Key Insights
Agentic AI-based Digital Twin Simulations revolutionize manufacturing by replacing traditional, human-driven processes with autonomous agents that optimize workflows in real-time. Unlike traditional systems, which often require manual intervention, AI-driven simulations offer enhanced adaptability, predictive maintenance, and efficient decision-making, leading to increased operational efficiency and cost savings. By continuously analyzing and adjusting systems, Agentic AI ensures a more proactive, dynamic approach to manufacturing operations, paving the way for smarter, more automated production.
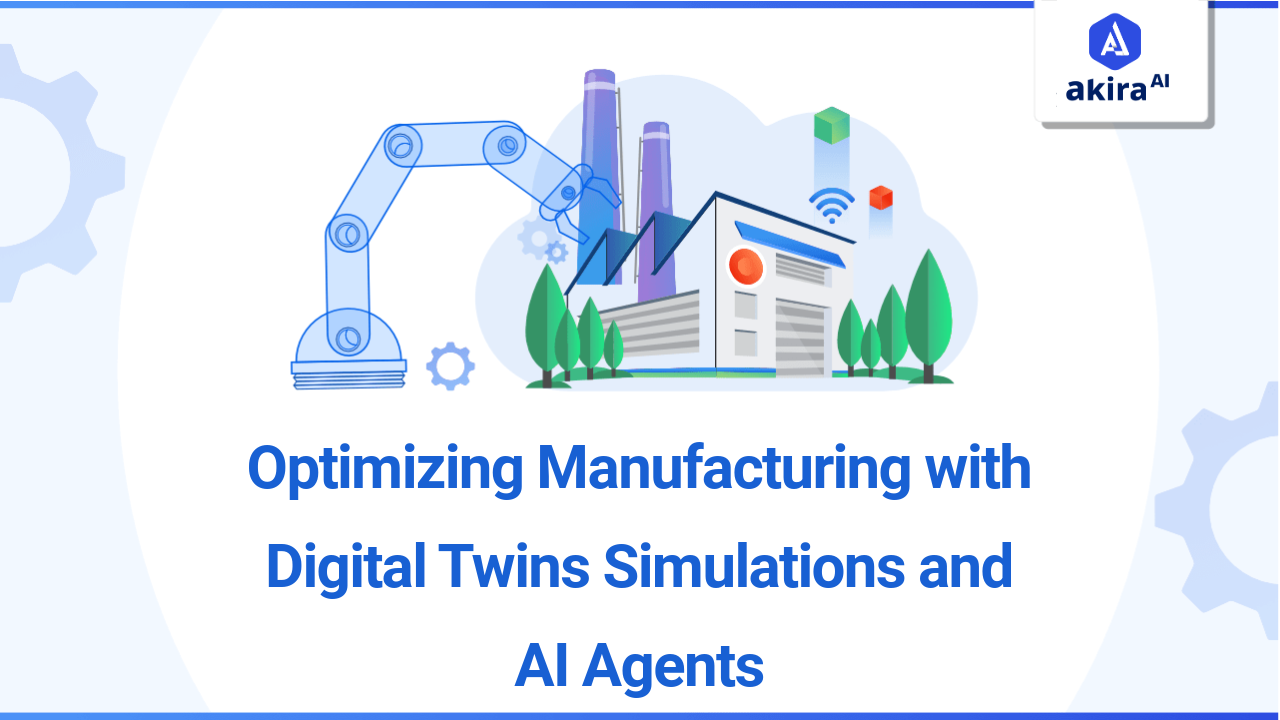
Imagine a world where machines don’t just replicate reality but actively improve it. This is the promise of digital twin simulations, which have already begun to revolutionize manufacturing. These digital replicas of physical systems allow real-time monitoring and predictive analysis, enhancing operational decision-making. However, the real magic happens when agentic AI is integrated. By integrating this technology into these simulations, the models become active structures that can control their environment, make decisions, and optimize reasoning mechanisms and processes in real-time. This combination brings new levels of automation, efficiency, and precision previously unimaginable. Manufacturers can anticipate challenges, optimize resources, and drive productivity, paving the way for a future where manufacturing processes are smarter, faster, and more cost-effective.
What are Digital Twin Simulations?
Digital twin simulations refer to developed computer models that mimic real-life systems, processes, or assets. These models are trained using data from the actual real-world models through sensors, ensuring that manufacturers can learn how their real models perform and their state all the time. Related to the concept of heightened manufacturing, digital twin technology helps control the processes, recognize potential failures, and improve the processes taking place within a business. Using virtual environments to model specific situations makes manufacturing operations more efficient, reduces total time not operating, and provides better knowledge. This capability also makes it possible to prevent equipment failure and better organization of available resources for improved efficiency.
A Brief Overview of Agentic AI-based Digital Twin Simulations in Enhanced Manufacturing
Agentic AI-based digital twin simulations represent a more advanced approach to traditional digital twin technology. In this model, autonomous agents powered by Artificial Intelligence take charge of various tasks and decision-making processes within the simulation. These agents are developed to act autonomously or in coordination with other agents to perform tasks without extensive control from human beings. The benefit of agentic AI is that it makes the digital twin simulation more flexible and better tuned to respond to new data.
In a multi-agent system, the agents perform specialized tasks in the simulation by collecting data, analyzing the data, predicting the outcome, and optimizing the data. The core decision ability enhances the autonomy of the systems and decreases the amount of human intervention to augment the existing decision systems. This system offers greater flexibility and efficiency, as each agent can focus on a particular task while interacting with others to improve the overall performance of the digital twin.
Traditional vs. Agentic AI-based Digital Twin Simulations
Aspect |
Traditional Digital Twin Simulations |
Agentic AI-based Digital Twin Simulations |
Automation |
Limited automation and human intervention required |
Autonomous agents handle tasks with minimal human input |
Real-time Adaptability |
Limited ability to adapt to real-time data changes |
Dynamic adaptation based on real-time data inputs |
Decision-Making |
Human-driven decisions based on data analysis |
AI agents make decisions autonomously, enhancing speed and accuracy |
Complexity of Workflow |
Single-agent or manual workflows |
Multi-agent systems handle complex, distributed tasks |
Operational Efficiency |
Efficiency gains through analysis, but manual intervention required |
AI agents continuously optimize systems, improving efficiency and productivity |
Cost Efficiency |
Requires human oversight for process changes |
Reduces the need for human intervention, improving cost efficiency |
Predictive Maintenance |
Maintenance based on static models or manual triggers |
Predicts maintenance needs proactively, minimizing downtime |
Akira AI: Multi-Agent in Action
Akira AI utilizes a multi-agent system, where different AI agents collaborate under the guidance of a Master Orchestrator. Each agent in this system is programmed to perform special tasks, including data gathering, processing, and fine-tuning. The key agents used by Akira AI include:
-
Master Orchestrator: The Master Orchestrator serves as the central command unit that oversees the coordination of all agents in the system. This ensures that the tasks are correctly allocated, agents interact well, and the system is functioning well and not hampered.
-
Data Collection Agent: The Data Collection Agent gathers real-time data from numerous sensors and production lines to get essential parameters concerning machines’ state, output rates, and conditions.
-
Data Processing Agent: After data collection, the Data Processing Agent analyses the raw data, removes unwanted noise, and makes conclusions. It includes tasks that clean data for analysis and enunciate trends, anomalies, and issues to which focus should be directed within the data.
-
Prediction Agent: The Prediction Agent incorporates complex decision-making techniques including statistical models and artificial intelligence to anticipate potential problems related to equipment breakdowns, faulty products, or bottlenecks. Analyzing historical and real-time data helps predict maintenance needs or areas where performance could decline.
-
Optimization Agent: As informed by the Prediction Agent, the Optimization Agent improves system performance by fixing anything in the system, be it a process, timetable, or mechanical parts that non-linearly cause inefficiency. This agent is very important in managing waste, time, and resources required in the production process.
-
Action Agent: The Action Agent has the role of making the appropriate changes within the table. These include adjusting some of the production parameters, triggering some form of maintenance, or modifying the processes in order to possibly get better results. The agent takes proactive measures based on the insights generated by the other agents
Uses Cases of Digital Twim Simulations
Predictive Maintenance: AI agents enable real-time monitoring of equipment and systems, predicting when maintenance is needed. This minimizes unplanned downtime and reduces maintenance costs.
Production Optimization: Agentic AI can virtually model and review production procedures, which enhances the efficiency of transitions between phases and negates congestion factors.
Supply Chain Management: These agents simulate different supply chain scenarios, improving inventory management and ensuring just-in-time production.
Quality Control: AI agents continuously monitor the production process for quality assurance, detecting defects early and minimizing waste.
Energy Management: Digital twin simulations with autonomous agents help manufacturers optimize energy consumption, leading to cost savings and environmental sustainability.
Operational Benefits of Digital Twin Simulations
-
Boosting Productivity with AI Automation: AI-powered systems can boost productivity by up to 40% in some cases, as automation and process optimization reduce manual interventions and streamline production cycles.
-
Cutting Downtime Through Predictive Maintenance: Predictive maintenance, an integral part of digital twin simulations, can reduce downtime by up to 50%. Adopting autonomous agents handling a significant portion of work accelerates this benefit.
-
Driving Efficiency Through Real-Time Optimization: With real-time adjustments, AI systems can enhance process efficiency by 25-35%, reducing resource consumption, less waste, and optimizing workflows.
-
Slashing Costs with Smarter Operations: Operational cost reductions of 15-25% are common due to better resource allocation, fewer errors, and predictive maintenance capabilities.
-
Empowering Decision-Making with AI Insights: AI agents enable faster decision-making, cutting decision time by 30-40% and offering real-time insights and automated analysis, leading to more proactive and strategic actions.
Technologies Transforming Digital Twin Simulations in Manufacturing
-
IoT Sensors for Real-Time Data: The connectivity of IoT sensors also contributes to constant feedback so that simulations are real-time, especially for dynamic manufacturing. This helps to make the working of systems manageable and easily adjustable in real time.
-
Edge Computing for Faster Decisions: Edge computing entails the processing of data at the edges, making it faster to arrive at decisions and enhancing the overall system’s throughput for fast responses in manufacturing lines.
-
Machine Learning for Enhanced Prediction: Machine learning algorithms improve the accuracy of predictive models, which are critical for forecasting potential issues, optimizing operations, and increasing manufacturing reliability.
-
5G Connectivity for Real-Time Communication: 5G connectivity provides faster, more reliable data transmission, which is crucial for enabling real-time simulations, rapid decision-making, and the smooth operation of interconnected systems across manufacturing processes.
The Future of Automated Digital Twin Simulations in Enhanced Manufacturing
-
Full Automation of Manufacturing Processes: Shortly, most manufacturing operations will be fully automated, with autonomous agents taking on most tasks, eliminating manual interventions and boosting production efficiency to unprecedented levels.
-
Advanced AI Agents for Complex Tasks: As these agents continue to evolve, they will gain the ability to handle increasingly complex tasks within digital twin simulations, including advanced decision-making, optimization, and autonomous system control.
-
Deeper Integration of Emerging Technologies: Digital Twin Simulations will become more integrated with emerging technologies like AI, 5G, and blockchain, providing enhanced performance, better security, and more efficient operations across the manufacturing industry.
-
Customization through Generative AI: With the rise of generative AI, Digital Twin Technology will allow for greater customization of products and manufacturing processes, tailoring solutions based on real-time data, customer needs, and production capabilities.
-
Wider Industry Adoption and Expansion: As the benefits of Digital Twin Simulations become increasingly clear, adoption will expand across industries, driving smarter, more efficient, and sustainable manufacturing practices worldwide.
Conclusion: Digital Twin Simulations in Enhanced Manufacturing
The adoption of agentic AI with Digital Twin Simulations has brought this change within the manufacturing industry and presents a large competitive advantage to companies. Manufacturers should, therefore, seek to incorporate AI agents into their work schemes to improve productivity as well as minimize oftentimes disruptive downtimes. These technologies facilitate timely decision-making and help prevent bottlenecks, making production management and control possible promptly at every phase of production. With the increased progression of agentic AI and its ongoing integration with digital twin technology, the capacity for maximizing outputs increases exponentially in terms of increased productivity and efficiency, as well as cutting costs on resources. This collaboration of digital and intelligent technologies will undoubtedly play a pivotal role in shaping the future of manufacturing, ensuring that industries remain adaptable, innovative, and ahead of the curve in an increasingly automated world.
Explore More About