Key Insights
With Agentic AI, insurers are no longer bound by the limitations of traditional risk assessment models. These intelligent agents leverage machine learning algorithms to analyze vast datasets that include both structured data, like client history and claims information, and unstructured data, such as social media activity or even weather patterns. AI agents are capable of rapidly processing and interpreting these data points to uncover correlations and risk indicators that might have previously gone unnoticed.
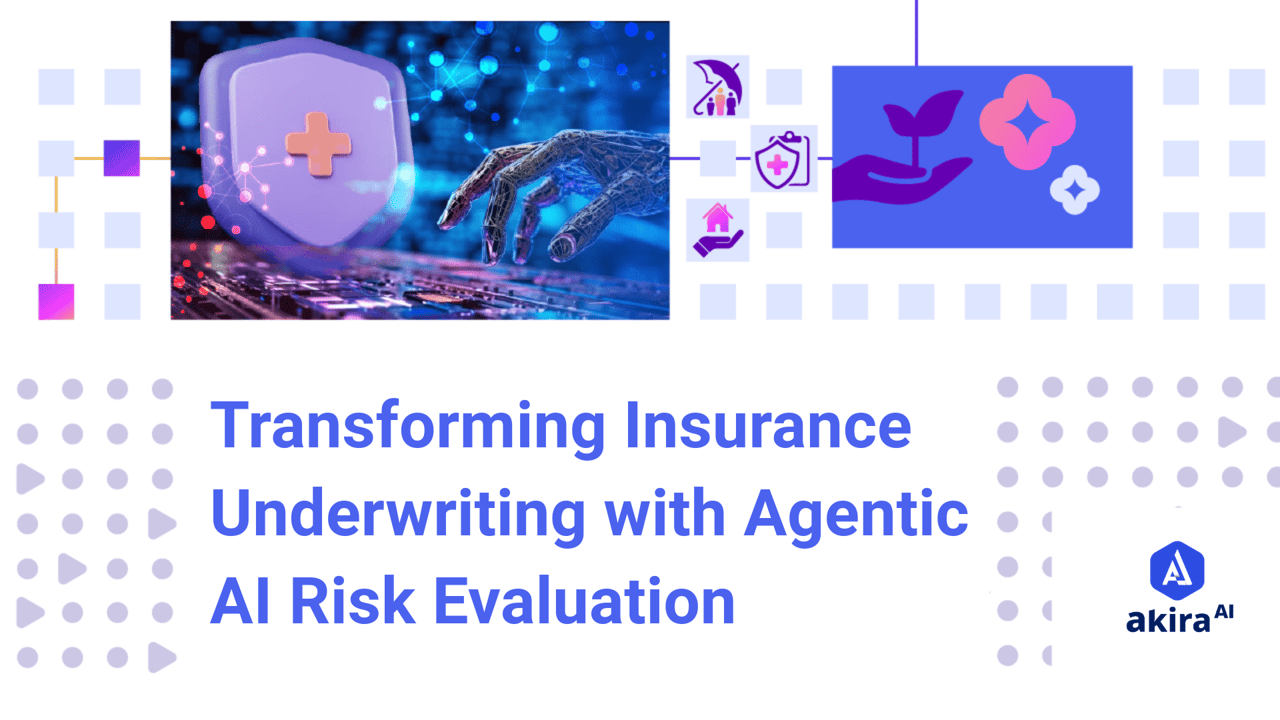
"What if insurance companies could predict the future?" While predicting the future is still a stretch, AI agents are bringing insurers much closer to understanding and predicting risk with remarkable accuracy. Insurance underwriting has always been a complicated process and comes with several risks. AI agents for risk assessment counter this by processing large amounts of data—such as historical claims, environmental data, and social media—faster and more precisely than traditional methods ever could. This enables insurers to assess risk much deeper, making smarter underwriting decisions. In this blog, we’ll explore how these agents are changing the game in the insurance sector and underwriting, making the entire process faster, more accurate, and more adaptable to today’s dynamic world.
What is Risk Assessment in Insurance Underwriting?
Risk assessment in insurance underwriting evaluates potential risks associated with insuring a person, asset, or business. It involves gathering and analyzing relevant data, such as the applicant's history, the type of coverage requested, and external factors like environmental or market conditions. The assessment aims to determine the likelihood of a claim occurring and set appropriate policy terms and premiums. A risk assessment tool is used to calculate and predict the risks, which is critical for insurers to offer competitive and sustainable policies.
A Brief Overview of Risk Assessment in Insurance Underwriting
Risk assessment in insurance underwriting has traditionally involved manual processes where underwriters assess the risk factors of an applicant based on predefined criteria and available data. However, these processes are normally time-consuming, highly manual, and have a tendency to be prone to errors. In the face of a growing volume of data, high speed and high accuracy of decision-making are suggested by the advanced application of AI in risk evaluation. These agents can run through large data sets and notice patterns that would not be noticed in a normal search, making insurance risk assessment even more accurate.
Autonomous agents are reshaping the risk assessment process by automating key functions, making them more efficient and scalable. These agents are trained using machine learning algorithms and can process vast amounts of structured and unstructured data, such as claims history, customer demographics, market trends, and environmental data. AI-enabled risk assessment tools help insurance companies better predict risk, streamline workflows, and improve decision-making. By integrating AI-powered insurance underwriting, insurers can offer more personalized policies and optimize risk management in real-time.
Traditional vs. Agentic AI Risk Assessment in Insurance Underwriting
Aspect |
Traditional Risk Assessment |
Agentic AI-Based Risk Assessment |
Data Processing |
It relies on historical data and manual analysis |
Utilizes machine learning to process vast datasets |
Accuracy |
Limited by human expertise and biases |
Highly accurate with minimal human intervention |
Speed |
Slow due to manual data entry and analysis |
Fast, with real-time analysis and automated workflows |
Scalability |
Not easily scalable without increasing resources |
Easily scalable with AI agents handling large volumes |
Personalization |
One-size-fits-all approach |
Highly personalized policies based on individual risk |
Cost Efficiency |
High due to manual labor and human errors |
Reduced operational costs with automated processes |
Akira AI Multi-agent in Action
Akira AI is a cutting-edge solution in AI-enabled risk assessment, which helps deal with insurance underwriting and risk assessment challenges through the multi-agent system. In this system, every sub-agent is coordinated by a master orchestrator, and each plays some role in the risk assessment process.
Master Orchestrator: The master orchestrator is the node that controls all the agents and through which all the communication is done. This agent makes certain that information is well transmitted between two or more agents and that decisions are made on the premise of the information provided
Key Agents Used by Akira AI:
-
Data Collection Agent: This agent compiles both structured and unstructured data from targets such as the customers’ database, the history of claims, social media, and market reports.
-
Risk Evaluation Agent: This AI agent uses advanced machine learning algorithms to analyze the data and assess the level of risk associated with the applicant.
-
Fraud Detection Agent: This agent utilizes supervised and unsupervised learning models to recognize the suspicion of fraudulent claims or applications.
-
Pricing Agent: Based on the risk evaluation, this agent determines the optimal pricing for a given policy, considering market conditions, competitor pricing, and customer behavior.
-
Decision-Making Agent: This agent makes the final underwriting decision based on the combined analysis of all agents. It can approve, deny, or recommend additional scrutiny for the application.
-
Compliance Agent: This agent ensures that all underwriting decisions adhere to legal and regulatory requirements.
Application in Risk Assessment for Insurance Underwriting
-
Life Insurance: AI agents take in basic biographical data and other details of an applicant, along with family health histories, to provide relevant life insurance policies at different but more appropriate premiums based on individual health states.
-
Auto Insurance: AI systems monitor the interaction of drivers with vehicles, vehicles’ history, and traffic to assess the likelihood of a particular driver causing an accident and provide insurance at individual real-time driving prices.
-
Cyber Insurance: Implementing these agents to evaluate a company’s risk factors by measuring its infrastructure, threats, and past attacks as input helps insurers target relevant coverages per cyber danger.
-
Property Insurance: These agents evaluate the risk of insuring a property by considering location, weather conditions, and building integrity, helping insurers offer more accurate pricing and better coverage based on environmental and structural factors.
-
Health Insurance: AI-enabled tools calculate the probability of developing a disease with the help of data such as age, medical history, and lifestyle and provide accurate premiums and coverage.
-
Travel Insurance: Agentic AI evaluates risk factors like destination, health conditions, travel history, and geopolitical events, enabling insurers to provide more personalized travel insurance policies with tailored risk mitigation strategies.
Operational Benefits of Risk Assessment
Increased Operational Efficiency: These agents can work on large amounts of data and process it in real-time, which has brought underwriting times down by a third. It leads to quicker policy issuance and happy customers and therefore organizations that want to thrive should consider implementing the solution.
Cost Reduction and Savings: AI-powered underwriting can reduce operational costs by up to 30%. Automation minimizes manual work; hence, there is less of a chance of making mistakes when it comes to the usage of resources.
Enhanced Accuracy in Risk Evaluation: AI systems are more accurate than traditional methods, leading to better risk evaluations and reduced underwriting errors. This can reduce loss ratios by 15%.
Seamless Scalability: As these agents can process a large number of applications simultaneously, insurers can scale operations without the need for additional human resources. This is particularly useful in handling peak times.
Superior Risk Prediction: The systems can use historical data and real-time information to predict potential risks more accurately, minimizing exposure to high-risk clients and ensuring profitability.
Technologies Transforming Risk Assessment in Insurance Underwriting
-
Machine Learning (ML) for Continuous Improvement: ML enables AI agents to learn from past data, improving their decision-making capabilities over time.
-
Natural Language Processing (NLP) for Data Understanding: NLP helps AI systems understand and process unstructured data, such as customer feedback, medical records, and claim descriptions.
-
Predictive Analytics for Proactive Risk Management: These analytics explore data based on standardized measures to help insurers create more realistic policies regarding future risks.
-
Blockchain for Secure and Transparent Transactions: Ensures the security and transparency of transactions between AI agents, particularly in sensitive insurance dealings.
-
IoT (Internet of Things) for Real-Time Risk Assessment: IoT collects real-time data from devices like smart home systems, wearables, and cars to assess ongoing risks dynamically.
The Future of Risk Assessment in Insurance Underwriting
-
Automation Powered by AI: Underwriting automation will evolve progressively as more specific AI technologies are incorporated into underwriting processes to reduce the time taken to arrive at risk assessment and increase organizational efficiency.
-
Customized Insurance Solutions: The implementation of agentic AI will make it possible to create insurance policies that will suit every policyholder, depending on their risk factors.
-
Enhanced Fraud Prevention: AI's growing role in risk assessment will help detect and prevent fraud more effectively by identifying unusual patterns and flagging potential fraudulent claims with higher accuracy.
-
Improved Customer Interactions: The significant improvement of underwriting procedures through artificial intelligence will cause better client experiences as underwriting processes will be faster and more suitable.
-
Dynamic Risk Analysis: Real-time data processing through AI will allow insurers to assess and adjust risk levels in real-time, enabling them to respond to emerging risks faster and more accurately.
-
Predictive Insights for Better Decisions: AI's ability to analyze historical data will enable predictive analytics to forecast future risks, helping insurers make smarter, forward-looking underwriting decisions.
Conclusion: AI Agents for Risk Assessment in Insurance Underwriting
Adopting AI-powered insurance underwriting is no longer a matter of if—it’s a matter of when. As the demand for faster, more accurate, and cost-efficient risk assessment grows, AI agents are positioned to revolutionize the underwriting process. By automating complex data analysis, these intelligent agents speed up decision-making and enhance accuracy, reducing the risk of human error and improving customer satisfaction. With AI in risk evaluation, insurers can process more applications with fewer resources, resulting in lower operational costs and better scalability. As this technology continues to mature, it will become an even more integral part of risk management in insurance, driving innovation, increasing efficiency, and ultimately delivering more personalized and competitive offerings to policyholders. The future of underwriting is undoubtedly AI-driven.