Key Insights
-
Smarter Automation – Enables decision-making with minimal human input, boosting efficiency.
-
Real-Time Optimization – Uses data-driven insights for proactive problem-solving.
-
From retail to healthcare, agentic automation is transforming industries, with growing focus on ethical AI governance and human-AI collaboration.
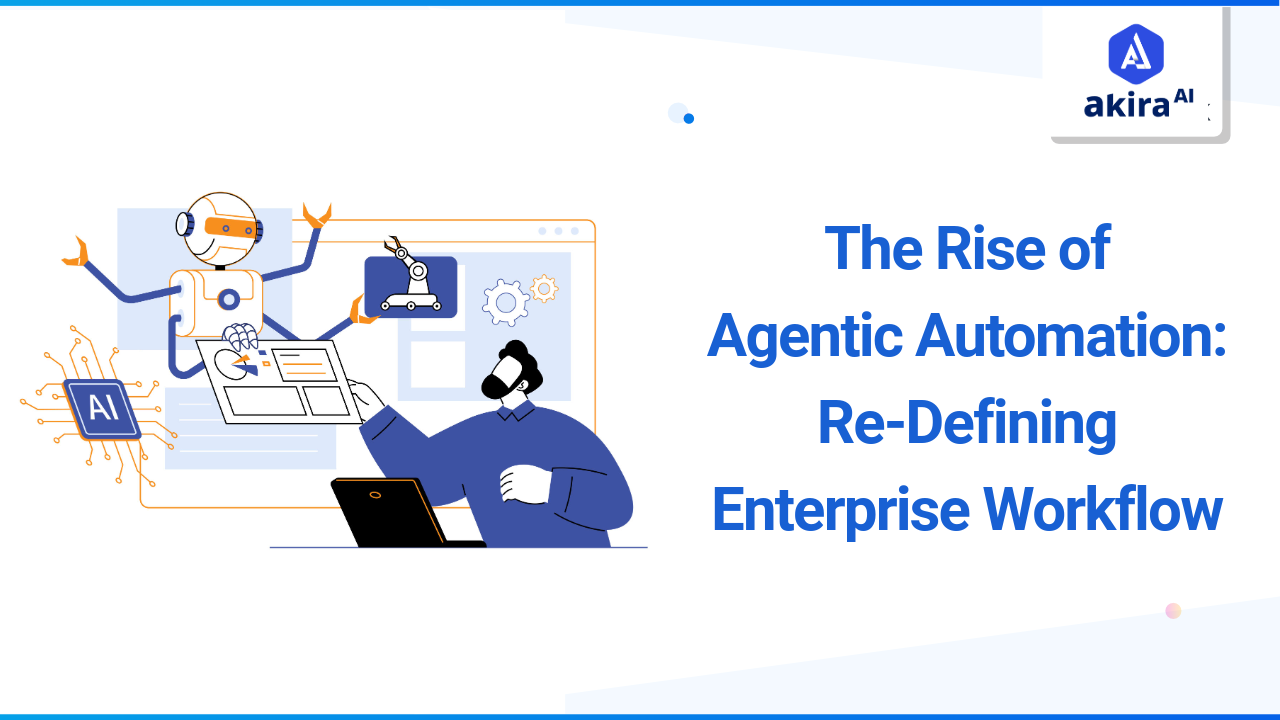
A Leading retail chain struggled with inventory mismanagement, long checkout lines, and inconsistent customer service. Despite using traditional automation tools, inefficiencies persisted, leading to lost sales and frustrated customers.
Everything changed when a retail chain implemented Agentic AI—an intelligent system capable of real-time decision-making, predictive analytics, and autonomous task execution.
This transformation showcases how AI Agents is redefining business efficiency. In this blog, we’ll explore how businesses across industries can harness the power of Agentic AI to unlock unprecedented growth and innovation.
Understanding Agentic Automation
At its core, agentic automation involves AI agents that operate with a high degree of autonomy. These agents can observe their environment, learn from data, and adjust their behavior to achieve specific goals without constant human oversight. This capability is a departure from traditional automation systems, which are typically limited to repetitive, rule-based tasks involving structured data.
Core Mechanisms of Agentic Automation
Autonomy: Agentic automation reduces human involvement by allowing AI agents to make decisions and execute tasks on their own, improving operational efficiency and reducing the risk of errors.
Adaptability: These AI agents are designed to respond to changing business conditions and unexpected events, ensuring continuity and flexibility in dynamic environments.
Intelligence: By using advanced AI models, such as large language models (LLMs), agentic automation enables real-time data analysis and decision-making, ensuring relevant, context-driven actions.
Integration: Platforms enable seamless integration of agentic automation into existing systems, enhancing operational workflows without disrupting current infrastructures or processes.
Challenges of Traditional Automation and Its Impact on Customers
While traditional automation has streamlined business operations, it is not without its limitations. Rule-based automation systems are constrained by pre-defined logic, making them inherently inflexible in handling complex, dynamic scenarios. These limitations have far-reaching consequences for both enterprises and their customers.
-
Lack of Adaptability and Context Awareness: Traditional automation operates on rigid workflows, requiring manual intervention whenever deviations occur. This rigidity often leads to inefficiencies in customer-facing processes, such as customer service chatbots failing to comprehend nuanced queries or process exceptions.
-
High Dependency on Human Oversight: Since traditional automation cannot independently resolve unexpected scenarios, human intervention is frequently required, resulting in increased operational costs and delays in service resolution. This negatively impacts customer satisfaction, as response times are prolonged.
-
Inability to Scale Dynamically: Static automation systems struggle to accommodate fluctuating demands. In industries like e-commerce or financial services, where demand surges during peak periods, traditional systems may fail to scale efficiently, leading to bottlenecks and degraded customer experiences.
-
Limited Decision-Making Capabilities: Without AI-driven contextual analysis, traditional automation cannot make data-driven decisions in real time. For instance, legacy fraud detection systems often rely on predefined thresholds rather than adaptive machine learning models, leading to higher rates of false positives and negatives.
-
Impersonal Customer Interactions: Many traditional automation solutions lack advanced natural language processing (NLP) capabilities, making customer interactions feel robotic and transactional rather than personalized. This impersonal approach diminishes customer engagement and retention.
Strategic Implementation of Agentic Automation in Enterprise Systems
Integrating agentic AI into an organization requires a well-structured approach to ensure a smooth transition, maximize efficiency, and achieve the intended business outcomes. Below are the key steps involved in successfully implementing agentic AI:
1. Define Objectives
Before deploying agentic AI, organizations must establish clear goals and expected outcomes. These objectives should align with business priorities, whether it’s improving operational efficiency, enhancing customer service, reducing costs, or driving innovation. Defining key performance indicators (KPIs) helps measure the effectiveness of AI implementation.
2. Identify Suitable Processes
Not all workflows require AI-driven automation, so it's crucial to pinpoint areas where agentic AI can deliver the most value. Processes that involve repetitive decision-making, high-volume data processing, customer interactions, and dynamic problem-solving are ideal candidates. Industries like finance, healthcare, and e-commerce benefit greatly from AI-driven adaptability.
3. Select Appropriate Tools
Organizations must choose AI tools and platforms that align with their technical infrastructure and strategic goals. Platforms like UiPath, Moveworks, and Microsoft AI offer solutions for integrating agentic automation into existing workflows. The selected tools should provide scalability, security, and compatibility with enterprise systems such as CRM and ERP software.
4. Develop and Train AI Agents
AI agents need to be customized for specific business functions. This involves:
-
Designing AI models tailored to handle contextual decision-making and autonomous task execution.
-
Training these models with relevant, high-quality data to enhance their predictive accuracy and adaptability.
-
Implementing reinforcement learning techniques so agents continuously improve based on real-world interactions.
5. Monitor and Optimize
After deployment, continuous monitoring is essential to assess AI agents’ performance. Organizations should:
-
Use real-time analytics to track efficiency, accuracy, and impact on business objectives.
-
Adjust algorithms and retrain models based on feedback and evolving business needs.
-
Ensure AI governance and compliance with regulations to mitigate risks and maintain transparency.
By following these steps, organizations can seamlessly integrate agentic AI, leveraging its autonomy, adaptability, and intelligence to optimize operations, enhance decision-making, and create more agile and efficient workflows.
Benefits of Agentic Automation
The integration of agentic AI into business operations revolutionizes workflow automation by introducing intelligent, adaptive, and autonomous systems. Below are the key benefits of implementing agentic AI in complex workflows:
1. Enhanced Autonomy: Workflows can be managed with minimal human intervention, reducing manual oversight and operational delays. Employees can focus on high-value strategic tasks instead of routine, repetitive processes. This leads to increased productivity and more efficient resource allocation.
2. Real-Time Decision-Making: Intelligent systems analyze live data to make immediate, context-driven decisions without waiting for human input. This ensures faster responses to changing business conditions, reducing delays and inefficiencies. Industries like finance and logistics benefit from instant fraud detection, risk assessment, and operational adjustments.
3. Adaptability: Automated processes can dynamically adjust to new business requirements, shifting priorities, and unforeseen challenges. They handle unstructured data effectively, making them suitable for industries with complex and evolving workflows. This flexibility improves operational resilience and responsiveness in fast-paced environments.
4. End-to-End Workflow Automation: Entire workflows, from data collection to execution, can be streamlined without manual intervention at multiple touchpoints. Automating multi-step processes reduces errors, accelerates task completion, and enhances operational efficiency. Businesses experience fewer bottlenecks and greater consistency in outcomes.
5. Continuous Learning and Improvement: Systems learn from past actions and refine their decision-making processes over time, improving accuracy and efficiency. Advanced models adapt based on new patterns, ensuring better performance with each iteration. This leads to long-term optimization of workflows and better predictive capabilities.
Key Use Cases of Agentic Automation
Customer Support Automation: Virtual assistants and chatbots manage customer inquiries, process service requests, and provide personalized responses. This reduces wait times, ensures faster issue resolution, and enhances overall customer experience. Fraud Detection in Finance: Automated systems continuously monitor transactions, identify unusual patterns, and detect fraudulent activities. By adapting to emerging threats, they help financial institutions prevent unauthorized transactions and minimize risks. Supply Chain Optimization: Intelligent automation predicts demand fluctuations, adjusts stock levels, and optimizes logistics operations. This results in reduced waste, better resource allocation, and improved efficiency across supply chains. Healthcare Diagnostics & Treatment Planning: Advanced automation processes patient data, assists in diagnostics, and provides insights for treatment planning. This enhances medical accuracy, improves decision-making, and ensures better patient care. HR & Recruitment Automation: Automated tools handle resume screening, schedule interviews, and streamline the hiring process. This reduces recruitment time, enhances candidate selection, and improves the overall onboarding experience.
Comparison of Agentic Automation, AI-Powered Automation, and RPA
Feature |
Agentic Automation |
AI-Powered Automation |
RPA (Robotic Process Automation) |
Definition |
Autonomous systems that learn, adapt, and make decisions. |
Uses AI to enhance automation with learning and reasoning. |
Rule-based automation for repetitive tasks. |
Decision-Making |
Analyzes, decides, and adjusts workflows dynamically. |
Uses AI models but often needs human oversight. |
Follows pre-set rules with no contextual decisions. |
Adaptability |
Continuously evolves to handle new scenarios. |
Limited adaptability based on training data. |
Rigid, requires manual updates for changes. |
Scope |
End-to-end automation, including unstructured tasks. |
Enhances workflows with AI-driven insights. |
Automates repetitive, structured tasks. |
Human Intervention |
Minimal, operates autonomously. |
Reduces human involvement but still needs monitoring. |
High, requires oversight and updates. |
Scalability |
Highly scalable, adapts to business needs. |
Scalable but depends on AI model quality. |
Limited, needs manual reconfiguration. |
The Road Ahead: Future Trends in Agentic Automation
As intelligent systems evolve, they will reshape industries by enabling smarter workflows, deeper collaboration, and greater adaptability. The next phase of automation will focus on enhancing decision-making, expanding applications, and ensuring ethical implementation.
-
Smarter Decision-Making: Systems will evolve from simple task execution to making complex decisions with minimal intervention, improving efficiency across industries.
-
Advanced Adaptability: Intelligent tools will enhance problem-solving, content creation, and customer interactions, making processes more dynamic and responsive.
-
Wider Industry Adoption: Beyond traditional sectors, applications will expand into legal, education, and creative fields, streamlining analysis, learning, and content generation.
-
Stronger Human-Machine Collaboration: Technology will complement human efforts by automating repetitive tasks, providing insights, and boosting overall productivity.
-
Focus on Ethics & Compliance: Transparent governance and regulatory frameworks will ensure fairness, accountability, and responsible decision-making.
These advancements will redefine efficiency, innovation, and competitiveness in the evolving digital landscape.
Next Steps with AI Agents
Talk to our experts about implementing compound AI system, How Industries and different departments use Agentic Workflows and Decision Intelligence to Become Decision Centric. Utilizes AI to automate and optimize IT support and operations, improving efficiency and responsiveness.