Key Insights
AI agents revolutionize task management with autonomous functionality, enhancing efficiency and reducing manual oversight. They excel at data processing, providing valuable insights for data-driven decision-making. Through machine learning, AI agents continuously improve, offering personalized responses and real-time decision-making. Their scalability and integration capabilities make them ideal for various industries, boosting productivity and customer satisfaction.
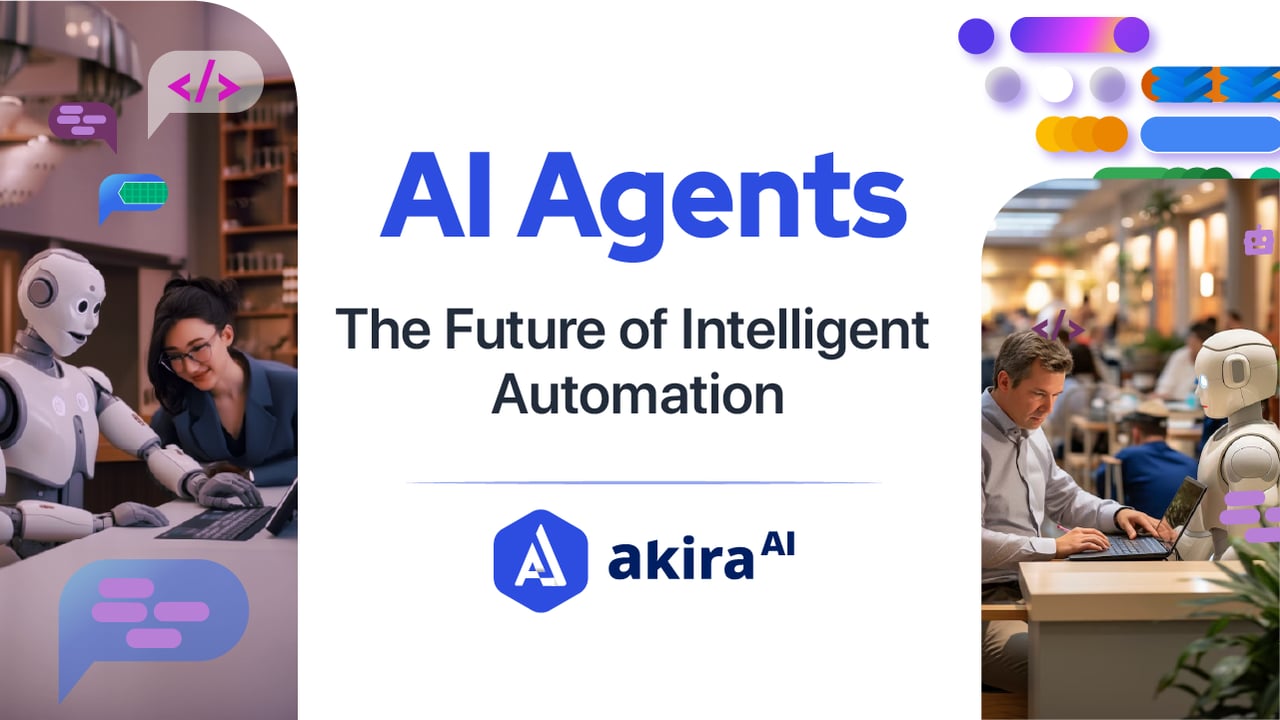
The field of artificial intelligence (AI) has witnessed remarkable evolution, transitioning from early machine learning (ML) models to sophisticated AI agents capable of dynamic and autonomous functioning. This progression reflects a shift from basic task automation to advanced systems that not only perform complex tasks but also learn, adapt, and make decisions with minimal human intervention.
Traditional ML models and robotic process automation (RPA) laid the groundwork, but the advent of AI agents marks a significant leap towards more intelligent and flexible systems. As AI agents become increasingly integral across various industries, understanding their evolution, capabilities, and impact is crucial for leveraging their full potential.
Evolution of Agentic AI
Automation has evolved significantly, moving from traditional Machine Learning (ML) to Deep Learning, Robotic Process Automation (RPA), and now to AI Agents. Each stage has advanced the capability to handle complex tasks, evolving from rule-based systems to intelligent systems that reason, learn, and adapt.
-
Traditional ML and Deep Learning: Early ML models like linear regression and SVMs were task-specific and required extensive training and retraining to adapt to new patterns. They excelled in tasks such as predictive analytics and customer segmentation but had limited flexibility.
Deep Learning, a subset of ML, introduced neural networks capable of analyzing unstructured data like text, images, and audio. Despite their advancements, these models still required human supervision and retraining.
-
Robotic Process Automation (RPA): RPA excels at automating repetitive, structured workflows, such as data entry and transaction processing. However, it struggles with unstructured workflows and complex decision-making, limiting its effectiveness in scenarios requiring intelligence.
-
AI Agents: The Next Evolution: AI Agents address the limitations of RPA and task-specific ML models by autonomously performing tasks, learning from data, and adapting to new situations without constant human oversight. They continuously improve their performance through interactions and historical data, making complex decision-making more efficient.
-
The Shift Towards Agentic AI: Agentic AI represents a shift from static, rule-based systems to dynamic, intelligent agents with contextual understanding and autonomous decision-making capabilities. This evolution is driven by advancements in orchestration, natural language processing, and advanced neural networks.
What are AI Agents?
The agents use advanced algorithms that include massive data processing and machine learning while interpreting data, making decisions, and performing actions. Its applications range from improving consumer service through virtual assistants to very complex data analysis. Its implications as AI agents for automating repetitive work assignments imply enhancing effectiveness, accuracy, and productivity across various industries.
There are different classifications of agents concerning functionality and learning. The reactive agent works according to reaction to specific inputs or changes in the environment; however, it does not have a memory relative to past activities. Instead, proactive agents exhibit planning and decision-making, both being considered together: the current data as well as the historical background.
While collaborative agents collaborate with humans in various helper duties as supplements to human functions, learning agents improve their performance through new knowledge obtained from experience and data. Such adaptability forms the basic foundation of the artificial intelligence agent's applicability across all wide-ranging sectors, which include finances, healthcare, and human resources, to optimize procedures, guide decisions, and offer personalized insights.
How do AI agents work?
Large Language Models (LLMs) like GPT-4 represent a specialized subset of AI agents focusing on natural language understanding and generation. This relationship is multifaceted and enhances the capabilities of AI agents in several key ways:
Fig1: Flow Diagram of AI Agents
-
Natural Language Processing (NLP): LLMs significantly enhance AI agents' ability to understand and respond to human language, making interactions more natural and intuitive. This is crucial for applications such as customer service chatbots and virtual assistants, where understanding user queries accurately and responding appropriately is essential.
-
Enhanced Decision-Making: LLMs can analyze and synthesize vast amounts of text data, providing AI agents with deeper insights and context for better decision-making. For instance, they can process and summarize reports, extract relevant information from large datasets, and provide comprehensive answers to complex questions, which helps in informed decision-making across various domains.
-
Continuous Learning: LLMs are designed to learn from new data continuously, enabling AI agents to improve their performance and adapt to new information over time. This ongoing learning process allows AI agents to stay up to date with the latest information, trends, and language usage patterns, which is particularly beneficial in dynamic fields such as finance and healthcare.
-
Versatility and Scalability: They equip AI agents with the ability to handle a diverse range of tasks, from generating detailed reports to engaging in complex conversations. This versatility allows AI agents to be deployed in various scenarios, providing scalable solutions that can cater to different business needs. For example, in customer service, an AI agent powered by an LLM can handle everything from simple FAQ responses to complex problem-solving interactions, thereby enhancing overall functionality and efficiency.
Impact of Agents on Decision-Making
-
Enhanced Data Analysis: AI agents revolutionize data analysis by swiftly processing and analyzing vast amounts of information with unparalleled speed and precision. Unlike human analysts, AI agents can sift through massive datasets to uncover hidden patterns and correlations that might be overlooked in manual reviews. They employ advanced statistical techniques and machine learning algorithms to generate actionable insights, facilitating more informed and strategic decision-making.
-
Real-Time Decision Making: One of the key advantages of AI agents is their ability to provide real-time insights and recommendations. This instantaneous processing capability allows businesses to adapt quickly to emerging trends and unforeseen changes. AI agents can continuously monitor data streams, detect anomalies, and offer timely suggestions, enabling organizations to make swift, evidence-based decisions and maintain a competitive edge.
-
Reduced Bias: AI agents mitigate the impact of cognitive biases that can skew human judgment. By relying on objective data-driven analysis rather than subjective opinions, AI agents help ensure that decisions are based on factual evidence. This reduction in bias enhances the fairness and consistency of decision-making processes, leading to more equitable outcomes and improved organizational transparency.
-
Improved Predictive Capabilities: AI agents leverage sophisticated algorithms and historical data to forecast future trends and potential outcomes. By analyzing past behaviours and current patterns, they generate predictive models that help businesses anticipate market shifts, customer needs, and operational challenges. This foresight enables organizations to implement proactive strategies, optimize resource allocation, and stay ahead of potential disruptions.
Key Challenges In Implementing AI Agent
-
Data Privacy and Security: Ensuring robust protection of sensitive information handled by AI agents is critical. Organizations must implement comprehensive data security measures and comply with privacy regulations to safeguard against breaches and unauthorized access.
-
Bias and Fairness: AI agents can inadvertently reinforce biases present in training data, potentially leading to biased d ecision-making. Addressing this requires ongoing efforts to identify and mitigate bias through careful data management and regular audits.
-
Explainability and Transparency: Many AI agents, particularly those employing complex models, operate as "black boxes," making their decision-making processes opaque. Enhancing transparency and explainability is essential for building trust and ensuring accountability..
-
Integration with Existing Systems: Incorporating AI agents into established business processes and IT systems can be complex and resource-intensive. Effective integration necessitates careful planning to address compatibility issues and minimize disruption.
-
Cost and Resource Intensity: The development, deployment, and maintenance of AI agents can entail significant financial and resource investments. Organizations must evaluate the cost-benefit balance to ensure that the benefits of AI adoption outweigh the associated expenses.
Comparison Between Traditional AI vs. AI Agent
Aspect |
Traditional AI |
AI Agents |
Autonomy |
Traditional AI systems operate with limited autonomy. They rely on predefined rules and algorithms to perform specific tasks. Any new behavior or decision requires human intervention to update or modify the system. |
AI Agents are highly autonomous. Once deployed, they can make decisions, learn from their environment, and take action without constant human supervision. This makes them more versatile in handling complex, dynamic tasks. |
Task Handling |
Traditional AI systems are typically designed for a single, well-defined task, like image recognition or spam filtering. They excel at these tasks but are limited to their specific function and can’t handle multiple unrelated tasks effectively. |
AI Agents, especially in multi-agent systems, can handle a range of tasks simultaneously. For example, an AI agent in a healthcare system could manage appointment scheduling, patient monitoring, and decision-making in treatment plans all at once. |
Adaptability |
Traditional AI systems are less flexible and struggle with new or unforeseen situations. They need to be retrained or reprogrammed if there’s a change in their task environment or input data. |
AI Agents are highly adaptable. They can adjust to new situations by learning from their interactions and environment, using techniques like reinforcement learning or feedback loops, allowing them to evolve without needing constant retraining. |
Interaction |
Traditional AI is often reactive. It waits for input and then processes it based on fixed rules or data. This reactive nature limits its ability to anticipate user needs or adapt in real time. |
AI Agents are proactive. They interact dynamically with users, systems, and environments, often predicting user needs or preemptively solving problems. For example, a customer service AI agent might suggest solutions before the user even asks. |
Collaboration |
Traditional AI systems typically function in isolation. They focus on solving specific problems individually, with no ability to collaborate with other systems or agents. |
AI Agents can collaborate with other agents and systems to solve complex, interconnected problems. In a multi-agent system, several agents may work together, each focusing on different aspects of a problem to achieve a common goal, such as in fraud detection or supply chain optimization. |
Scalability |
Traditional AI systems require manual scaling. When a new task or function needs to be added, it often involves creating new models or upgrading existing ones, which can be resource-intensive. |
AI Agents are easily scalable, especially in multi-agent environments. You can add more agents or extend their capabilities without major infrastructure changes, allowing for efficient handling of growing or evolving tasks. |
Conclusion
The evolution from traditional AI models to advanced AI agents represents a transformative shift in how we approach automation and decision-making. While traditional ML and RPA provided valuable tools for specific tasks, AI agents offer a new level of autonomy, adaptability, and intelligence. They excel in handling complex, dynamic environments and provide real-time, data-driven insights that enhance decision-making processes.
Despite challenges such as data privacy, bias, and integration complexities, the benefits of AI agents—ranging from improved data analysis and reduced bias to enhanced predictive capabilities—are substantial. As industries continue to adopt and integrate AI agents, their role in driving efficiency, innovation, and strategic decision-making will become increasingly pivotal.