Key Insights
-
Multimodal AI integrates medical imaging, lab reports, genetic data, and real-time monitoring to provide precise diagnoses and reduce errors.
-
By analyzing patient-specific factors like history, genetics, and lifestyle, AI enables tailored treatments that improve outcomes and reduce ineffective therapies.
-
AI-driven automation speeds up diagnostics, optimizes resource utilization, and lowers healthcare costs through early disease detection and reduced hospital readmissions.
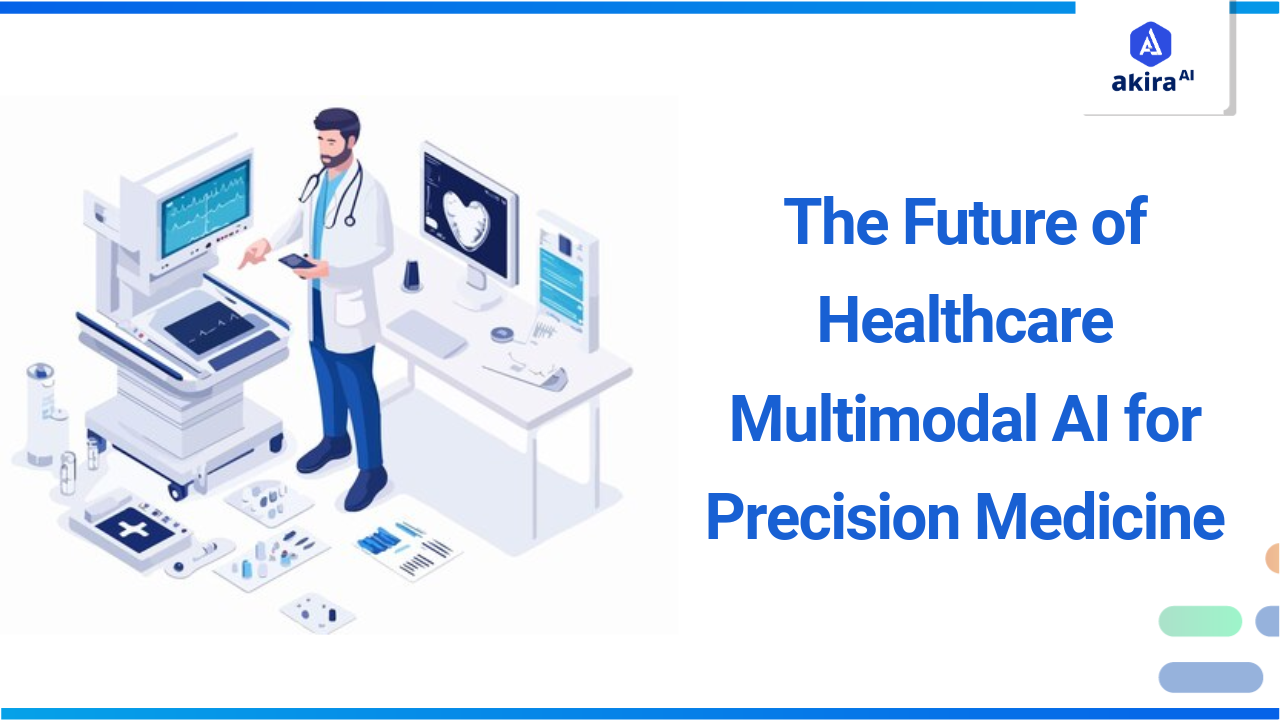
What if diseases could be detected earlier, diagnoses became more accurate, and treatments were tailored just for you? Multimodal AI is making this a reality.
Unlike traditional diagnostics that rely on isolated data, Multimodal AI integrates medical imaging, lab tests, genetic data, and real-time monitoring for a complete health analysis. This leads to faster diagnoses, personalized treatments, and better patient outcomes.
From detecting cancer at its earliest stages to predicting disease progression with unmatched precision, AI Agents is reshaping the future of medicine. In this blog, we’ll dive into how this cutting-edge technology is making healthcare smarter, faster, and more effective than ever before.
What is Multimodal AI in Healthcare Diagnostics?
Multimodal AI in healthcare diagnostics refers to the approach of combining multiple types of medical information—such as medical images, lab test results, patient history, genetic data, and real-time health monitoring—to provide a more accurate and comprehensive diagnosis. Instead of relying on a single source of information, this method integrates different types of patient data to give a holistic view of a medical condition.
Example: AI-Assisted Cancer Diagnosis
Consider a patient suspected of having lung cancer. A traditional diagnostic approach might involve analyzing a CT scan of the lungs and performing a biopsy. However, these methods alone may not provide a complete picture of the disease.
With multimodal AI, the system integrates:
-
CT scan images to detect potential tumors.
-
Pathology reports to confirm cancerous cell presence.
-
Genomic sequencing data to determine if the patient has specific genetic mutations that influence cancer progression.
-
EHRs to analyze medical history, lifestyle factors, and past treatments.
-
Blood test results to detect tumor markers or signs of inflammation.
By combining all these data sources, the AI model can provide a more accurate diagnosis, predict disease progression, and recommend a personalized treatment plan, such as targeted therapy based on the patient’s genetic profile.
Key Concepts of Integrated Medical Data Analysis
Data Fusion: Multimodal AI integrates various data sources such as medical imaging (X-rays, MRIs, CT scans), electronic health records (EHRs), laboratory test results, wearable device data, and genomic sequencing reports. Feature Extraction and Correlation: AI models analyze and extract relevant features from each modality, correlating findings across different data types to provide a more comprehensive diagnosis. Machine Learning and Deep Learning: Multimodal AI leverages machine learning models, particularly deep learning networks, to process vast amounts of heterogeneous medical data and derive actionable insights. Contextual Understanding: Instead of analyzing isolated data points, multimodal AI systems assess the context of each data source, allowing for more accurate and personalized treatment recommendations.
Traditional Approaches to Medical Diagnostics and Treatment Planning
Historically, medical diagnostics relied on a combination of clinician expertise, diagnostic tests, and imaging techniques. Some common traditional approaches include:
-
Clinical Examination: Physicians assess symptoms, medical history, and physical signs to form a preliminary diagnosis.
-
Medical Imaging: X-rays, ultrasounds, MRIs, and CT scans have been pivotal in diagnosing conditions ranging from fractures to tumors.
-
Pathology and Laboratory Testing: Blood tests, biopsies, and genetic screenings help detect infections, cancers, and metabolic disorders.
-
EHR-Based Diagnosis: Electronic health records provide past patient data for reference, but they often lack real-time integration with imaging and other diagnostic sources.
While these traditional methods have been effective, they come with inherent limitations, such as the risk of human error, limited data interpretation, and delays in diagnostic workflows.
Impact on Patient Outcomes Due to Conventional Diagnostic Limitations
Such issues contribute to late diagnosis, less effective treatment strategies, and therefore, worse results in the patients. As such, failing to notice a minor imaging sign or overlooking some abnormality in a lab result may prolong diagnosis to the advanced stages hence affecting the disease treatment’s prognosis as well as vastly influencing the care quality.
-
Delayed Diagnosis and Treatment: When patient data is scattered across different systems, doctors may not have immediate access to crucial information, leading to delays in identifying diseases and starting treatment. This can result in worsened health conditions, reducing the effectiveness of early interventions.
-
Increased Diagnostic Errors: Without a unified approach to analyzing medical data, doctors may misinterpret test results, leading to missed or incorrect diagnoses. Fragmented information increases the risk of human error, which can cause unnecessary treatments or overlooked health issues.
-
Suboptimal Treatment Plans: If a patient’s medical history, lifestyle, and genetic factors are not considered together, treatments may not be tailored to their unique needs. This can lead to ineffective therapies, prolonged illness, and potential complications.
-
Higher Healthcare Costs: A lack of integrated diagnostics can lead to repeated tests, unnecessary hospital stays, and frequent doctor visits, increasing medical expenses for both patients and healthcare systems. Inefficiencies in treatment planning also contribute to wasted resources and longer recovery times.
Akira AI: Multi-Agent in Action
Multimodal AI streamlines healthcare diagnostics by integrating various data sources and processing them through specialized agents. This approach enhances accuracy, enables predictive insights, and ensures timely medical interventions.
Fig 1: Architecture Diagram of Multi-Modal in Healthcare
-
Data Collection & Ingestion
Various sources such as electronic health records (EHRs), medical imaging, genomic data, wearable devices, and patient surveys are gathered. The Data Ingestion Agent preprocesses this information to standardize and organize it for further analysis.
-
Data Processing & Specialized Analysis
Different Domain Specialized Agents analyze specific types of data. The Image Analysis Agent interprets medical scans, the Genomic Analysis Agent identifies genetic markers, and the Patient Monitoring Agent tracks real-time health metrics from wearable devices. These agents extract relevant insights to support diagnosis and treatment planning.
-
Data Integration & Predictive Modeling
The Master Orchestrator Agent aggregates insights from all specialized agents, ensuring seamless integration. The Predictive Analytics Agent then analyzes this combined data to assess health risks, predict disease progression, and generate early warnings for potential complications.
-
Decision Support & Personalized Recommendations
Based on integrated insights, the Decision Support Agent provides tailored treatment recommendations. Healthcare providers receive detailed reports, which help them make informed decisions regarding patient care, medication plans, and lifestyle adjustments.
-
Continuous Monitoring & Real-Time Adjustments
AI-driven alerts notify doctors and patients of critical health changes, allowing for timely intervention. As new data becomes available, treatment plans are dynamically adjusted, ensuring personalized and up-to-date healthcare management.
Prominent Technologies in the Space of Medical Decision Support
Advancements in AI have significantly improved medical decision-making by integrating data-driven insights into diagnostics and treatment. These technologies enhance accuracy, reduce human error, and enable proactive healthcare management.
-
Natural Language Processing (NLP): NLP helps AI analyze and interpret unstructured medical data from clinical notes, research papers, and patient records. This improves information retrieval, enabling better diagnosis and treatment planning.
-
Computer Vision: Agentic AI-powered computer vision analyzes medical images like X-rays, MRIs, and pathology slides to detect anomalies with high accuracy. This assists radiologists and pathologists in identifying diseases at early stages.
-
Predictive Analytics: Machine learning models analyze historical patient data to forecast disease progression and predict treatment effectiveness. This allows healthcare providers to make proactive, data-driven decisions for better patient outcomes.
-
AI-Driven Robotics: AI-assisted robotic systems enhance precision in complex surgeries by minimizing human error and improving procedural accuracy. These robots assist surgeons in delicate operations, reducing complications and recovery time.
-
Wearable AI and Remote Monitoring: Smart wearable devices track vital signs like heart rate, oxygen levels, and activity patterns in real time. They help detect early health issues and reduce hospital visits by providing continuous remote monitoring.
By integrating these AI-driven technologies, healthcare systems can improve patient outcomes, reduce diagnostic delays, and enhance overall efficiency in medical decision-making. As AI continues to evolve, its role in healthcare will become even more transformative.
Benefits: Transforming Patient Care Through Multimodal Intelligence
Multimodal intelligence in healthcare enhances patient care while delivering a strong Return on Investment (ROI) for hospitals, insurers, and healthcare providers. It optimizes diagnostics, improves efficiency, and reduces overall costs.
Reduced Diagnostic Errors → Cost Savings on Malpractice & Readmissions
AI-driven diagnostics minimize misdiagnoses, leading to fewer malpractice claims and unnecessary treatments. Studies show that reducing diagnostic errors can save healthcare systems $20–30 billion annually by preventing avoidable complications and hospital readmissions.Faster & More Accurate Diagnoses → Increased Operational Efficiency
Automated data processing speeds up disease detection, decreasing patient wait times and hospital stays. AI-enabled hospitals report a 30–40% improvement in workflow efficiency, allowing providers to treat more patients without increasing staff workload.Personalized Treatment Plans → Better Patient Outcomes & Retention
AI-powered recommendations lead to more effective treatments, improving patient satisfaction and reducing treatment failures. Hospitals implementing personalized care strategies see a 15–20% increase in patient retention and follow-up engagement, boosting long-term revenue.Preventive Care & Remote Monitoring → Lower Long-Term Healthcare Costs
Wearable devices and predictive analytics enable early disease detection, reducing emergency visits and hospital admissions. Studies indicate that remote patient monitoring can lower hospitalization costs by 25–30%, translating to significant savings for both providers and insurers.Optimized Resource Utilization → Higher Revenue & Profitability
AI-driven automation streamlines administrative tasks, optimizes staff scheduling, and reduces paperwork. Large-scale health systems report $50 million+ annual savings through AI-driven operational efficiencies, increasing overall profitability.
How AI Agents Supersede Other Diagnostic Technologies
Modern diagnostic approaches have surpassed traditional methods by integrating multiple data sources, improving accuracy, and enabling faster decision-making. Unlike conventional techniques that rely on isolated test results, newer solutions provide a more comprehensive understanding of a patient’s health.
-
Multimodal Data Integration: Combining information from medical imaging, lab reports, genetic analysis, and real-time health tracking creates a holistic view of a patient’s condition. This reduces reliance on a single test, leading to more precise diagnoses.
-
Higher Accuracy and Reduced Errors: Automated systems help detect subtle patterns in medical data that might be overlooked in manual assessments. This minimizes misdiagnoses and ensures more reliable clinical decisions.
-
Faster Decision-Making and Early Detection :Real-time analysis speeds up disease identification and treatment planning, reducing delays that could impact patient outcomes. Early detection is crucial for managing chronic and life-threatening conditions.
-
Personalized Treatment Approaches: By analyzing a patient’s history, genetic factors, and lifestyle, modern systems support customized treatment plans. This improves effectiveness compared to standardized medical protocols.
-
Continuous Learning and Adaptation: Unlike traditional diagnostic tools, modern systems improve over time by incorporating new medical research and patient data. This ensures that healthcare decisions are based on the latest available information.
Successful Implementations of AI Agents in Major Healthcare Systems
-
IBM Watson Health in Oncology: Some cancer centres have adopted Watson to help in diagnosis and developing treatment plan base on patient records, publications as well as scan images in a bid to increase chances of survival rates.
-
DeepMind Health for Eye Diseases: The AI developed by DeepMind is capable of detecting diabetes retinopathy through scanning of the eyes; this means a way of sparing the patient from going through invasive diagnosis.
-
NVIDIA Clara in Radiology: Several hospitals have witnessed enhanced acceleration in the process of analysing images and enhance efficiency in the radiology departments and have also seen a decrease in the number of misdiagnoses.
-
Google DeepMind’s AlphaFold: AlphaFold has transformed drug discovery by accurately predicting protein structures, a task that previously took years. This breakthrough accelerates research in developing new treatments for diseases like Alzheimer’s and cancer.
-
Aidoc and Qure.ai: These AI-driven imaging tools assist radiologists by automatically detecting abnormalities in medical scans. By reducing diagnostic errors and workload, they enhance efficiency and ensure faster, more accurate interpretations.
These success stories underline how multimodal AI is already reshaping diagnostics and treatment planning across diverse healthcare settings.
Next Steps with MultiModal AI
Talk to our experts about implementing Multimodal AI in healthcare, where agentic workflows and decision intelligence enhance precision medicine. Utilize AI to automate diagnostics, optimize treatment planning, and improve patient outcomes with data-driven decision-making.