Key Insights
Effective AI prompting boosts clarity, precision, and context, leading to better outcomes across multiple fields. Techniques like Chain-of-Thought, Few-Shot, and Zero-Shot learning enhance AI adaptability, problem-solving, and efficiency. As prompting methods evolve, they enable more personalized, context-aware, and multimodal interactions, improving user experience and AI performance.
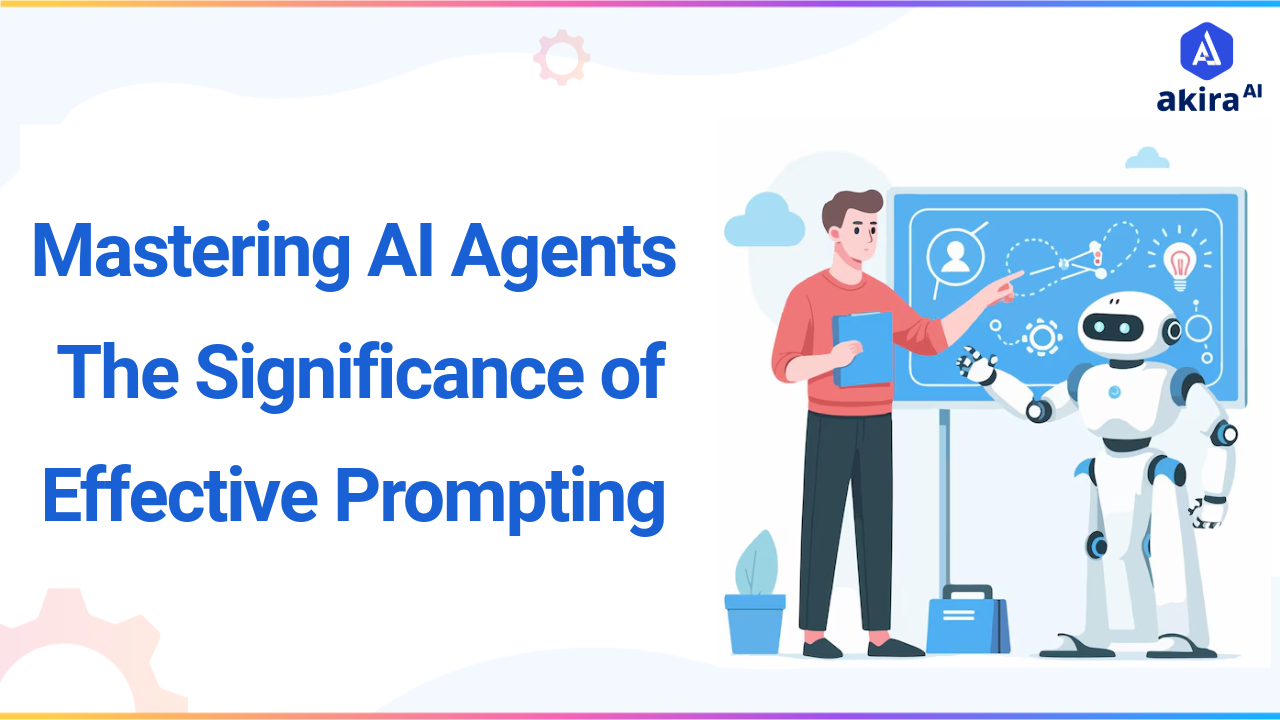
Mastering the art of effective prompting has never been more crucial. This blog delves into the significance of AI prompting across various fields, highlighting its potential to revolutionize interactions and drive global business development. Whether you’re a developer crafting complex applications, a content creator weaving engaging narratives, or a business professional analyzing trends and data, the precision of your prompts can dramatically influence outcomes.
As Agentic AI becomes integral to diverse sectors, understanding how to leverage different prompting techniques will empower you to maximize the capabilities of these tools. In this blog, we will explore the fundamental principles of effective prompting, practical strategies for implementation, and the transformative impact it can have on your work.
What is Prompting?
Prompting is the art and science of crafting inputs that guide AI models to produce desired outputs. Think of it as having a conversation with a highly capable assistant – the clarity and structure of your requests directly influence the quality of responses you receive.
How prompting is effective for AI Agents?
Prompting significantly enhances the effectiveness of AI agents by providing clear direction, context, quality control, efficiency, and consistency. Clear prompts direct the AI as a map does, for example, a request for an article of 300 words analyzing trends in the retail market in 2024. In the neat west, it enables the AI to understand the spirit of a message. For instance, this is a reply from the viewpoint of a financial advisor when addressing a first-time investor. Specific requirements enhance the quality of the output, while good prompts make expected responses less reactionary and quicker. Last, clear prompts keep attention on certain assignments and guarantee that all specifications are fulfilled in case of a lengthy exercise.
The Anatomy of an Effective Prompt
Creating effective prompts is essential for optimizing AI interactions, and it involves several key components:
1. Clarity and Specificity
1.1 Be precise: Do not just say things like “write about dogs”; instead, tell your writer exactly what you expect of him or her, such as “write an article of no less than 500 words on the training needs of German Shepherds.” This procedure clarifies the content pertinent to the instant requirement, thus helping the AI do the work.
1.2 Set Parameters: It is important to state your requirements for the format, the length of the output, and its tone and style. Doing so helps the AI better meet your expectations.
1.3 Provide Context: Adding concern context and providing additional information gives AI the rules for answering the question.
2. Structure and Format
2.1 Use Clear Headers: Break up complex instructions with sections and headers where possible. This makes it easier for the AI to comprehend what you want done and makes it more coherent.
2.2 Break Down Tasks: When making complex instructions, enumeration of the procedures or the parts also helps the AI in that none of such parts may be left out.
2.3 Include Examples: Forcing examples of desired outputs can also help solve misunderstandings and serve as a guide for the AI, enhancing the quality of the outcomes produced.
3. Context Control
3.1 Role Definition: Identify the viewpoint or the level of proficiency required in the output. For example, when a question is posed, phrasing the question in such a way as to the request for content from the viewpoint of ‘a veterinary expert’ forces the AI to respond to the query from that perspective.
3.2 Audience Specification: The AI can determine its audience, or who the output is for, whether beginners, professionals, or everyone in between, thus helping it choose how complex or simple the content should be.
3.3 Purpose Clarification: To elaborate on the effect defined by the type of output, such as a blog post, a presentation, or educational material, it’s useful to explain how it will be used, which also improves relevance and efficiency.
Advanced Prompting Techniques
1. Chain-of-Thought Prompting
Description: The chain-of-thought prompting technique helps AI consider the creation of a task as a sequence of logically connected actions. This approach copies how human beings approach problems by decomposing them into parts for solutions.
Key Points:
1.1 Sequential Reasoning: Facilitates structured working of an AI system.
1.2 Clarity: Includes each aspect of the problem to avoid ambiguity.
1.3 Thoroughness: This approach covers all areas of the problem, seeking to diagnose and offer workable solutions to every aspect.
1.4 Problem-solving: Systematically deals with complicated problems, thus copying human thought processes.
Instead of: "Calculate the profit margin"
Use: "Let's solve this step by step:
1. First, identify the total revenue
2. Then, calculate total costs
3. Finally, determine the profit margin using the formula..."
2. Few-Shot Learning
Description: A unique form of learning known as Few-Shot Learning is used with AI, where the machine is shown examples of how to answer certain questions. This approach enables the AI to learn from a few instances of a given pattern and apply the learnt data to similar diversified circumstances. By giving input-output pairs, users assist the AI in understanding what answer they provide.
Key Points:
2.1 Generalization: This enables the AI to use examples from a few samples in other instances it has not encountered before.
2.2 Contextual Understanding: This helps the AI understand the response tone and writing style expected of her/him.
2.3 Efficiency: Cuts the robust data requirement using only taming instances or a few.
2.4 Flexibility: This enables suitable alteration of the responses depending on several contexts and formats demonstrated in the examples.
Input: "Sunny day"
Output: "The golden sun bathes the landscape in warmth"
Input: "Stormy night"
Output: "Thunder crashes as rain pelts the windows"
Now write for: "Misty morning"
-
Zero-Shot Prompting
Description: Zero-shot prompting is a technique that enables users to request specific outputs from the AI without providing prior examples. Instead of relying on sample inputs to guide its responses, the AI uses clear, direct instructions to understand what is being asked.
Key Points:
3.1 Direct Instructions: Encourages clear and explicit prompts to shape the AI’s output effectively.
3.2 Independence: Functions without needing examples, making it adaptable to various requests.
3.3 Utilization of Knowledge: Relies on the AI’s extensive pre-existing knowledge to produce relevant and accurate responses.
3.4 Adaptability: This enables the AI to handle a variety of queries, showcasing its flexibility in generating responses without prior training on those specific requests.
"Write a professional email declining a job offer while maintaining good relationships"
Unlocking the Potential of AI Agents Through Strategic Prompting
-
Clarity in Communication: Well-structured prompts break down complex instructions, making them easier for systems to understand and execute precisely. This ensures that even intricate tasks are carried out accurately.
-
Adaptability: Strategic prompting allows systems to interpret context and adjust responses dynamically. This fosters continuous learning, enabling better handling of evolving requirements or challenges.
-
Broader Applications: Thoughtful prompting expands the range of topics a system can address effectively, making it versatile across diverse industries and use cases.
-
Enhanced User Experience: Personalizing prompts improves interaction quality, aligning outputs with user needs and preferences and creating more meaningful and engaging experiences.
-
Future-Ready Solutions: By refining how tasks are conveyed, systems remain effective in rapidly changing environments, ensuring long-term relevance and utility.
Seamless Integration with Akira AI
Centralized Hub for Prompts: Akira AI provides a unified platform with a diverse library of prompts, streamlining interactions and enhancing task execution across multiple AI agents.
Empowers AI Capabilities: The robust prompt library enables users to unlock the full potential of AI agents, guiding them through structured processes for efficient communication and workflow execution.
Facilitates Collaboration: Integration with Akira AI fosters collaboration among AI agents, allowing them to share insights and leverage each other’s strengths for improved performance.
Enhances User Experience: By offering tailored prompts and a cohesive ecosystem, Akira AI ensures users can confidently navigate tasks with greater ease and effectiveness.
Transforms AI Interaction Landscape: Akira AI redefines AI functionality, creating a dynamic, interconnected hub that elevates agent performance and enriches user engagement.
Exploring the Key Benefits of Prompting Techniques for AI Agents
-
Enhances AI Competency and User Interaction: Useful prompts significantly improve the skill levels of AI agents, making user interactions more seamless and intuitive.
-
Improves Query Comprehension and Accuracy: Structured reasoning enables AI agents to understand queries better and provide more accurate, relevant responses.
-
Facilitates Learning and Adaptability: Prompts allow AI agents to learn from examples, adapt to new tasks, and meet users' diverse needs effectively.
-
Enriches Interaction Quality: Prompting techniques create more natural and meaningful conversations, enhancing the overall communication experience.
-
Boosts Efficiency and Client Satisfaction: By effectively improving efficiency and handling unexpected requests, AI agents contribute to higher satisfaction levels and brighter prospects for future communication advancements.
Discover how Relational RAG integrates structured databases with AI, enabling intuitive, precise, and real-time data retrieval.
Future Trends in Prompting Techniques for AI Agents
As AI technology advances, prompting techniques for AI agents are expected to evolve in several exciting ways. Here are some anticipated future trends:
-
Increased Personalization: Subsequent prompting techniques are expected to produce multiple natural language interactions based on users’ data. Agents will also be programmed to retrieve information about individual users, their interests, actions, and previous engagements, making the experience much more interactive.
-
Context-Aware Interactions: Thanks to improvements in NLP, contextual understanding will be even more pronounced. People will rely on contextual cues from dialogues to give better and finer answers so that the conversation seems natural.
-
Integration of Multimodal Inputs: The future of prompting will require consolidating different inputs, including text, voice, and visual. This will help AI agents gather and parse the necessary data to perform query processing more efficiently when dealing with various forms of information.
-
Improved Learning Efficiency: Sub-techniques like Few-Shot and Zero-Shot Learning will mature, allowing the AI agent to be trained with fewer examples while yielding higher performance. This will also improve their learning, enabling them to use their skills for other new tasks and in an environment without much training.
Conclusion: AI Agents for Prompting
Developing prompting orthodoxies with AI-specialized representatives will result in a radical change in user-enacted interactions and prompt improvement. As we advance, users will be able to get more latent personalized service enhanced with contextual information and multimodal interaction capabilities. Improving the learning method makes it easier for the agents to learn new tasks with less data, and collaborating with intelligent systems will improve their performance through shared information.
Moreover, it will ensure that the optimization of sophisticated technologies will occur more ethically and responsibly when implementing them. In conclusion, the development of prompting techniques will enhance the performance of AI agents and create more profound relationships between people and AI to open the path to a smarter and more reactively advanced future in artificial intelligence.