Key Insights
The use of AI agents in managing engineering data within manufacturing allows for greater precision and adaptability. By automating key engineering processes, manufacturers can achieve higher throughput, improve product quality, and respond more quickly to market demands. This not only enhances efficiency but also fosters innovation and opens new possibilities for product development.
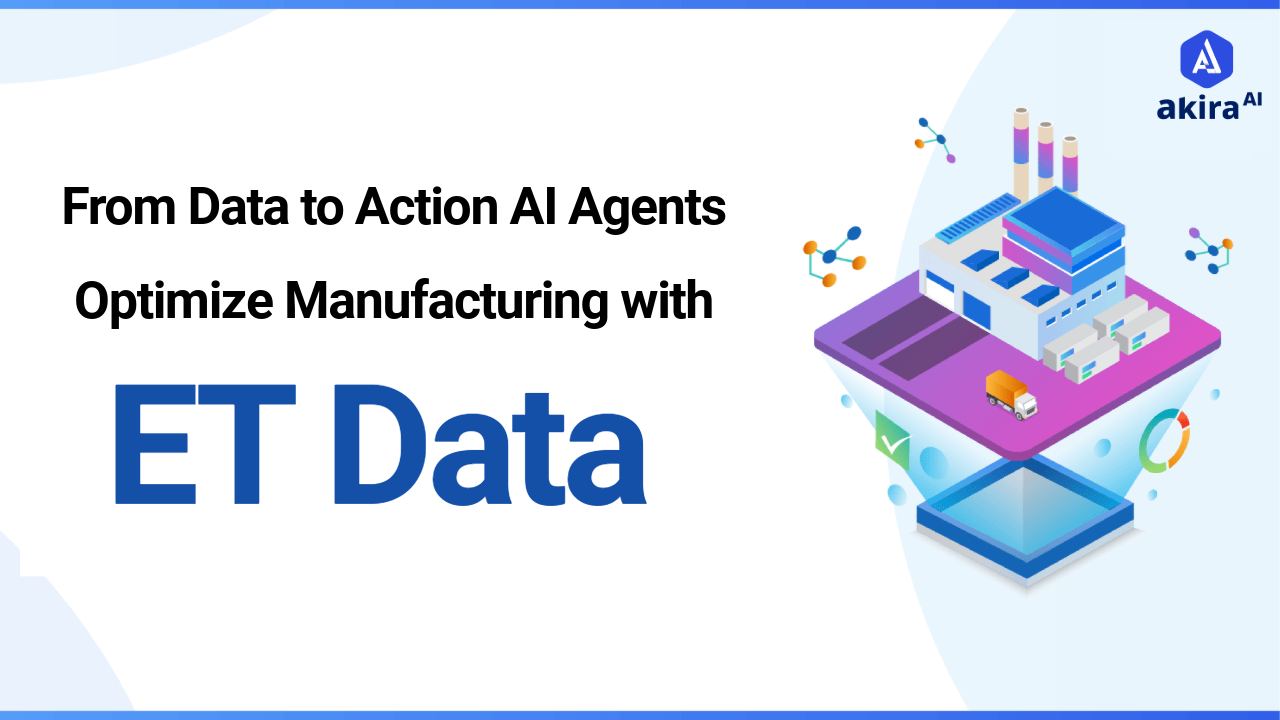
The world of manufacturing automation is evolving at lightning speed, and AI agents are at the forefront of this transformation. These intelligent systems are altering the use of engineering technology data and transforming it into actionable information in real-time. From increasing production output to optimizing processes to making forecasts for maintenance, these autonomous agents are paving the way for smart manufacturing.
Because of their flexibility, understanding of problems, and capacity to solve problems independently, the agents optimize procedures and achieve unparalleled efficiency. In this blog, we will explore how these innovations drive the next wave of manufacturing excellence and reshape the industry.
What is ET Data in Manufacturing?
Engineering technology (ET) data refers to the vast amounts of data generated during the design, production, and operational phases of manufacturing. Such information can include the designs of the products, the manufacturing schedule, the efficiency of plant and machinery, and perhaps other live data from machinery and sensors.
ET data is crucial in optimizing manufacturing processes, improving product quality, and maintaining equipment. Using this data can lead to informed decisions and high productivity levels with less expenditure.
A Brief Overview of Automated ET Data in Manufacturing
Automated ET data uses intelligent systems and technologies like AI agents to collect, analyze, and act upon engineering technology data without significant human intervention. This enables manufacturers to control the various processes efficiently, improve interconnectivity, and execute interventions as they happen. Automating ET data management is gaining paramount importance as manufacturing processes become more sophisticated and frequently characterized by large amounts of data.
Autonomous agents in ET data are self-governing and constantly adapting by acquiring new knowledge and making decisions independently in real-time. These agents can interact with each other and the broader manufacturing ecosystem, seamlessly integrating smart systems across the production floor. They can handle tasks ranging from routine maintenance checks to complex predictive analytics.
Traditional vs. Agentic AI ET Data in Manufacturing
Aspect |
Traditional ET Data |
Agentic AI-Based ET Data |
Data Processing |
Manual data entry and analysis |
Automated data processing with AI agents |
Decision-Making |
Dependent on human interpretation and action |
AI-driven autonomous decision-making. |
Error Handling |
Human-driven error correction |
AI agents can identify and correct errors autonomously |
Real-Time Analytics |
Limited real-time insights |
Real-time analytics and proactive responses |
Operational Efficiency |
Prone to delays due to manual processes |
Continuous optimization and dynamic workflows |
Adaptability |
Static systems with limited learning ability |
Systems that learn and adapt over time |
Akira AI Multi-Agent in Action
Akira AI is an advanced AI agent system that enhances manufacturing automation using a multi-agent framework. At the core of this system is a master orchestrator, which controls different types of specialized AI agents and is subordinate to them. Every agent is programmed to perform certain duties, for instance, health of equipment, analysis of production data of products, and identification of probable maintenance requirements. Below is an example of how this multi-agent system operates:
-
Master Orchestrator: The Master Orchestrator begins by collecting real-time data from sensors across the production floor. It assigns tasks to various agents based on the current operational requirements.
-
Predictive Maintenance Agent: The Predictive Maintenance Agent uses machine learning algorithms to analyze sensor data and predict potential equipment failures. It can autonomously schedule maintenance or alert human operators to take action.
-
Quality Control Agent: The Quality Control Agent monitors the production line in real-time to ensure product quality standards are met. If it detects anomalies, it can then set off a string of problem-solving operations, which include changing settings on a particular machine or halting production.
-
Production Scheduling Agent: The Production Scheduling Agent uses the production information obtained by the Predictive Maintenance Agent and Quality Control Agent to make the most efficient use of resources.
-
Energy Management Agent: The Energy Management Agent analyzes energy consumption patterns and suggests adjustments to reduce costs while maintaining production output.
-
Logistics Agent: The Logistics Agent manages material handling, tracking inventory, and ensuring that the right materials are available at the right time to avoid production delays.
Use Cases and Application of ET Data in Manufacturing
-
Predictive Maintenance for Preventing Failures: AI agents analyze machine data to determine the likelihood of equipment failure, minimizing unplanned downtime and maintenance expenses. For example, these agents can predict when a part will fail based on vibration, temperature, and other sensor data, scheduling maintenance in advance.
-
Automating Quality Control Inspections Efficiently: Autonomous agents can inspect products on the assembly line for defects, ensuring consistent quality without requiring manual inspections. This reduces human error and increases throughput.
-
Streamlining Supply Chain and Production: These agents can predict the likely demand and make changes to the production calendar to have the right number of materials in the right quantities at the right time, greatly reducing the need for inventory.
-
Maximizing Energy Efficiency and Sustainability: By monitoring energy usage patterns, these agents can adjust processes to minimize energy consumption, reducing costs and environmental impact.
-
Adapting Operations with Real-Time Decisions: Agentic AI allows real-time adjustments to production schedules and workflows, ensuring manufacturers can adapt to changing conditions without human intervention.
Operational Benefits of ET Data
Boosting Productivity with AI Agents: These agents can perform tedious tasks such as data gathering, monitoring for maintenance indication, and quality control so that human operators can manage more complex things. This leads to a 30% increase in productivity as processes become more streamlined and efficient.
Enhancing Efficiency through Automation: Agentic AI can enhance manufacturing effectiveness by 25% by processing real-time data and improving work processes. Tasks such as material flow, energy application, and timing are automated, thus minimizing staggers and log jams.
Minimizing Downtime with Predictive Maintenance: Autonomous agents predict equipment failures before they occur, enabling scheduled maintenance that prevents costly downtime. Studies suggest that predictive maintenance reduces downtime by up to 40%, increasing machine availability.
Achieving Cost Savings with Automation: Automation through these agents can significantly reduce operational costs. For instance, AI-driven energy management can reduce energy consumption by up to 15%, and quality control can lower defect rates, reducing waste and rework costs.
Scaling Operations with Agentic AI: The multi-agent systems can scale quickly to accommodate increased production demands. As the system learns from more data, it becomes more efficient at managing increasingly complex workflows, leading to faster production cycles.
Technologies Transforming ET Data in Manufacturing
-
Machine Learning Driving Continuous Improvement: Autonomous agents continuously learn from data, improving their predictions and decision-making over time.
-
Industrial IoT (IIoT) Connecting Data and Devices: Sensors and connected devices feed real-time data into AI systems, providing a comprehensive view of operations.
-
Robotic Process Automation (RPA) for Efficiency: AI agents automate repetitive tasks, improving efficiency and accuracy.
-
Large Language Models (LLMs) Enhancing Understanding: Used in natural language processing, LLMs help these agents understand and process unstructured data, like production reports and maintenance logs.
-
Cloud Computing Supporting Scalable AI Systems: Facilitates the storage and processing of large amounts of data, enabling such agents to work with scalable infrastructure.
The Future Trends of Automated ET Data in Manufacturing
-
Greater Adoption of Autonomous Systems: By 2025, it is predicted that autonomous systems will drive 80% of manufacturing tasks.
-
Enhanced Integration with IoT: The integration of AI agents with Industrial IoT will create smarter and more efficient manufacturing ecosystems.
-
Advanced Predictive Analytics: AI systems will continue to advance in their ability to predict equipment failures and optimize production schedules, reducing downtime and costs.
-
Human-AI Collaboration: As AI systems take over routine tasks, human workers will shift to more strategic roles, fostering collaboration between humans and machines.
-
AI Enabling Mass Customization at Scale: Autonomous agents will allow for more personalized and flexible manufacturing processes, enabling mass customization at scale.
Conclusion: ET Data in Manufacturing with Agentic AI
As AI agents continue to advance, the transformation of engineering technology data in manufacturing is accelerating at an extraordinary pace. Agentic AI is providing manufacturers with unparalleled opportunities to optimize every facet of their operations. From predictive maintenance that prevents unplanned downtime to real-time decision-making that allows for agile responses to production challenges, the multi-agent system enhances efficiency and drives smarter workflows.
By embracing these technologies today, manufacturers are positioning themselves to not only stay competitive but to lead in a rapidly evolving industry. With AI agents automating routine tasks and streamlining complex processes, businesses can achieve higher productivity, reduce operational costs, and enhance product quality.
Explore Further