Key Insights
In the fast-paced world of FMCG, optimizing shelf space allocation and merchandising is crucial for maximizing sales and enhancing customer experience. By leveraging Agentic AI, businesses can analyze vast amounts of data to dynamically adjust product placement based on factors like demand trends, consumer behavior, and competitor activity. Ultimately, Agentic AI empowers brands to deliver tailored, data-backed strategies that drive growth and enhance the shopper's journey.
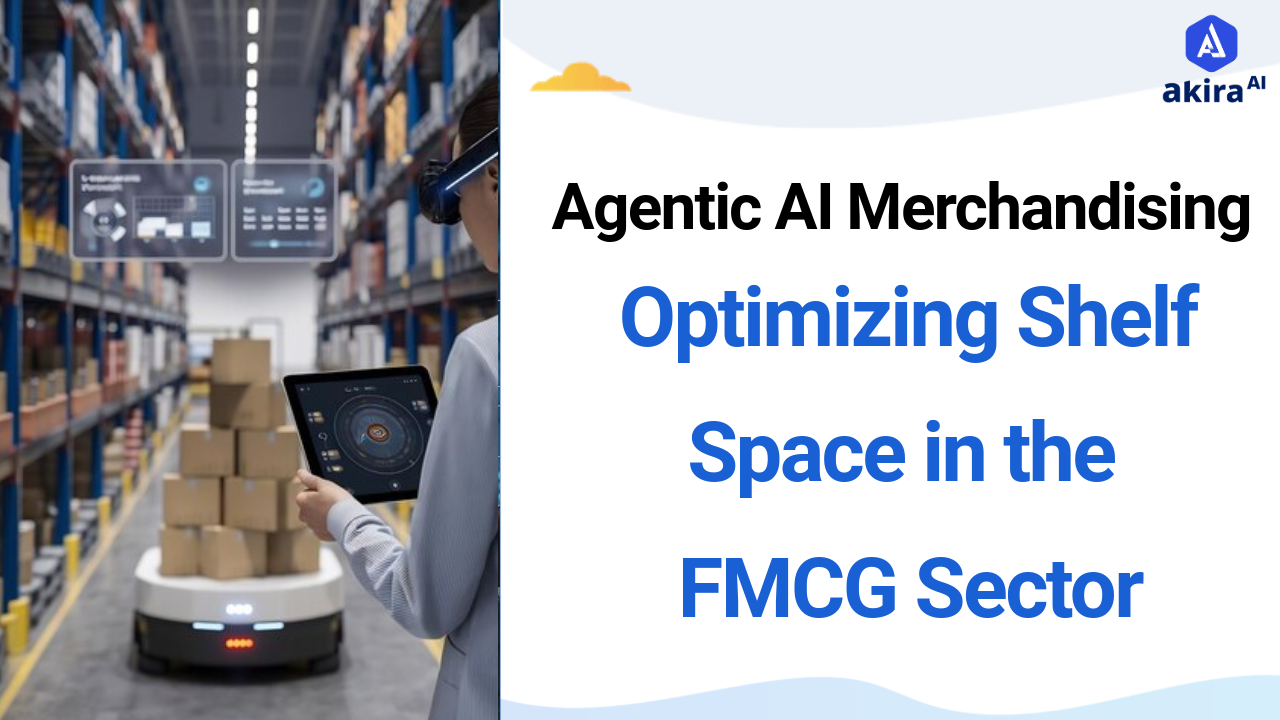
Are you looking to improve how products are displayed in your store? Shelf space allocation and merchandising optimization are essential strategies for achieving this, and agentic AI is the secret tool that makes it possible. By leveraging AI agents like those in Akira AI, FMCG companies can dynamically optimize store shelves in real-time. This ensures that products end up where they are most likely to be wanted and, therefore, sell most often. In this blog, we’ll take a closer look at how AI is transforming the retail space, making product management smarter, more efficient, and more profitable for retailers.
What is Shelf Space Allocation and Merchandising Optimization?
Shelf space allocation refers to the process of determining how to assign retail space effectively to different products on store shelves. This practice has a direct implication on retail planning and optimization because it alters external product exposure levels as well as consumer options, ultimately affecting sales results. Merchandising optimization is closely tied to this, focusing on improving product placement, assortment, pricing, and promotions to enhance sales performance.
In the FMCG industry, where products are consumed quickly and need to be replenished frequently, managing shelf space and optimizing merchandising are vital for staying competitive. There is enormous consumer demand for products within this sector; therefore, getting the product on the retail shelf and the buyer’s radar contributes significantly to sales and margins.
Key Concepts of Shelf Space Allocation in CPG
Shelf Space Management: This encompasses a kind of rationing process whereby every product is rated potentially based on how much space it deserves on the shelf in order to maximize sales.
Product Assortment: Refers to the strategic selection of products within a specific category, ensuring that the product mix meets customer demands and enhances the shopping experience.
Product Placement: Involves determining the physical arrangement of products on store shelves to maximize visibility, increase customer engagement, and improve the likelihood of purchases.
Pricing Strategy: Specifically targeting, the goal was to implement the right prices that would entice the customers to buy by equally considering the cost of products and the amount of money the retailer needs to earn for the product to be more appealing to the customer.
Promotions: Tactics like discounts or product displays designed to attract customer attention, stimulate interest, and boost sales for certain products or categories.
Traditional Way of Shelf Space Allocation and Merchandising Optimization
In the past, shelf space allocation and merchandising optimization were often handled manually, relying on basic data and human judgment. This traditional approach created several challenges for retailers and companies in the CPG industry.
-
Manual Decision-Making: Traditionally, methods involved direct manual allocation of shelf space or using simple computing structures that only incorporated historical physical unit sales. These methods did not provide information in real-time to make full optimal decisions.
-
Gut Feelings Over Data: Store managers often relied on intuition instead of data-driven insights. Through this, decisions were made in light of previous occurrences and not with the current growing demand.
-
Inefficiency in Product Placement: Traditional shelf space management often led to poor placement of high-demand or high-margin items. This caused missed sales opportunities and inefficient use of space.
-
Lack of Flexibility: Often, traditional merchandising optimization was not able to grow well and was rigid in adjusting to market changes. FMCG companies often introduce new products, but traditional methods cannot keep up.
-
Slow Response Times: Traditional methods were slow to respond to market shifts. CPG companies missed trends or consumer preferences, affecting sales.
Impact on Customers Due to Traditional Shelf Space Allocation
Inefficient shelf space allocation affected not only CPG companies but also the customer experience. Poor product placement led to frustration and missed sales opportunities.
-
Dissatisfaction from Poor Product Placement: Inefficient shelf space management frustrated customers, making it difficult to find products. Disorganized or cluttered shelves led to a poor shopping experience.
-
Missed Sales Opportunities: CPG companies lost sales when popular products were out of stock or placed in the wrong areas. Traditional methods couldn’t ensure the right products were available at the right time.
-
Overstocking of Slow-Moving Items: Traditional methods often led to overstocking of low-demand products. This occupied valuable space that could be used for higher-demand or faster-selling items.
-
Difficulty in Adjusting to Promotions: Traditional merchandising optimization struggled to accommodate promotions quickly. As a result, products didn’t get the visibility or placement they needed during important sales periods.
Akira AI: Multi-Agent in Action
Fig1: Workflow Diagram of Shelf Space Allocation
Akira AI is an advanced agentic AI solution designed specifically to tackle the challenges of shelf space allocation and merchandising optimization in the FMCG industry. Akira’s multi-agent system features a number of AI agents that share in the decision-making process and, more specifically, the task of optimally managing the retail shelf space and improving customer perceived value.
The system consists of several AI agents with specialized functions:
-
Master Orchestrator: The Master Orchestrator is a central point in the multi-agent system, controlling and managing communication with other agents. It makes certain that all the actions of the agents promote overall strategy and objectives while allowing the occasional modification of the actions and responses in real-time.
-
Product Demand Agent: The Product Demand Agent analyses the patterns of product demand and the factors that may influence the level of demand for products, such as cyclical, seasonal, promotional, or even climatic factors. It helps ensure that products are available in the right quantities, avoiding both stockouts and overstocking.
-
Placement Optimization Agent: The Placement Optimization Agent is responsible for decision-making regarding how best to position a particular product on the shelves. It employs user statistics to enhance product exposure and availability, thereby increasing customer relations as well as volume sales.
-
Stock Level Agent: The Stock Level Agent checks the stock status continuously in real-time and notifies when the stock status is low or when the product is out of stock. It plays a part in starting the right time restocking processes so that the shelves are well stocked with the appropriate products.
-
Pricing Strategy Agent: The Pricing Strategy Agent analyzes competitor prices and adjusts product pricing dynamically. It ensures products remain competitive while maximizing profit margins, contributing to a more efficient pricing strategy.
-
Consumer Behavior Agent: By analyzing consumer interactions and purchasing patterns, the Consumer Behavior Agent refines product assortment and placement strategies. It tailors the shopping experience to consumer preferences, increasing sales potential and customer satisfaction.
Prominent Technologies in Agentic AI Shelf Space Allocation and Merchandising Optimization
Over the last few years, the possibilities in AI and machine learning have produced new technologies that enhance shelf space management and merchandising at an entirely different level. These technologies help businesses better understand consumer behaviour, forecast demand, and optimize product placement in real-time. Some prominent technologies include:
-
Computer Vision: This technology provides real-time information on the shelf occupancy and position of products. It assists in determining issues of stock outs, misplaced or off-display products to ensure that the shelves are optimally stocked as well as arranged.
-
Predictive Analytics: By analyzing historical data and real-time insights, predictive analytics forecasts future product demand. This helps the retailers provide the right stocks at the right place, thus reducing stock-out situations and, at the same time, not overstocking.
-
Machine Learning Algorithms: Machine learning continuously analyzes sales data, customer preferences, and market trends. This enables CPG companies to fine-tune retail merchandising and proactively adjust shelf space.
-
Reinforcement Learning: This artificial intelligence technique independently helps agents enhance decision-making based on feedback. It makes it possible for organizatiZons to learn in the process and change shelf space management approaches and store placements correctly.
With Agentic AI, FMCG businesses can optimize forecasting, enhance customer experiences, and drive efficiency. Source – How AI Agents Improve FMCG Consumer Behaviour Forecasting.
Successful Implementations of AI Agents in the Fast-Moving Consumer Goods
1. Coca-Cola
Coca-Cola uses AI to enhance its marketing by analyzing customer data to refine targeting strategies. AI helps the company segment audiences based on preferences and behaviours, allowing for personalized and more effective marketing campaigns.
Impact: Coca-Cola’s AI-driven insights have improved the effectiveness of its campaigns, leading to better targeting, higher engagement, and stronger ROI by aligning marketing efforts with customer preferences.
2. Mondelez International
Mondelez employs AI for global retail analytics, analyzing consumer behaviour and market trends across regions. This helps the company optimize sales strategies and tailor marketing campaigns to local markets while maintaining global efficiency.
Impact: AI has enhanced Mondelez’s ability to adapt marketing strategies to local preferences while maintaining overall global efficiency and boosting competitiveness and market presence.
3. Johnson & Johnson
Johnson & Johnson uses AI to gather consumer health insights from various data sources. This helps align product development with emerging health trends and consumer needs, particularly in wellness and medical products.
Impact: AI has strengthened Johnson & Johnson’s market position by enabling the company to effectively address emerging health trends, improve product development, and increase customer satisfaction.
Future Trends: How AI Agents Supersede Other Technologies
-
Real-Time Adjustments Through Advanced AI Capabilities: Agentic AI will surpass traditional technologies by making real-time adjustments based on micro-trends, seasonal shifts, and global events. This allows retailers to stay ahead of trends and better meet consumer demand as it changes in real-time.
-
Agentic AI and IoT Integration for Hyper-Personalization: AI integrated with IoT will provide more precise data, allowing for hyper-personalized shopping experiences. This will enable tailored product offerings, pricing, and placements, boosting customer satisfaction and sales potential.
-
Predictive Analytics for Inventory and Demand Forecasting: AI agents will enhance the system's ability to predict inventory needs and demand patterns, reducing waste and increasing efficiency. These predictions will help retailers stock the right products at the right time, improving overall inventory management and customer satisfaction.
-
Enhanced Customer Insights and Experience: AI will provide deeper insights into consumer behaviour, enabling businesses to personalize interactions further and optimize the shopping experience. AI will allow retailers to refine marketing strategies by analysing buying habits and preferences, resulting in stronger customer loyalty and increased sales.
Conclusion: AI Agents for Shelf Space Allocation
It’s clear that agentic AI is the future of shelf space allocation and merchandising optimization. With AI agents constantly improving product placement and stock management, CPG companies and retailers are achieving better results with less effort. These agents make real-time decisions to optimize store shelves, ensuring that products are always stocked, visible, and strategically placed. This leads to higher sales, reduced waste, and improved customer satisfaction. Embracing AI in optimizing shelf space is a smart move for any business looking to stay ahead of the curve and succeed in the competitive FMCG industry.