Key Insights
Agentic AI-driven genomic data analysis transforms healthcare by providing faster, more accurate insights into genetic data, enabling precision medicine. Specialized AI agents optimize various tasks, from data preprocessing to predictive modeling, ensuring efficient and error-free analysis. This integration enhances disease prediction, treatment personalization, and accelerates advancements in drug discovery, ultimately leading to improved patient outcomes and proactive healthcare solutions.
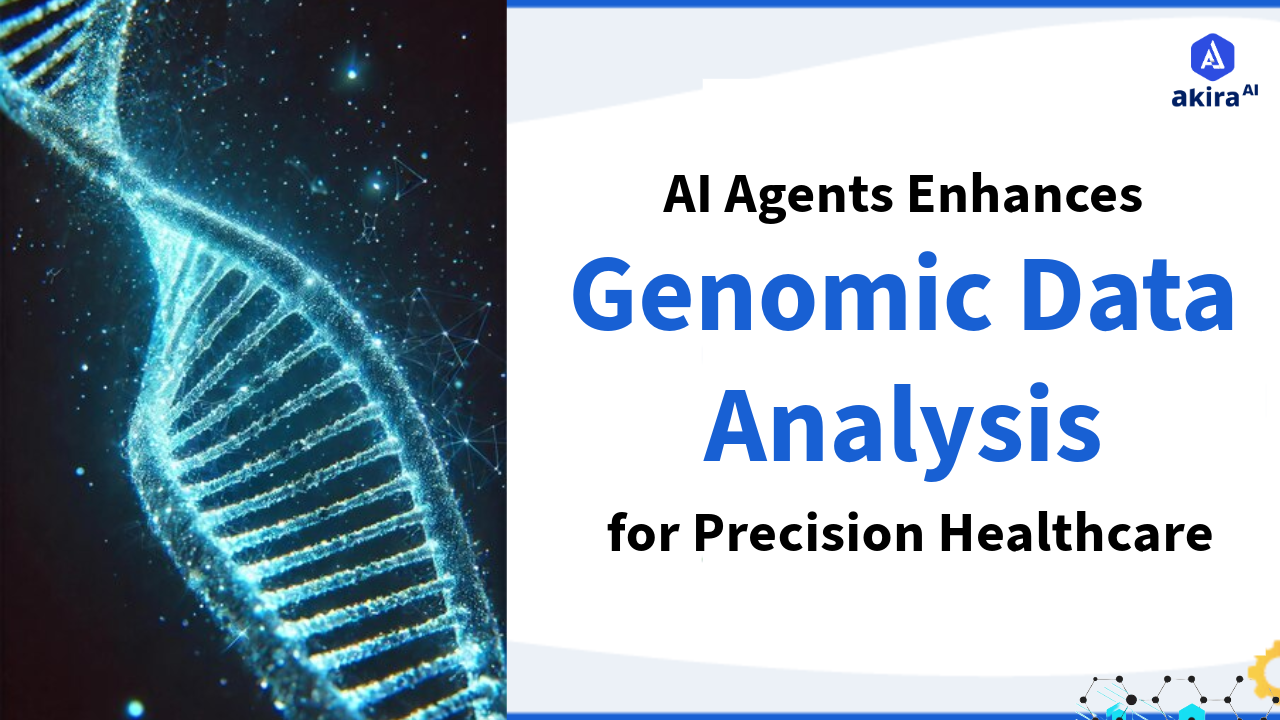
The rapid growth of genomic data is revolutionizing how healthcare professionals approach disease detection, treatment, and prevention. Traditional methods often fall short in managing the complexity and scale of these datasets, creating a critical need for innovative solutions. As highlighted in a recent Sify article, the integration of AI in genomics addresses these challenges by delivering transformative advancements in precision medicine.
Researchers can now decode complex DNA sequences faster and more accurately by leveraging AI agents such as predictive modelling and pattern recognition systems. This blog explores how Agentic AI-driven genomic analysis is reshaping healthcare, unlocking the future of personalized medicine.
What is Genomic Data Analysis?
Genomic data analysis is the systematic approach of examining an organism’s complete DNA sequence to understand its genetic structure, variations, and associated biological processes. It involves studying genes, their functions, interactions, and their role in health and disease. By examining genetic variations, this analysis identifies inherited traits, predicts disease risks, and informs the development of targeted treatments. Traditionally reliant on bioinformatics tools, genomic data analysis today is crucial in advancing fields like oncology, rare disease research, and personalized medicine by offering insights into the intricate mechanisms driving genetic expression.
Key Concepts of Genomic Data Analysis
Genetic Structure: This involves studying the complete DNA sequence to identify genes, their locations, and interactions with regulatory elements. Researchers explore how these genes work together to influence biological functions and traits. Understanding genetic structure is essential for identifying mutations linked to inherited diseases and complex health conditions.
Disease Prediction: By analyzing genetic variations, researchers can identify markers associated with an increased risk of diseases like cancer or diabetes. This information enables early detection and preventive measures, reducing the likelihood of severe outcomes. AI enhances this process by uncovering subtle patterns and providing more precise risk assessments.
Treatment Development: Genomic data helps create targeted therapies that cater to an individual's genetic profile, improving treatment effectiveness and reducing side effects. It also plays a pivotal role in pharmacogenomics, ensuring medications are tailored to a person’s genetic makeup. Additionally, genomic insights accelerate drug discovery by identifying specific molecular targets.
Bioinformatics Tools: These computational tools assist in organizing and analyzing large genomic datasets, identifying variants, and visualizing results. Tools like BLAST and genome browsers have been crucial for early genomic research. However, traditional methods struggle with today’s data volumes, prompting the adoption of AI for faster and more accurate analysis.
Traditional Way of Genomic Data Analysis
-
Manual Data Processing: Researchers manually curated and analyzed genomic data, often leading to slow, error-prone processes. This reliance on human effort increased the chances of inaccuracies and inconsistencies.
-
Limited Computational Power: Early bioinformatics tools could not handle complex datasets, restricting their capacity to identify intricate genetic patterns. This limited the scope of analysis and often led to oversimplified insights.
-
High Costs: Traditional genomic analysis requires expensive equipment, specialized tools, and highly trained personnel, making the process costly and less accessible for smaller institutions.
-
Lack of Scalability: As genomic datasets grew, traditional tools were overwhelmed and struggled to analyze large-scale data efficiently. This created bottlenecks in processing and delayed research outcomes.
-
Time-Intensive: The analysis process often took months due to the need for manual input and limited automation. This delayed critical healthcare decisions, particularly in time-sensitive scenarios like cancer diagnostics.
Challenges in Traditional Genomic Data Analysis
-
Data Overload: The rapid expansion of genomic datasets overwhelmed traditional tools, which lacked the computational power to process and analyze data efficiently. This resulted in slower research and analysis workflows.
-
Error-Prone Processes: Manual handling of repetitive tasks increased the likelihood of errors, undermining the accuracy and reliability of genetic data interpretation.
-
Resource Limitations: Traditional methods require costly equipment and specialized expertise, restricting the accessibility of genomic research to only well-funded institutions.
-
Slow Turnaround: Due to manual and time-intensive processes, traditional genomic analysis often delays critical diagnoses and treatment decisions, particularly in urgent cases like cancer.
-
Inadequate Predictive Models: Traditional bioinformatics tools struggled to capture intricate genetic patterns, leading to basic and often imprecise predictions that limited personalized care.
Impact of Traditional Methods on Customers in Genomic Analysis
The impact on customers due to the traditional way of genomic analysis can be significant, especially as the field of genomics evolves.
-
Longer Wait Times for Results: Genomic analysis methods often involve labor-intensive processes, such as manual data interpretation and slower sequencing technologies. This can lead to long wait times for patients and healthcare providers, delaying diagnoses and treatments.
-
Limited Accuracy and Precision: Traditional methods may rely on less sophisticated tools for analyzing genomic data, resulting in lower accuracy. Some genetic variations or mutations may be missed, leading to incomplete or inaccurate diagnoses, which could affect treatment decisions.
-
Higher Costs: The manual nature of traditional genomic analysis and the need for specialized equipment and personnel can result in higher costs for healthcare providers and patients. These costs might limit access to genomic testing for some individuals.
-
Lack of Personalized Insights: This may not be able to provide the level of personalized insight that modern methods offer. As a result, patients may not receive tailored treatment plans based on their unique genetic profiles, reducing the potential for effective, personalized care.
-
Inconvenience and Accessibility Issues: Traditional methods may require patients to travel to specific locations for testing or rely on physical paperwork, which can be inconvenient, especially for patients in remote areas or those with mobility issues. Additionally, the limited scalability of traditional approaches can restrict access to genomic testing for underserved populations.
Akira AI: Multi-Agents in Action
Fig1: Architecture Diagram of Genomic Data Analysis
Akira AI utilizes a sophisticated multi-agent system to enhance genomic data analysis, ensuring precise and efficient interpretation of complex genetic information. Each specialized agent, from data preprocessing to predictive modelling, autonomously handles specific tasks, optimizing the entire analysis pipeline. This integrated approach enables researchers and healthcare professionals to uncover genetic insights with accuracy, predict potential health risks, and provide personalized treatments, empowering better decision-making and accelerating advancements in genomics.
-
Data Preprocessing Agent: It prepares raw genomic data by cleaning, filtering, and normalizing it. This ensures the data is free from errors and inconsistencies, making it suitable for further analysis. By organizing data into a standardized format, the reliability of the analysis process is enhanced.
-
Pattern Recognition Agent: AI Agents analyze large genomic datasets to identify hidden patterns and correlations. They detect genetic variations linked to specific diseases or conditions using machine learning. This helps researchers uncover important genetic markers that can lead to more accurate diagnoses.
-
Predictive Modeling Agent: It creates probabilistic models that assess the genetic risk of developing certain diseases. Integrating genomic data with other factors provides insights into potential health outcomes, enabling early intervention and more personalized healthcare strategies.
-
Interpretation Agent: Agentic AI converts complex genetic data into understandable insights for healthcare providers. Translating technical genetic information into clear clinical recommendations ensures that doctors can make informed decisions. This agent makes genetic results accessible for improving patient care.
-
Visualization Agent: This agent creates visual representations of genetic data to simplify complex information. Interactive charts and graphs make identifying trends and relationships within the data easier. These visual tools enhance understanding and decision-making in genomic research and healthcare.
Prominent Technologies in the Space of Genomic Data Analysis
-
Machine Learning Algorithms: Power tasks such as variant classification, disease prediction, and biomarker discovery. Both supervised learning (e.g., pathogenic variant prediction) and unsupervised learning (e.g., clustering genetic profiles) are widely utilized for analyzing genomic datasets.
-
Deep Neural Networks (DNNs): Enable complex pattern recognition in genomic data. Techniques like Convolutional Neural Networks (CNNs) help analyze genetic sequences, while Recurrent Neural Networks (RNNs) excel at interpreting sequential DNA data for mutation detection.
-
Natural Language Processing (NLP): Extracts valuable information from unstructured textual data, such as scientific literature and clinical notes, aiding in genomic variant interpretation and decision-making.
-
Predictive Analytics Platforms: Leverage statistical and AI techniques to forecast disease risks and therapeutic outcomes based on genomic profiles, offering proactive healthcare strategies.
-
Genome Sequencing AI Tools: These tools automate sequencing workflows, identify genetic variants, and enhance the precision of gene mapping, making large-scale genomic studies more efficient.
-
Bayesian Networks: Use probabilistic models to handle uncertainties in genomic datasets and incorporate prior knowledge for better predictions and analyses.
A comprehensive platform for early disease detection, consolidating real-time insights and interactions into one seamless feed, accessible via mobile, web, or desktop app. Explore how our Agentic AI-powered system transforms healthcare with faster, more accurate diagnoses and improved patient outcomes
Future Trends: How AI Agents Supersede Other Technologies
-
Enable Real-Time Genetic Insights: Sophisticated AI technologies will process vast genomic datasets instantaneously, delivering actionable insights to clinicians and researchers in real-time.
-
Develop More Precise Predictive Models: Advanced algorithms will uncover intricate patterns within genomic data, enabling breakthroughs in disease prediction and personalized medical interventions.
-
Integrate Multi-Omics Data: AI will seamlessly combine genomics, transcriptomics, proteomics, and other omics data to provide a holistic view of patient health and enhance multi-dimensional research.
-
Create Personalized Medicine Protocols: Tailored therapeutic solutions based on an individual’s genetic makeup will become increasingly feasible through ongoing advancements in AI-driven genomic research.
-
Enhance Global Health Strategies: AI will contribute to global health by addressing regional genomic variations, enabling the development of localized treatments and a better understanding of genetic diseases worldwide.
-
Drive Ethical Genomic Advancements: Collaboration among researchers, regulatory bodies, and policymakers will ensure the ethical use of genomic data, fostering equitable access while safeguarding privacy.
Successful Implementations of AI Agents in Genomic Data Analysis
-
Cancer Genomics: Genomic data analysis identifies mutations specific to cancer, enabling the development of personalized treatment plans. This precision approach enhances the effectiveness of therapies, improving patient outcomes by targeting the exact genetic alterations causing the cancer.
-
Rare Disease Diagnostics: Genetic markers linked to rare diseases can be detected more rapidly through advanced analysis. Early identification of these markers ensures quicker diagnoses, leading to timely interventions that improve the prognosis for individuals with rare conditions.
-
Pharmacogenomics: Genomic insights predict how individuals will respond to specific medications. By analyzing genetic data, treatment plans can be optimized, reducing the risk of adverse drug reactions and improving the efficacy of prescribed drugs for each patient.
-
Hereditary Disease Prediction: Familial genetic patterns are analyzed to assess the risk of hereditary diseases. By understanding these patterns, individuals can be better informed about their potential risks and take preventive measures to manage inherited conditions.
-
Drug Discovery: Advanced genomic analysis expedites the identification of molecular targets for drug development. This accelerates the discovery of new treatments, allowing researchers to focus on compounds that are more likely to succeed in clinical trials.
-
Public Health Management: Genomic trends across populations are analyzed to inform healthcare policies and address disparities in health outcomes. Understanding genetic variations on a broader scale helps tailor public health interventions to improve health equity across different regions.
Conclusion: AI Agents for Genomic Data Analysis
AI-driven genomic data analysis is revolutionizing our understanding of genetics and its impact on human health. Researchers can unravel complex genomic patterns by employing advanced algorithms and intelligent agents, advancing precision medicine toward a highly personalized and proactive healthcare future. While challenges such as data quality, ethical considerations, and integration remain, collaborative efforts across disciplines are paving the way for equitable and transformative healthcare solutions. This synergy between AI and genomics holds the potential to redefine how we diagnose, treat, and prevent diseases on a global scale.
Next Steps