Key Insights
-
AI Agents Drive Efficiency: AI agents automate data processing and analysis, significantly speeding up medical research and improving accuracy in decision-making.
-
Personalized Healthcare Solutions: It enables tailored treatment plans and precision medicine by analyzing vast datasets, such as genomics and medical imaging.
-
Cost-Effective Innovation: By reducing manual effort and minimizing errors, AI agents lower operational costs, enhancing drug discovery, clinical trials, and patient care.
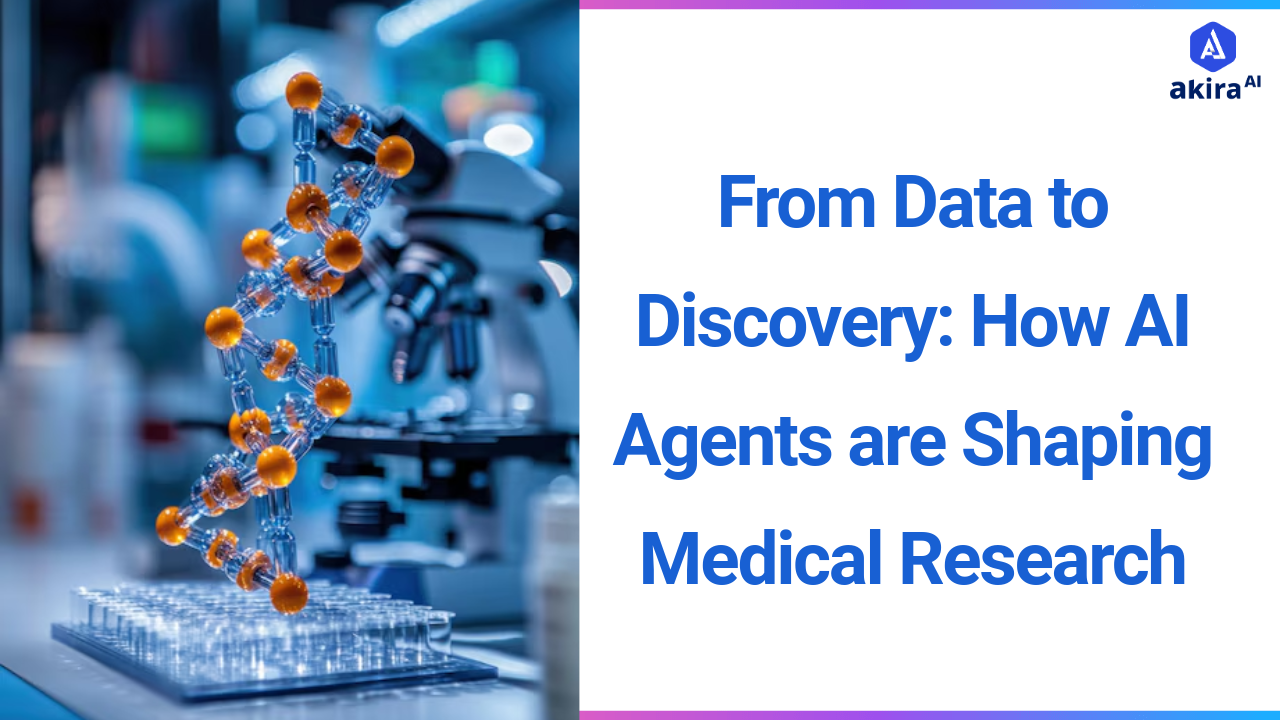
The healthcare sector is witnessing a transformative shift as AI agents redefine medical research and data analysis. With the explosion of medical data from clinical records, imaging, and genomics, traditional methods often fail to uncover actionable insights. AI agents—advanced algorithms capable of processing and analyzing vast datasets—are stepping in to bridge this gap.
From early disease detection and personalized treatments to accelerating drug discovery, AI agents are revolutionizing how healthcare professionals approach challenges. In this blog, we explore how these technologies drive innovation, improve patient outcomes, and set a new standard for efficiency and precision in healthcare. The future of medical research is here—and it’s powered by AI agents.
What is Medical Research and Data Analysis?
Medical Research and Data Analysis in Healthcare is studying and interpreting health-related data to improve patient care and medical understanding. It involves examining information from patient records, medical imaging, and health surveys to identify patterns, understand trends, and support decision-making.
This process is vital in diagnosing diseases, developing treatments, improving public health strategies, and enhancing healthcare outcomes. By carefully analyzing data, healthcare professionals gain insights that help create better approaches to treatment, prevention, and management of medical conditions, ultimately improving the quality of care provided to individuals and communities.
Key Concepts of Medical Research and Data Analysis
Agentic AI systems in medical research continuously adapt to real-time data, enabling dynamic, human-like decision-making. They provide accurate, context-sensitive insights that evolve with emerging medical trends and discoveries.
Data Collection and Integrity :Gathering accurate, comprehensive, and reliable data from diverse sources like patient records, clinical studies, and medical imaging ensures the foundation for meaningful insights.
Pattern Identification and Trend Analysis: Analyzing data to uncover patterns, trends, and correlations helps understand diseases, predict outcomes, and improve care strategies.
Outcome Evaluation: Measuring the effectiveness of treatments and interventions provides critical insights for enhancing patient care and medical practices.
Ethical Considerations: Maintaining patient confidentiality, ensuring data privacy, and adhering to ethical standards are vital for responsible research and analysis.
Insight Application: Transforming analyzed data into actionable strategies for disease prevention, treatment planning, and healthcare policy development drives improved outcomes and innovation.
Traditional Way of Medical Research and Data Analysis
Medical research and data analysis relied heavily on manual processes and human expertise. Clinicians and researchers manually collected and analyzed data from various sources such as patient records, lab results, and medical imaging. This approach was time-consuming, slow, and resource-intensive, requiring significant human effort to sift through structured and unstructured data. The manual nature of the process also introduced a higher risk of human error, such as misinterpretation or oversight of essential details.
As healthcare data grew in volume and complexity, traditional methods struggled to keep up, making it increasingly difficult to extract insights quickly and efficiently. Moreover, these methods were limited in scalability, requiring more personnel and resources to handle larger datasets and often delayed insights that could have informed timely interventions. This highlighted the need for more advanced, automated solutions to address the challenges posed by the evolving demands of modern healthcare.
The future of medical research is not just in data, but in the intelligence that interprets it. With AI agents at the forefront, we are witnessing a revolution that empowers healthcare professionals to make faster, more accurate decisions—turning complex data into life-saving insights.
Impact on Customers Due to Traditional Way of Medical Research and Data Analysis
Due to the limitations of traditional methods, customers (patients and healthcare organizations) face several challenges:
-
Slow Diagnostics: Manual analysis of medical data results in delays in diagnosis, which can affect patient outcomes.
-
Limited Personalization: Traditional research cannot often provide personalized treatment recommendations based on genetic and clinical data.
-
Higher Costs: Manual data analysis's labour-intensive nature increases operational costs, particularly in drug development and clinical trials.
-
Increased Human Error: Traditional methods are prone to human error, leading to misdiagnoses, ineffective treatments, and incorrect conclusions in research.
Akira AI: Multi-Agents in Action
The Akira AI multiagent system revolutionizes medical research and data analysis by orchestrating specialized agents to tackle various complex tasks. Below is a detailed description of the architecture, process flow, and agent roles contextualized to healthcare applications.
Fig1: Architecture Diagram of Medical Research and Data Analysis
-
Data Collection Automation: The Data Collector Agent efficiently gathers diverse datasets from electronic health records (EHRs), genomic databases, medical imaging, and IoT devices, streamlining the data acquisition process and reducing manual effort.
-
Data Quality Enhancement: The Data Cleaner and Transformer Agents ensure data accuracy by removing duplicates, filtering irrelevant information, and standardizing formats, creating a reliable and interoperable dataset for analysis.
-
Advanced Analysis for Early Insights: The Predictive Analytics and Diagnostic Analysis Agents apply machine learning to forecast disease outcomes and detect anomalies in medical data, providing early insights for better decision-making.
-
Tailored Decision Support: The Decision Support and Recommendation Agents synthesize analyzed data to offer customized recommendations, such as treatment options or research directions, helping clinicians and researchers make informed, timely decisions.
-
Real-Time Collaboration and Workflow Management: The Communication Agent facilitates global research team coordination, enabling seamless task management, real-time updates, and efficient collaboration to address challenges and accelerate progress.
Prominent Technologies in the Space of AI Agents for Medical Research and Data Analysis
Several technologies are powering AI agents in healthcare, each contributing to different facets of medical research and data analysis:
-
Machine Learning (ML): ML models are capable of identifying patterns and making predictions based on large datasets, aiding in disease diagnosis and predicting treatment outcomes.
-
Deep Learning: Deep learning techniques enhance the accuracy of medical imaging analysis, enabling early detection of conditions like tumours and heart disease.
-
Natural Language Processing (NLP): NLP extracts insights from unstructured data sources such as medical records and research papers, helping researchers and clinicians gain valuable knowledge from diverse datasets.
-
Edge Computing: Edge computing allows for processing medical data closer to its source (such as wearable devices), enabling real-time decision-making and reducing latency.
-
Blockchain: Blockchain ensures the secure and transparent sharing of medical data across organizations, fostering collaboration and privacy in research.
AI agents optimize genomic data analysis, paving the way for personalized treatments and faster, more accurate disease predictions. Click here to read more.
How AI Agents Supersede Other Technologies
AI agents offer several advantages over traditional methods and other technologies in medical research and data analysis:
-
Speed and Efficiency: Unlike manual processes that take days or weeks, AI agents can process large datasets in real time, offering instantaneous insights.
-
Accuracy and Precision: AI agents continuously learn from data, improving their predictive capabilities and minimizing human error, leading to more accurate diagnoses and research outcomes.
-
Scalability: AI agents can scale effortlessly, handling vast amounts of data from various sources, including genomic databases, clinical records, and medical imaging systems.
-
Cost Reduction: AI agents automate repetitive tasks, which reduces the need for manual labour and decreases operational costs, particularly in drug development and clinical trials.
-
Predictive Analytics: AI agents use advanced predictive models to foresee future trends, such as disease outbreaks, patient deterioration, or treatment effectiveness, enabling proactive interventions.
Successful Implementations of AI Agents in Healthcare
-
Drug Discovery and Development: Insilico Medicine uses AI-driven platforms to simulate the behaviour of molecules and predict their interactions, significantly accelerating the drug discovery process. Their AI system helped identify a potential drug candidate for fibrosis in just 46 days, a task that would have typically taken years using traditional methods.
-
Genomic Research and Precision Medicine: IBM Watson for Genomics uses AI to analyze genetic data and identify mutations linked to diseases like cancer. It has been applied to help oncologists match patients with the most appropriate treatments based on their genetic profiles, improving the outcomes of personalized cancer therapies.
-
Medical Imaging: Google's DeepMind has developed AI systems that analyze medical images such as retinal scans, MRIs, and CT scans with remarkable accuracy. DeepMind’s AI technology has been used in the UK’s National Health Service (NHS) to detect over 50 types of eye diseases and to diagnose certain forms of cancer earlier than traditional methods.
-
Clinical Trials: TrialX, an AI platform, optimizes participant selection for clinical trials by analyzing vast datasets to identify suitable candidates. By streamlining the recruitment process and predicting dropout risks, TrialX ensures more efficient and effective clinical trials with diverse participant representation.
-
Disease Surveillance: BlueDot, a global health AI company, uses AI to track infectious disease outbreaks. In 2019, BlueDot’s AI system detected the early spread of COVID-19 in Wuhan before the World Health Organization (WHO) issued an official alert, allowing governments and health organizations to respond more rapidly.
Conclusion: AI Agents for Medical Research
AI agents are transforming healthcare medical research and data analysis by automating complex tasks, providing real-time insights, and improving decision-making. By superseding traditional methods, AI agents offer a more efficient, accurate, and cost-effective approach to tackling the challenges faced by healthcare professionals and researchers. As AI continues to evolve, its potential to revolutionize healthcare research is boundless, paving the way for personalized, data-driven solutions to improve patient outcomes and streamline operations.
Next Steps in Healthcare
Talk to our experts about implementing Agentic AI-driven system in medical research, optimizing data analysis, and accelerating discoveries. By leveraging AI Agents, researchers can uncover valuable insights from vast datasets, driving innovation in healthcare and transforming complex data into actionable findings. This allows the medical field to progress faster, enabling breakthroughs that improve patient outcomes and treatment methodologies.