Key Insights
-
Advanced AI-powered tools like Akira AI enable precise demand forecasting, helping optimize routes, schedules, and resource allocation for public transit systems.
-
Multi-agent workflows leverage specialized AI teammates for data analysis, forecasting, and decision-making, improving operational agility and responsiveness.
-
Agentic AI-driven innovations pave the way for eco-friendly transit systems and hyper-personalized passenger experiences, driving the evolution of urban mobility.
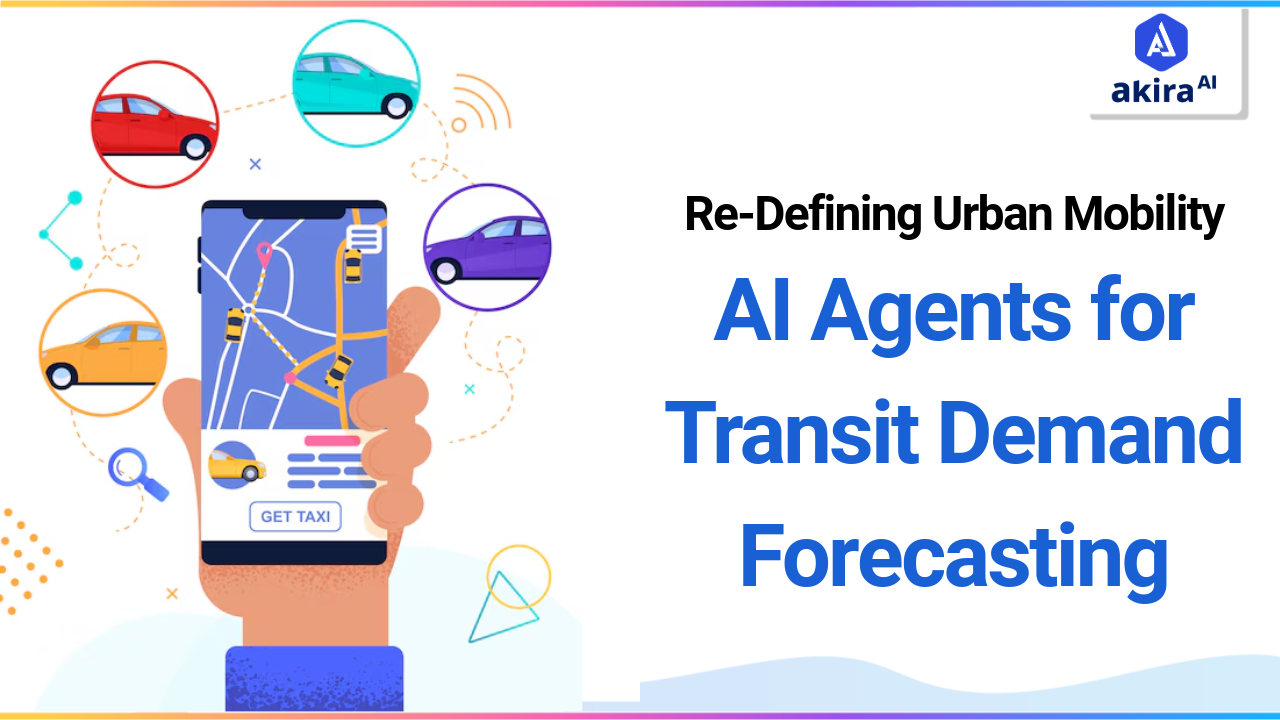
Public transportation systems are the lifeblood of urban mobility, enabling millions of people to commute daily. However, optimizing these systems to meet ever-changing passenger demands effectively has always posed a significant challenge. Thankfully, AI-powered demand forecasting is revolutionizing how we approach this issue.
By leveraging AI agents to predict and manage passenger volumes, tools like Akira AI provide a vision for the future of smarter, more efficient public transportation. In this blog, we’ll dive into how these AI-driven tools reshape transportation services, making the experience smoother and more reliable for commuters everywhere.
What is Demand Forecasting in Public Transportation?
Demand forecasting predicts future passenger traffic patterns based on historical data, seasonal trends, and other influencing factors. These forecasts help transportation agencies anticipate demand spikes or declines, adjust service levels, and optimize route planning, ultimately ensuring that the system operates efficiently and cost-effectively.
Demand forecasting for public transportation involves understanding how many passengers will use different routes, at what times, and under various weather conditions. Accurate forecasts enable transport authorities to ensure that resources, such as buses, trains, and staff, are allocated optimally, ensuring the best service without unnecessary overheads.
Key Concepts of Demand Forecasting
Several methods can be used to forecast demand in public transportation. These include time series analysis, machine learning algorithms, regression models, and more. AI-driven tools, particularly those incorporating AI in demand forecasting, leverage historical data and demand forecasting tools to produce highly accurate predictions. These tools use complex algorithms to process vast amounts of data, improving over time as more data becomes available.
Forecasting and Demand Management: The goal of demand forecasting is not only to predict future passenger volumes but also to manage them effectively. By having a precise understanding of future demand, transportation authorities can better manage scheduling, fleet management, and customer experience.
AI-powered Demand Forecasting: AI-driven demand forecasting has revolutionized how transport companies manage their operations. AI in transportation uses advanced techniques like deep learning, reinforcement learning, and natural language processing to improve predictions and automate decision-making.
Autonomous Scheduling: AI agents can autonomously adjust schedules based on forecasting and planning, helping to improve efficiency and ensure that transportation services meet real-time demand fluctuations.
Traditional Way of Automating Demand Forecasting
Before AI-driven tools, demand forecasting was largely dependent on manual methods or simple mathematical models. These approaches often relied on historical data, such as ridership numbers from previous years, seasonal patterns, and static trends. Although useful, traditional methods often lack the flexibility and accuracy that AI-driven systems now provide.
Traditional demand forecasting involved experts reviewing past ridership data and predicting demand based on limited input variables. This often resulted in over-saturation of services during low-demand periods or insufficient services during peak hours. The consequences included inefficient transportation services, higher operating costs, and poor customer satisfaction due to overcrowded buses or delays.
Impact on Customers Due to Traditional Demand Forecasting Processes
For public transport customers, the traditional demand forecasting methods often led to:
-
Overcrowding: Public transit systems frequently struggle with overcrowded buses or trains during peak hours without the ability to adjust to dynamic demand. This led to an uncomfortable travel experience for passengers and dissatisfaction with the service.
-
Underutilized Resources: Traditional demand forecasting often resulted in the deployment of too many vehicles during off-peak hours, leading to unnecessary operational costs. This waste of resources directly impacted the financial health of the transportation system.
-
Lack of Real-time Adjustments: Due to rigid, traditional forecasting methods, passengers often faced delays or route changes that could not be anticipated or quickly adjusted.
The core of Agentic AI-driven demand forecasting lies in the AI agents that power real-time predictions and insights. These agents are responsible for analyzing vast datasets, identifying trends, and optimizing business strategies across industries. Click to explore how our Demand Forecasting AI agents can transform your business operations.
Akira AI: Multi-Agent in Action
The Agentic AI workflow in public transportation involves a team of AI teammates working in collaboration to optimize operations, predict demand, and enhance service efficiency. Each AI teammate plays a distinct role, with a Master Orchestrator overseeing and coordinating all activities to ensure seamless performance. Here's a breakdown of the key AI teammates and their roles in the workflow:
-
Master Orchestrator: The Master Orchestrator ensures smooth coordination between all AI teammates. It oversees the workflow, directing each teammate to collaborate effectively, prioritizing tasks, and ensuring that all components function harmoniously for optimal decision-making and service delivery.
-
Data Collection Agent: The Data Collection AI teammates gather data from diverse sources, including historical ridership data, weather forecasts, social media trends, ticket sales, and real-time traffic updates. These teammates ensure a comprehensive understanding of all factors that could affect demand.
-
Data Cleansing & Analysis AI Teammates: Data Cleansing AI teammates clean and organize the collected data, eliminating inconsistencies. The Analytics AI teammates then analyze this data, identifying patterns and anomalies to help detect demand fluctuations and other factors influencing public transportation.
-
Forecasting AI Teammates: Forecasting AI teammates utilize historical and real-time data to build predictive models that forecast future ridership. They account for events, seasonal patterns, and disruptions to accurately predict demand, ensuring transit services are prepared for future needs.
-
DSS AI Teammates: The DSS AI teammates transform forecasted demand into actionable insights. They recommend adjustments like increasing service frequency, rerouting vehicles, or deploying extra resources to meet changing demand patterns effectively.
Prominent Technologies in Agentic AI-based Demand Forecasting
The integration of AI has completely transformed demand forecasting, especially in public transportation systems. Key technologies employed in agentic AI-based demand forecasting include:
-
Machine Learning Algorithms: Machine learning (ML) models, such as decision trees, random forests, and neural networks, are key components in AI-powered workflows for transport demand forecasting. These models analyze historical demand data and environmental variables to predict future passenger numbers accurately.
-
Natural Language Processing (NLP): NLP allows AI agents to analyze textual data from news, social media, or customer feedback to detect patterns that affect demand, such as public events or weather forecasts.
-
Reinforcement Learning: Reinforcement learning enables AI agents to continuously improve their decision-making by learning from past actions and outcomes. This is especially valuable for AI in transportation, where dynamic adjustments are crucial.
-
Sensor Technology & IoT: Sensors embedded in vehicles or stations can feed real-time data into demand forecasting tools, improving predictions with live traffic, weather, and occupancy data.
AI agents revolutionize transportation by automating real-time traffic monitoring, optimizing traffic flow, and proactively resolving issues, ensuring safer and more efficient urban mobility.
Successful Implementations of AI Teammates in Public Transportation
Several public transportation systems have already embraced AI-powered demand forecasting with impressive results:
-
Santa Clara Valley Transportation Authority (VTA): California's VTA has successfully integrated a cloud-based AI solution for its transit signal priority (TSP) system. This system utilizes machine learning models to optimize traffic signals based on real-time bus locations, improving travel times by 18% to 20% on specific routes.
-
Project Luna by Arriva Rail London: Project Luna is an innovative AI-powered solution designed to improve accessibility for passengers who are deaf or hard of hearing. Developed by GoMedia, this system translates digital information into British Sign Language (BSL) and delivers it to passengers' smart devices. By providing real-time updates on station information and service disruptions, Project Luna significantly enhances the travel experience for these passengers, making public transport more inclusive.
-
Northern Train Operator's Automatic Passenger Counting: Northern, the second-largest train operator in the UK, has deployed an automatic passenger counting (APC) system that uses AI algorithms to count passengers on trains accurately. The APC technology has shown over 98% accuracy in passenger counting, which is crucial for operational planning.
-
Dutchess County Public Transit and Predictive Maintenance: Dutchess County Public Transit in New York has partnered with several agencies to test an AI-driven predictive maintenance solution that connects to bus sensors to detect mechanical issues before they become serious problems. This technology has reportedly reduced labour hours by over 50%, showcasing how AI can streamline maintenance processes and enhance fleet management efficiency.
Future Trends: How AI Agents Supersede Other Technologies
The future of public transportation lies in the increasing integration of AI-driven demand forecasting systems. As AI technology advances, we can expect:
-
Enhanced Demand Prediction Accuracy: AI agents will become increasingly proficient at predicting future demand precisely, reducing over-servicing and under-servicing issues currently plaguing many transport systems.
-
Full Automation in Scheduling and Dispatching: With more powerful agentic AI systems, transportation systems will achieve autonomous scheduling, where AI agents not only forecast demand but also optimize routes, schedules, and vehicle allocations without human intervention.
-
Hyper-Personalized Passenger Experiences: With AI agents capable of analyzing detailed passenger preferences and behaviour, public transportation could offer more personalized, flexible services, improving the commuter experience.
-
Driving Sustainability in Transit: As public transportation adopts AI-powered forecasting tools, they can optimize energy use, reduce emissions, and contribute to more sustainable cities by reducing congestion and improving traffic flow.
Conclusion: AI Agents for Demand Forecasting
AI-driven demand forecasting is revolutionizing the way public transportation systems operate. Through tools like Akira AI’s multi-agent system, agencies can accurately predict demand, optimize scheduling, and enhance customer satisfaction while improving operational efficiency. As the transportation industry continues to evolve with AI-powered workflows and autonomous scheduling, the future of public transport systems looks more dynamic, efficient, and sustainable. By embracing these innovations, public transit services can ensure they are well-prepared to meet future challenges and provide high-quality, responsive transportation options.
Next Steps
Talk to our experts about implementing Agentic AI systems for transit and exploring how industries can leverage agentic workflows and decision intelligence to optimize transit demand forecasting, automate operations, and improve efficiency.