Key Insights
AI agents are revolutionizing predictive network maintenance in telecom by enabling proactive issue detection and resolution. These agents analyze real-time data to predict failures, minimizing downtime and optimizing resources. With seamless integration across networks, AI agents improve scalability and operational efficiency.As the technology continues to evolve, telecom companies can expect even greater automation, sustainability, and improved customer satisfaction.
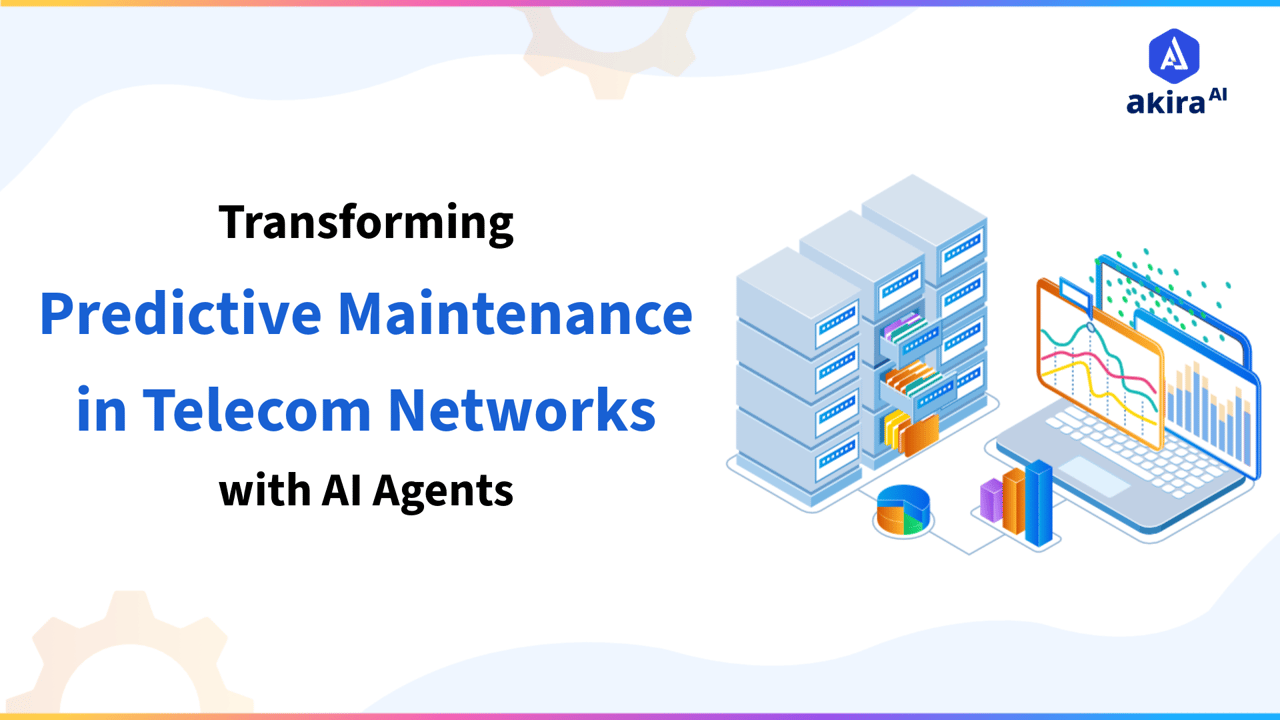
What if your telecom network could predict problems before they even occur? Sounds futuristic, right? Well, AI agents are making this a reality today. The telecom industry is increasingly turning to predictive network maintenance powered by Agentic AI, and the results are nothing short of transformative. Experts predict that the global market for AI Agents for telecom will grow significantly in the coming years, with predictive maintenance being one of its most valuable applications. In fact, a growing number of telecom companies are already harnessing the power of AI agents to proactively identify and fix issues, saving time, money, and resources.
In this blog, we'll explore how AI agents are reshaping predictive maintenance in telecom, helping companies ensure continuous service and stay ahead of potential disruptions. Prepare to see how AI Agents is not just a tool, but a true game-changer for the telecom industry.
What is Network Maintenance?
Network maintenance can be defined as the holistic exercise to ensure that the performance and efficiency of telecommunications networks operate perfectly. Routine inspections are the basis for identifying potential problems that could arise, while periodic updating or patching is applied so security and performance are not at risk. Configuration management effectively offers grounds for troubleshooting and optimization; continuous performance monitoring will assist the organization in detecting bottlenecks and allocating resources adequately, and regular backups for quick recovery from failures. Even if such practices are standardized incident response, cleaning up unexpected outages should help quickly. Proactive approaches to network maintenance should then ensure minimizing downtime, reliability increases, and improved user satisfaction critically necessary elements of successful operations for today's telecom activities.
A Brief Overview of Predictive Network Maintenance in Telecom
Predictive network maintenance is a modern strategy that uses big data analytics, machine learning, and artificial intelligence algorithms to detect probable failure and maintenance areas within the telecommunications network ahead of time. It isn't as traditional as traditional maintenance schedules or even immediate response to failures as predictive maintenance determines actions on real-time based data. This means problems are detected at an early stage when they are yet to be major issues, the risk of unforeseen outages is reduced drastically, and maintenance costs are minimized. Predictive network maintenance thus develops overall network reliability and encourages more proactive and efficient management approaches to network resources.
Traditional vs. Agentic AI Predictive Network Maintenance
Feature |
Traditional Predictive Maintenance |
Agentic AI-Based Predictive Maintenance |
Intelligence |
Based on historical data and predefined models |
Learns and adapts in real-time from network data |
Task Complexity |
Identifies simple, recurring issues |
Analyzes complex, dynamic network conditions and predicts future issues |
Integration |
Limited integration with network components |
Seamless integration across network systems, including IoT and real-time analytics |
Scalability |
Difficult to scale for large, complex networks |
Easily scalable to handle large-scale, distributed networks |
Response to Exceptions |
Requires manual intervention for irregularities |
Autonomous decision-making and issue resolution in real-time |
Operational Efficiency |
Moderate improvements in maintenance schedules |
Significant reductions in downtime and resource utilization with proactive interventions |
Maintenance Prediction |
Reactive, based on predefined thresholds |
Proactive, predicting potential failures before they occur |
Cost Management |
This may lead to unnecessary maintenance and costs |
Optimizes resources, reduces unnecessary downtime, and minimizes operational costs |
AI Agents in Action: Architecture and Process Flow
Fig 1: Architecture Diagram of Predictive Network Maintenance
The integration of AI agents into predictive network maintenance involves a multi-agent architecture that optimizes data collection, analysis, and maintenance actions. Below is a simplified architecture diagram illustrating this workflow:
A predictive network maintenance framework comprises several subcomponents with high functionalities for enhancing the reliability of the network.
-
Data Collection Agent: This module retrieves real-time data from sensors and network equipment for always-available network performance monitoring. These metrics accumulate, including crucial traffic load, latency, and health of equipment that the base requires further analysis.
-
Data Analysis Agent: Analyzes the collected data using machine learning algorithms to detect anomalies and predict potential failures.
-
Prediction Engine: The prediction engine has actionable insights and then related forecasting about network conditions. It lets the teams decide how to intervene and help in decisions about resource allocation
-
Maintenance Agent: This carries out the maintenance actions required or notifies personnel of what needs to be done. It is a very vital function in averting downtime; that is, the whole network operates better.
-
Action and Feedback Loop: The results of the maintenance work are fed back into the system. This creates an endless feedback loop that makes continuous process optimization possible in predictive maintenance, as algorithms improve with increased accuracy over time. All these functions come together into a working configuration that not only predicts but also smoothes out the fast response system, in turn making the network even better and efficient.
Applications in Predictive Network Maintenance
-
Network Performance Monitoring: AI agents continuously monitor network performance in real-time and can modify functionality right there on the spot. Regular proactive control of dips in performance keeps service levels at their optimal level.
-
Fault Prediction: Telecom companies apply machine learning algorithms on both historical and real-time data that may predict potential faults and then act before faults develop in their service.
-
Resource Allocation: It optimizes resource allocation in maintenance activities, and uses data and predictions to apply the right resources at the right time, thus optimizing their effectiveness while avoiding waste.
-
Cost Reduction: Early detection of maintenance needs helps AI ensure that a small problem would not transform into a full-scale outage. Generally, this contributes to high savings in the cost of operation in case such may come out from emergencies and downtime.
-
Improved Customer Experience: Predictive maintenance would mean fewer service disruptions and more reliable connectivity, hence better customer satisfaction for users who experience better service that is more constant and reliable.
Benefits of Predictive Network Maintenance
Integrating AI agents into network maintenance brings numerous operational benefits that enhance overall performance:
Enhanced Efficiency: AI agents will automate as much as 80% of routine maintenance by 2025. Human operators are free from doing routine tasks, and they shift their focus to more strategic initiatives driving innovation and growth.
Increased Productivity: Predictive maintenance techniques usually result in improvements in productivity up to 30% in an operations environment. Organizations can now keep operations running smoothly and deliver constant service through fault detection and early intervention.
Cost Savings: This decrease also saves unscheduled outages. Many savings would there be for the telecom companies concerning amount. The system would have higher reliability, fewer disruptions, and cheaper repair costs, hence thereby boosting the overall ROI.
Together, these benefits illustrate how AI agents can transform network maintenance, making operations more efficient, productive, and cost-effective.
Technologies Transforming Telecom Maintenance with AI Agents
The integration of advanced technologies like IoT devices, machine learning, cloud computing, and big data analytics significantly enhances network maintenance processes:
-
IoT Devices: These devices play a crucial role in real-time data collection from network equipment. By continuously monitoring performance metrics and operational conditions, IoT devices provide valuable insights into potential issues, allowing for timely interventions.
-
Machine Learning: This technology enables predictive analytics, which is essential for early identification of maintenance needs. By analyzing historical and real-time data, machine learning algorithms can forecast when equipment is likely to fail, allowing teams to act proactively and reduce downtime.
-
Cloud Computing: Offering scalable processing capabilities, cloud computing enhances data analysis and storage. It allows for the efficient handling of large datasets generated by IoT devices, ensuring that insights can be processed quickly and accessed from anywhere.
-
Big Data Analytics: This technology is vital for processing vast amounts of data to uncover patterns and predict failures. By analyzing large datasets, organizations can identify trends that indicate potential problems, leading to more informed decision-making and effective maintenance strategies.
Future Trends in Predictive Network Maintenance
The future of predictive network maintenance with AI agents promises to be transformative, driven by several key trends:
-
Deeper Integration: There will be more integration into the existing network management systems of AI agents. This will improve usability and fluid operations. There should be easier flows, more coherent management strategies, and an ability through which operators monitor and manage network health better.
-
Advanced Analytics: As analytics technologies continue to evolve, they will provide even deeper insights into network performance and maintenance requirements. Enhanced analytical capabilities will enable organizations to uncover complex patterns and trends, leading to more informed decision-making and proactive maintenance strategies.
-
Decentralized Decision-Making: AI will facilitate localized decision-making, allowing for quicker responses to fluctuations in network conditions. By empowering local systems to analyze data and make decisions autonomously, organizations can enhance responsiveness and adaptability, minimizing downtime and improving service continuity.
-
Sustainability Initiatives: Future applications of AI in network maintenance will increasingly prioritize sustainability. By optimizing resource usage and reducing energy consumption, these initiatives will help telecom companies minimize their environmental impact while maintaining efficient operations.
Conclusion: AI Agents for Predictive Network Maintenance
The integration of AI agents in predictive network maintenance is a game-changer for the telecom industry, enabling organizations to anticipate issues before they disrupt services. By leveraging AI, IoT, and advanced analytics, telecom operators can move from reactive to proactive maintenance, optimizing resources, reducing downtime, and significantly cutting costs. As Agentic AI technology evolves, telecom companies will continue to gain a competitive edge by ensuring more reliable networks, improving customer satisfaction, and driving innovation. The future of telecom network maintenance lies in the seamless collaboration between human expertise and AI-powered automation, setting the stage for smarter, more efficient, and resilient networks. The shift to predictive maintenance is no longer just a possibility; it’s a strategic necessity for telecom companies aiming to stay ahead in an increasingly digital world.