fault Detection: E.ON in Germany employs advanced systems to detect faults like power line breaks or short circuits. These systems alert operators immediately, enabling faster repairs and preventing extended energy loss or outages.
-
Intelligent Energy Management Systems: Ørsted in Denmark optimizes wind power integration into the grid, adjusting energy inflow based on demand. This helps match renewable energy production with real-time consumption, minimizing waste and improving system efficiency.
How AI Agents Supersede Other Technologies
-
Enhanced Predictive Capabilities: Predictive models will become more accurate as algorithms advance, enabling systems to anticipate potential energy losses or system failures. This allows for proactive measures that address inefficiencies before they occur.
-
Integration with Smart Grids: Real-time dynamic optimization of energy distribution based on actual demand and supply will become more seamless. Smart grids, supported by AI-driven systems, will reduce transmission losses and improve grid reliability.
-
Advanced Anomaly Detection: AI will enable the detection of apparent issues and subtle patterns that indicate early-stage problems. This improvement will lead to quicker, more accurate interventions to minimize energy losses.
-
Decentralized Energy Management: As renewable energy sources grow, AI-powered systems will help optimize the integration of renewable power into decentralized energy grids, ensuring efficient distribution and storage despite fluctuating generation.
-
Self-Learning Systems: Future energy systems will leverage self-learning AI agents that continuously improve based on historical and real-time data. These systems will optimize energy flow, reduce losses, and require minimal human intervention.
These trends indicate the continued evolution of energy transmission systems, with AI playing a key role in driving smarter, more efficient, and sustainable solutions.
Redefining Energy Loss Management with AI Agents
Implementing AI agents in energy loss detection and prevention transforms energy transmission systems. By leveraging AI, utilities can reduce energy waste, lower operational costs, and enhance the reliability and efficiency of the transmission network. With continued advancements in AI technologies, the future of energy transmission looks set to become more intelligent, efficient, and sustainable. AI agents help detect and prevent energy loss and optimize the entire energy distribution process, paving the way for more innovative, greener energy grids.
Explore More About Customer Data Analytics with AI Teammates
Next Steps in Telecom
Talk to our experts about implementing compound AI system, How Industries and different departments use Agentic Workflows and Decision Intelligence to Become Decision Centric. Utilizes AI to automate and optimize IT support and operations, improving efficiency and responsiveness.