Key Insights
The integration of AI agents in autonomous material handling is transforming manufacturing by enhancing efficiency, accuracy, and scalability. These intelligent systems optimize workflows, reduce downtime, and adapt dynamically to changing production demands. By automating material transport, storage, and sorting, AI agents ensure seamless operations, improved safety, and significant cost savings. As manufacturing evolves, AI agents are driving smarter and more sustainable production processes.
The manufacturing industry is transforming rapidly, with efficiency and precision becoming essential for success. Autonomous Material Handling (AMH) systems, powered by Agentic AI, are leading this revolution by automating key processes such as material transport, storage, and distribution. These advanced systems offer unparalleled accuracy and reliability, enabling manufacturers to meet increasing demands while minimizing errors and streamlining workflows.
By integrating AI agents, AMH systems can adapt to dynamic production environments, optimize material flow, and ensure seamless operations across warehouses and assembly lines. The result is a significant boost in productivity, cost savings, and overall operational efficiency. In this blog, we explore the transformative role of Agentic AI in AMH systems, their applications in manufacturing, and how they are shaping the future of intelligent, automated production environment.
What is Autonomous Material Handling?
Autonomous Material Handling in manufacturing involves the automated movement, storage, and control of materials and goods within production facilities using technologies like robotics, AI agents, and smart sensors. These systems, including Automated Guided Vehicles (AGVs), Automated Mobile Robots (AMRs), and intelligent conveyor systems, operate independently, leveraging real-time data to optimize routing, streamline workflows, and enhance efficiency.
By integrating advanced AI capabilities, these systems can dynamically adapt to changing production demands, ensure accurate material placement, and reduce the need for manual intervention. This approach not only boosts productivity but also improves safety, reduces costs, and enables manufacturers to meet the growing demand for faster, more flexible operations.
A Brief Overview of Autonomous Material Handling in Manufacturing
It represents a significant leap forward in manufacturing, merging automation with intelligent decision-making to revolutionize how materials are moved, stored, and processed. Unlike traditional material handling methods that rely heavily on manual labor and fixed systems, AMH leverages AI agents, robotics, and machine learning to create adaptive, efficient workflows.
These systems aren’t limited to just moving goods; they analyze operations in real-time, identifying bottlenecks, optimizing routes, and improving throughput. Examples include Autonomous Mobile Robots (AMRs) that transport items within warehouses and intelligent sorting systems that handle materials with precision and speed.
The shift to AMH is a response to the manufacturing sector’s need for greater agility and scalability. By integrating advanced technologies, manufacturers can achieve higher productivity, lower costs, and improved safety—all while adapting to ever-changing production demands.
Traditional vs. Agentic AI Material Handling Systems
Aspect |
Traditional Material Handling Systems |
Agentic AI Material Handling Systems |
Flexibility |
Limited flexibility requires significant reconfiguration for changes in layout or product. |
High flexibility can adapt to changes in layout, product types, and operational needs in real-time. |
Adaptability to Environment |
Fixed or semi-fixed systems cannot respond to unexpected changes or obstacles. |
Able to detect and respond to obstacles and environmental changes autonomously using sensors and AI. |
Efficiency |
Efficiency depends on pre-set schedules, fixed paths, and limited real-time adjustments. |
High efficiency through continuous optimization, real-time route planning, and resource allocation. |
Maintenance |
Requires regularly scheduled maintenance based on predefined criteria. |
Predictive maintenance powered by AI, identifying potential failures before they occur, minimizing downtime. |
Cost |
Higher upfront costs for installation of rigid systems and frequent system upgrades. |
Potentially higher initial costs due to AI technologies but can reduce long-term operational costs through efficiency improvements and reduced labor. |
Safety |
Safety depends on manual monitoring and basic safety features in machines. |
Enhanced safety through AI sensors, collision avoidance, and real-time monitoring, reducing human-related accidents. |
Akira AI: Multi-Agent System in Autonomous Material Handling
Fig1 :Architecture Diagram of Autonomous Material Handling
-
Material Identification: The Sensor Agent employs IoT sensors and cameras to detect and classify materials, capturing crucial details such as type, size, and condition. This data triggers appropriate actions, ensuring precise handling and efficient material flow within the system.
-
Routing and Planning: The Routing Agent processes the material data and strategically plans the most efficient movement paths. It dynamically adjusts for obstacles, resource availability, and changes in the environment, ensuring smooth, uninterrupted transportation of materials within the facility.
-
Movement Execution: The Movement Agent autonomously navigates materials along the optimized route. It avoids obstacles and adapts to environmental changes using real-time data, ensuring materials are transported safely and efficiently without human intervention or delays.
-
Storage and Sorting: The Sorting Agent categorizes and stores materials based on predefined criteria such as size, type, or usage. It optimizes available storage space and ensures accurate inventory management, making materials easy to access and ready for the next process.
-
Monitoring and Feedback: The Monitoring Agent continuously tracks system performance, identifying inefficiencies, disruptions, or potential failures. It provides real-time feedback, ensuring smooth operation, enabling quick troubleshooting, and supporting ongoing improvements to maintain operational efficiency.
-
Maintenance and Optimization: The Maintenance Agent uses predictive algorithms to assess the system's health. It schedules necessary maintenance and repairs before failures occur, optimizing the system's performance, reducing downtime, and ensuring the longevity and reliability of the entire material handling operations.
Use cases of Autonomous Material handling
-
Automated Warehousing and Inventory Management: Robots and guided vehicles transport goods within warehouses, optimizing storage and inventory control. These systems automatically update stock levels, retrieve products, and deliver them to the right locations with minimal human input.
-
Automated Sorting and Packaging: Sorting systems can automatically identify, classify, and organize materials based on predefined criteria such as size, weight, or product type. This is especially useful in high-volume environments, reducing human error and improving sorting efficiency.
-
Smart Storage and Retrieval Systems: Intelligent systems manage material storage in complex racking systems, retrieving items as needed. They optimize space usage and ensure that products are stored and retrieved efficiently, improving overall workflow.
-
Automated Assembly Line Support: Robots assist with loading and unloading materials on assembly lines, ensuring smooth transitions between manufacturing stages. They handle components with precision, preventing delays and improving operational efficiency.
-
Predictive Maintenance for Material Handling Equipment: Sensors monitor the health of material handling equipment like conveyor belts and robots, predicting potential failures. Maintenance is scheduled before breakdowns occur, reducing downtime and keeping operations running smoothly.
-
Automated Quality Control: Equipped with sensors and cameras, robots conduct real-time inspections of materials and products, identifying defects or inconsistencies in quality. This ensures high-quality production with minimal manual oversight.
Operational Benefits of Autonomous Material Handling in Manufacturing
-
Increased Efficiency: Autonomous systems powered by AI can operate 24/7 without breaks, significantly increasing throughput and reducing downtime. For example, warehouse AI agents will drive 80% of the workload by 2025.
-
Productivity Boost: AI-driven systems can improve overall productivity by 30% by automating routine tasks and allowing human workers to focus on higher-level activities.
-
Improved Accuracy: AI-enabled AMHS can minimize human errors, such as misplacement of goods, ensuring that products are correctly stored, sorted, and shipped.
-
Faster Processing: With real-time decision-making capabilities, AI systems can optimize routing and reduce transit times for materials, speeding up the overall manufacturing process.
-
Safety and Risk Reduction: Autonomous robots reduce the risk of workplace accidents. Sensors and machine learning algorithms allow robots to navigate around obstacles and adapt to their environment, keeping human workers safe.
Technologies Transforming Autonomous Material Handling
Several key technologies are enabling the rise of AI-driven autonomous material handling systems:
-
Robotics and Machine Learning: Robots use machine learning to improve their operations over time, learning how to handle different materials and environments efficiently.
-
Internet of Things (IoT): IoT sensors track the movement and condition of materials in real-time, providing critical data for AI systems to optimize routing and handling.
-
Computer Vision: AI-powered cameras and sensors help robots understand their surroundings, identifying obstacles, inventory, and packaging.
-
Cloud Computing: Cloud-based platforms allow AMHS to share data across different machines and locations, making it easier to manage large-scale automated operations
The Future Trends of Autonomous Material Handling
The future of autonomous material handling in manufacturing looks incredibly promising. Here’s what we can expect:
-
Collaborative Human-Robot Work Environments: The next generation of material handling will feature greater collaboration between human workers and robots. While robots handle repetitive and physically demanding tasks, humans will focus on higher-level decision-making, improving safety and productivity through teamwork.
-
Real-Time Data and IoT Integration: Increased use of IoT sensors will enhance material tracking and provide real-time operational insights. These systems will monitor inventory, material condition, and overall process performance, allowing for better resource allocation and faster decision-making.
-
Improved Mobility of Autonomous Robots: Future autonomous vehicles will become more flexible in navigating complex environments. With enhanced navigation and obstacle avoidance, these systems will be able to operate seamlessly in unstructured settings, including outdoor or crowded warehouse environments.
-
Comprehensive Automation Across Manufacturing Plants: Full integration of autonomous systems will allow for complete automation of material handling from start to finish. These systems will manage everything from material intake to final delivery, optimizing workflows and increasing efficiency throughout the production cycle.
-
Customizable and Flexible Automation Solutions: Material handling solutions will become more customizable, enabling manufacturers to tailor systems to their unique needs. This flexibility will allow for rapid adjustments to production schedules, product types, and fluctuating demand.
Conclusion: AI Agents for Autonomous Material Handling
The integration of AI in autonomous material handling systems is a game changer for modern manufacturing. As AI teammates continue to evolve, they are set to transform not only the logistics side of manufacturing but also the entire production cycle. By automating material handling, companies can enhance efficiency, reduce costs, and improve safety, all while scaling operations to meet growing demand. Whether it's AI-driven robots in warehouses, semiconductor fabs, or automotive plants, the future of manufacturing will undoubtedly be shaped by autonomous material handling systems. As manufacturing industries continue to explore these technologies, the synergy between automated material handling systems and AI teammates will further push the boundaries of what’s possible, driving smarter, more sustainable production processes for the future.
Explore More Optimizing Production Lines with Agentic AI
Discover More About Manufacturing Production with AI Agents
Upgrade Your Manufacturing with Agentic AI-Powered Automation
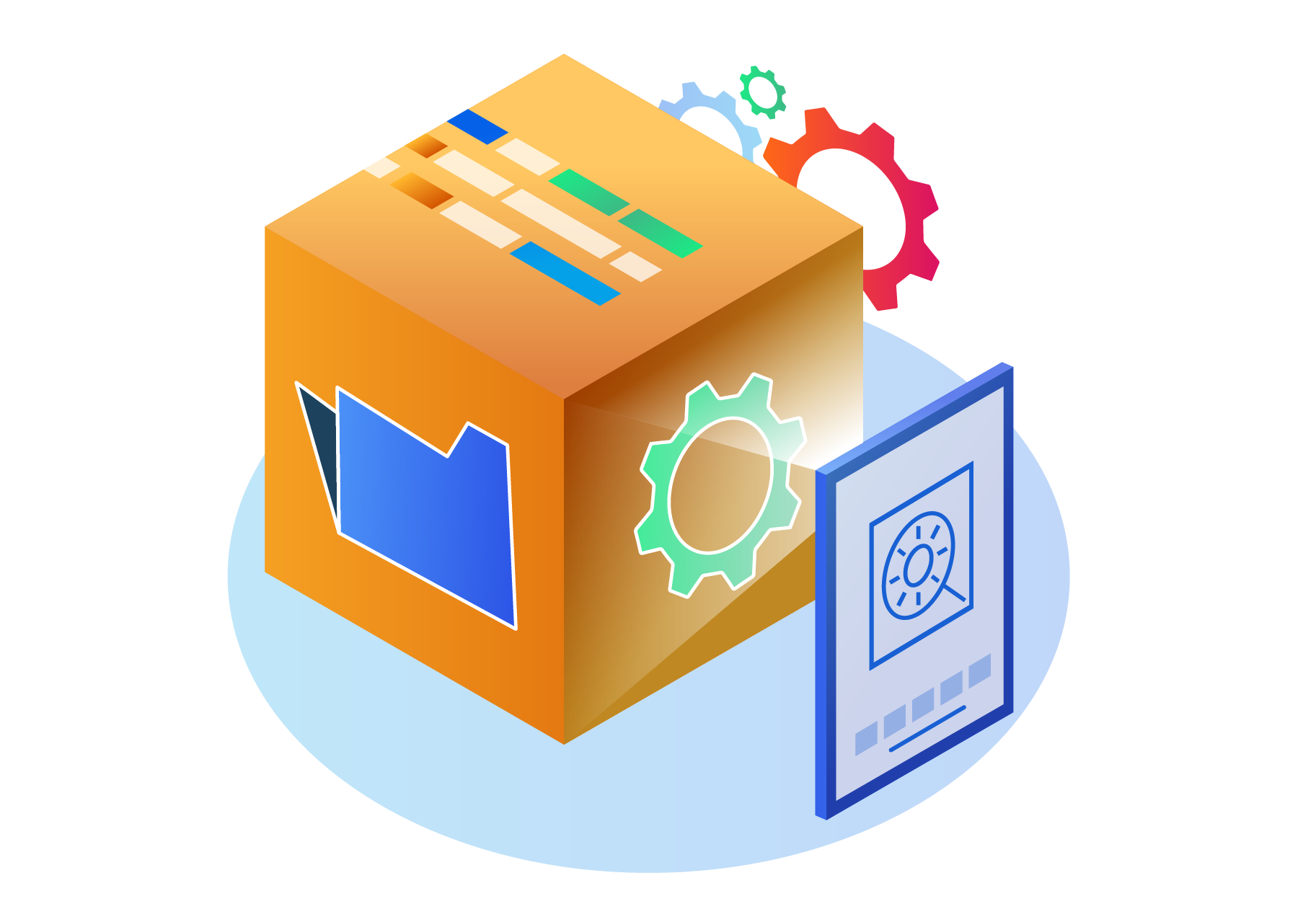